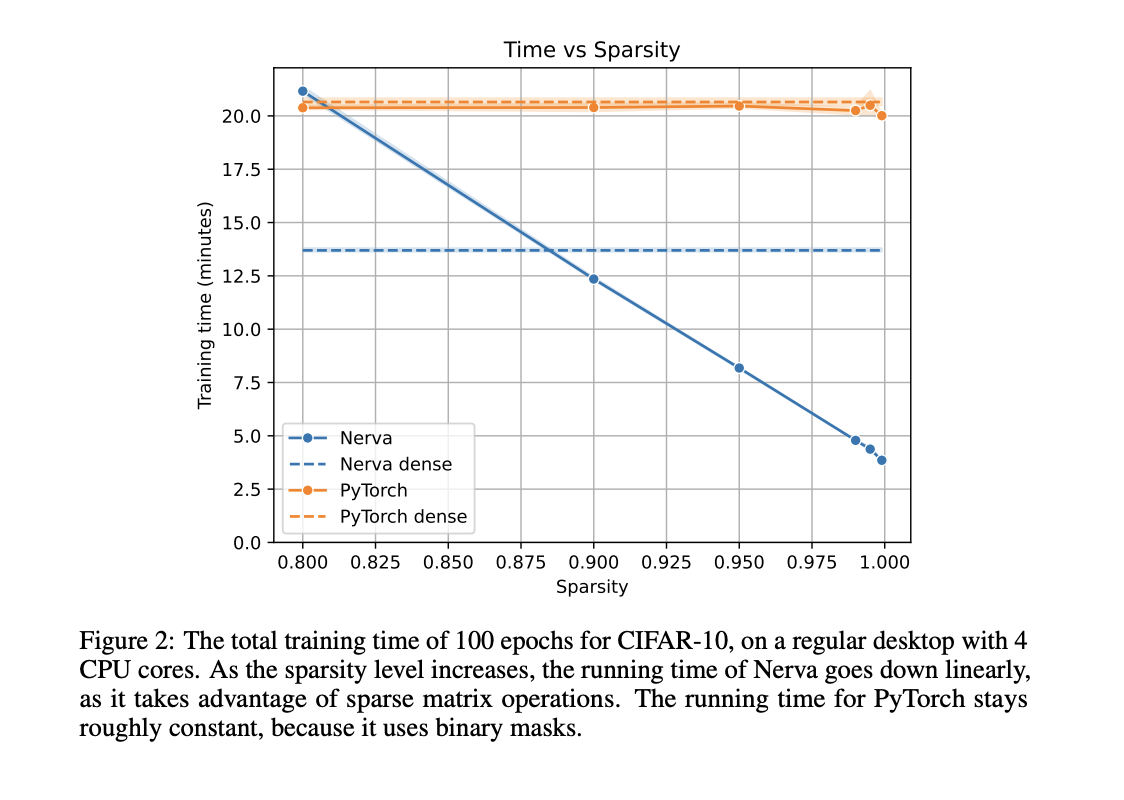
Practical Solutions for Efficient Sparse Neural Networks
Addressing the Challenge
Deep learning has shown potential in various applications, but the extensive computational power needed for training and testing neural networks poses a challenge. Researchers are exploring sparsity in neural networks to create powerful and resource-efficient models.
Optimizing Memory and Computation
Traditional compression techniques often retain zeroed weights in memory, limiting the potential benefits of sparsity. This calls for genuinely sparse implementations that fully optimize memory and computational resources.
Introducing Nerva
Eindhoven University of Technology researchers have introduced Nerva, a novel neural network library in C++ designed to provide a truly sparse implementation. Nerva leverages sparse matrix operations to significantly reduce the computational burden associated with neural networks, leading to substantial memory savings.
Performance Evaluation
Nerva demonstrated a linear decrease in runtime with increasing sparsity levels, outperforming PyTorch in high sparsity regimes. It achieved accuracy comparable to PyTorch while significantly reducing training and inference times, highlighting its efficiency in sparse neural network training.
Value of Nerva
Nerva’s design focuses on runtime efficiency, memory efficiency, energy efficiency, and accessibility, ensuring it can effectively meet the research community’s needs. With ongoing development and plans to support dynamic sparse training and GPU operations, Nerva is poised to become a valuable tool for optimizing neural network models.
AI Solutions for Your Business
If you want to evolve your company with AI, stay competitive, and enhance efficiency and performance, consider leveraging Nerva. Connect with us at hello@itinai.com for AI KPI management advice and continuous insights into leveraging AI.