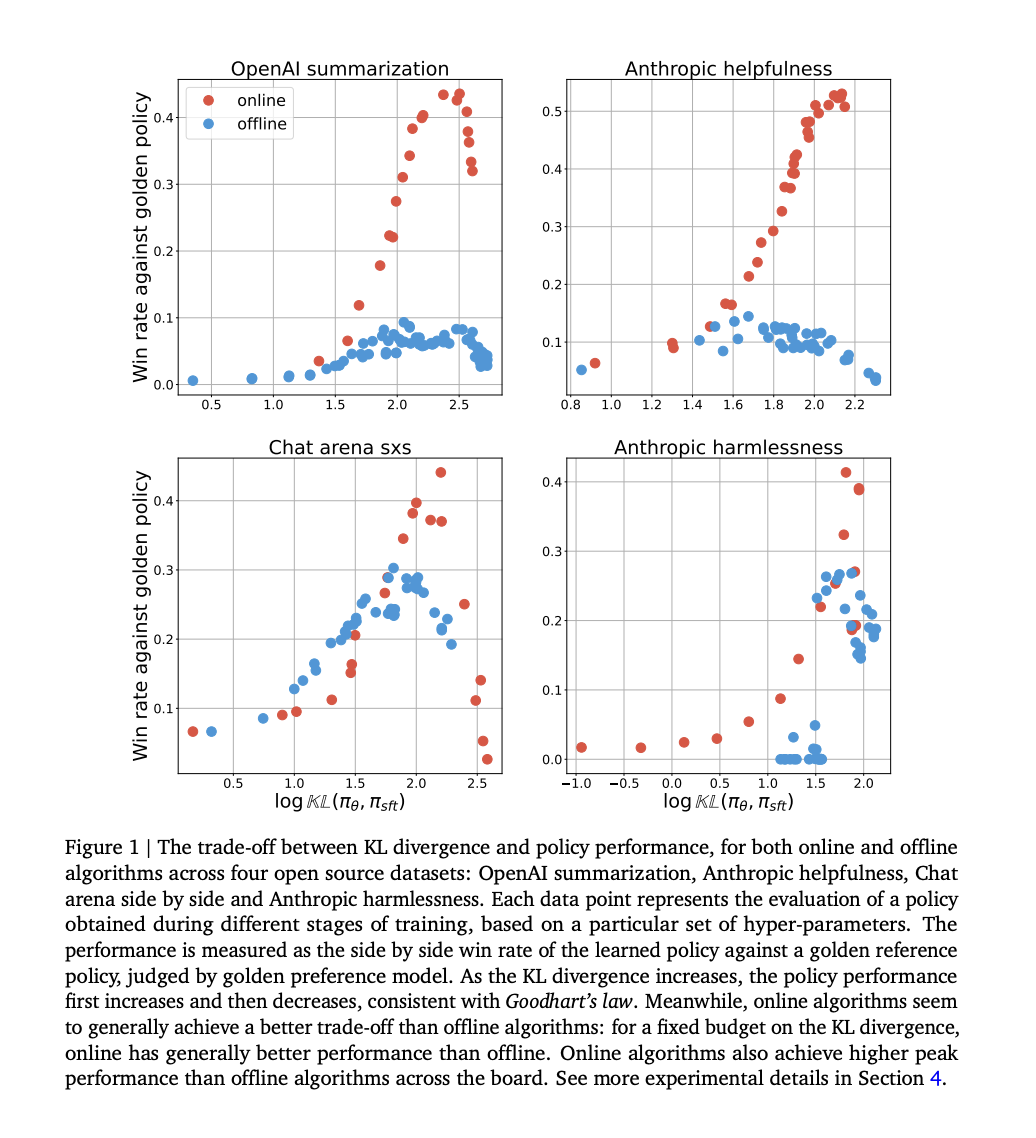
AI Solutions for Effective Alignment of Language Models
Research Highlights
Recent advances in AI alignment show that offline alignment methods, such as direct preference optimization (DPO), challenge the necessity of on-policy sampling in Reinforcement Learning from Human Feedback (RLHF) approaches. Offline methods align language models efficiently using pre-existing datasets without active online interaction, making them simpler and cheaper to implement.
Controlled experiments by Google DeepMind researchers demonstrate that online methods outperform offline methods in initial tests, indicating the crucial role of on-policy sampling in AI alignment. Comparing online and offline methods underlines the challenges in offline alignment, emphasizing the need for careful budget calibration to measure performance fairly.
Practical Value
The study provides practical insights into the performance gap between online and offline AI alignment methods, urging businesses to consider on-policy sampling as crucial for effectively aligning language models.
For businesses looking to leverage AI, identifying automation opportunities, defining measurable KPIs, selecting customized AI solutions, and implementing AI gradually are recommended. In this context, AI Sales Bot from itinai.com/aisalesbot is highlighted as a practical solution to automate customer engagement and improve sales processes.
For AI KPI management advice and continuous insights into leveraging AI, connect with itinai.com through their Telegram channel or Twitter.
Further Exploration
This research opens avenues for further exploration, such as hybrid approaches combining the strengths of both online and offline AI alignment methods, as well as deeper theoretical investigations into reinforcement learning for human feedback.
For deeper insights into how AI can redefine your work processes, explore AI solutions at itinai.com and stay competitive in the evolving AI landscape.