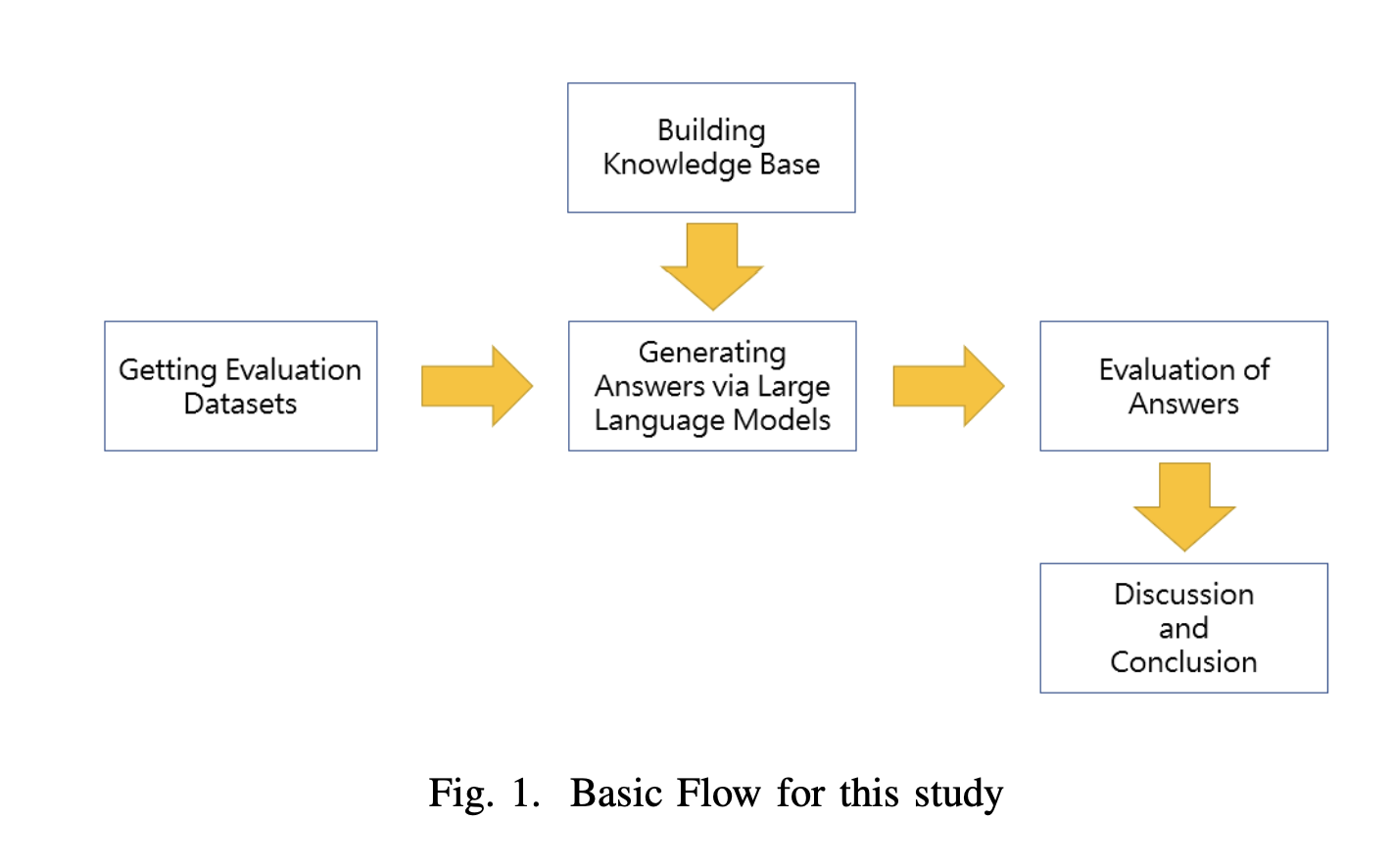
Enhancing Knowledge Retrieval Systems with AI
Knowledge retrieval systems have been used for many years in various fields like healthcare, education, and finance. Today, they are improved by large language models (LLMs) that provide more accurate answers. However, there is a need for better handling of unclear queries and up-to-date information. Researchers from National Taiwan University and National Chengchi University have developed a new method that combines retrieval-augmented generation (RAG) with adaptive mechanisms to improve LLM accuracy and reliability.
Challenges with Traditional Systems
Traditional retrieval systems often depend on keyword matching, which can lead to irrelevant answers, especially with vague inputs. They also struggle to adapt to new information, resulting in incorrect outputs. RAG is an advanced method that integrates retrieval and generation but can still be unreliable due to its reliance on outdated knowledge bases. A new approach is needed to enhance both processes.
Proposed Methodology
The new method introduces a multi-step, dynamic strategy to improve RAG and information retrieval:
- Contextual Embedding Techniques: Queries are transformed into vector representations to better capture their meaning and handle ambiguity.
- Adaptive Attention Mechanisms: This method uses a dynamic attention mechanism to focus on the specific context of user queries, integrating real-time information effectively.
- Dual-Model Framework: It includes a retrieval model that extracts information and a generative model that compiles this information into coherent responses.
- Fine-Tuned Training: The model can be customized for specific industries to enhance contextual understanding.
Results and Benefits
This method was tested on Chinese Wikipedia and Lawbank, showing significant improvements in retrieval accuracy and a reduction in errors. It maintained competitive speed for real-time applications across various fields. Users reported higher satisfaction due to more accurate and relevant responses.
Significance of the Research
The RAG-based system represents a major improvement over traditional systems, offering better accuracy and reliability through dynamic adaptation and enhanced knowledge integration. Its scalability and adaptability make it a significant step forward for future AI systems, especially in information-heavy industries.
Take Action with AI
If you want to enhance your business with AI, consider the following steps:
- Identify Automation Opportunities: Find key customer interactions that can benefit from AI.
- Define KPIs: Set measurable goals for your AI initiatives.
- Select an AI Solution: Choose tools that fit your needs and allow for customization.
- Implement Gradually: Start with a pilot project, gather data, and expand wisely.
For AI management advice, contact us at hello@itinai.com. Stay updated on AI insights by following us on Telegram or @itinaicom.