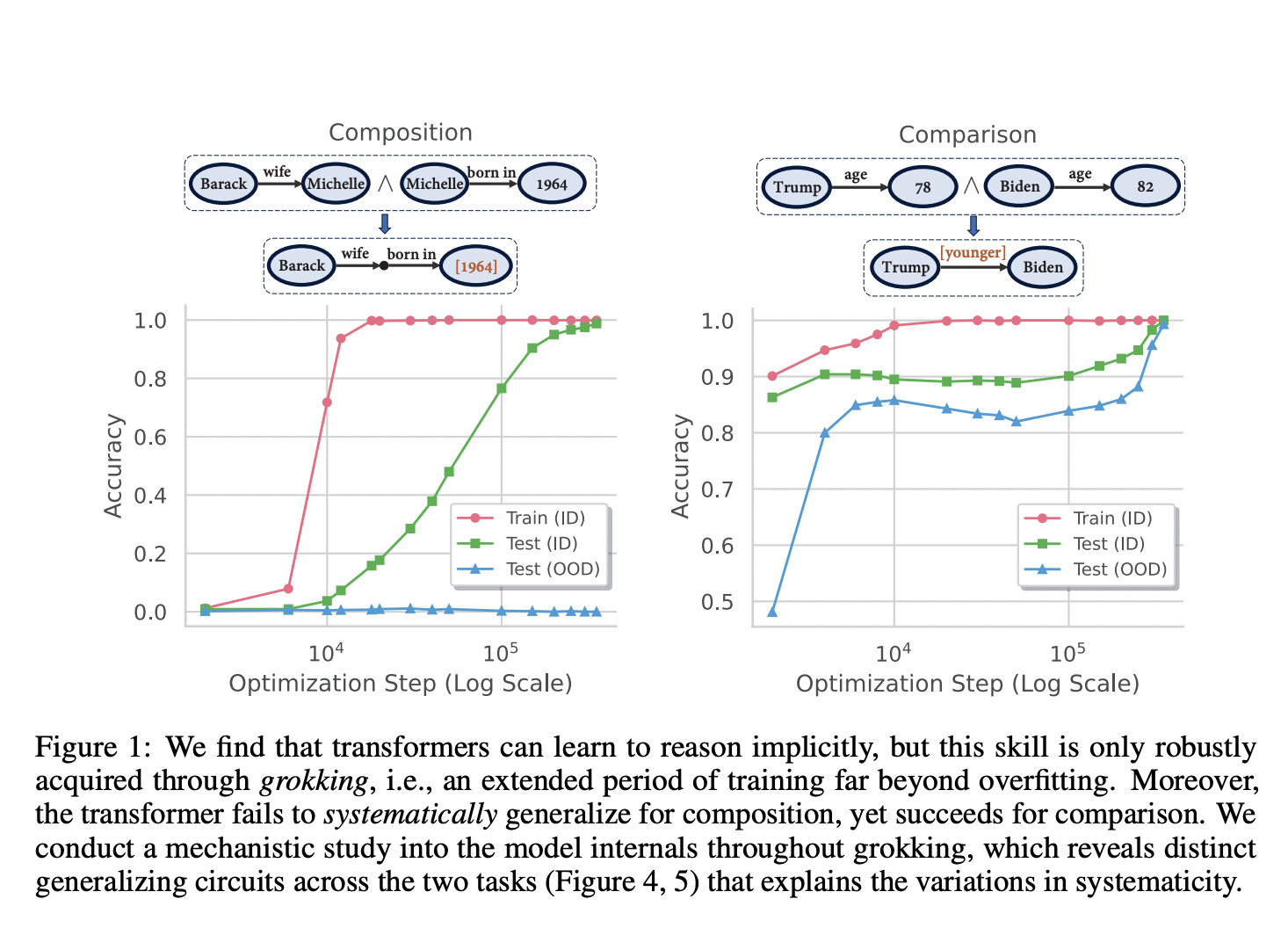
Implicit Reasoning in Transformers: Practical Solutions and Value
Challenges in Implicit Reasoning
Large Language Models (LLMs) face limitations in implicit reasoning, leading to difficulties in integrating internalized facts and inducing structured representations of rules and facts. This results in redundant knowledge storage and impairs the model’s capacity to systematically generalize knowledge.
Research on Deep Learning Models
Researchers from Ohio State University and Carnegie Mellon University have studied whether transformers can learn to reason implicitly over parametric information, focusing on comparison and composition reasoning. They found that transformers can learn implicit reasoning through a process called grokking, which enables robust reasoning capabilities.
Impact on Transformers’ Reasoning Abilities
Transformers struggle to generalize effectively for composition tasks with out-of-distribution examples but perform well for comparison tasks. The research has identified the mechanism of grokking and its impact on the model’s ability to perform implicit reasoning.
Improving Transformer Architecture
The study suggests that the transformer architecture can be enhanced by promoting cross-layer knowledge sharing, which could strengthen the reasoning capabilities of the model. Additionally, the research demonstrates the promise of parametric memory in enabling sophisticated reasoning in language models.
Practical Applications of AI
AI can redefine work processes by identifying automation opportunities, defining measurable KPIs, selecting appropriate AI solutions, and implementing AI gradually. For AI KPI management advice and insights into leveraging AI, connect with us at hello@itinai.com or follow us on Telegram and Twitter for continuous updates.