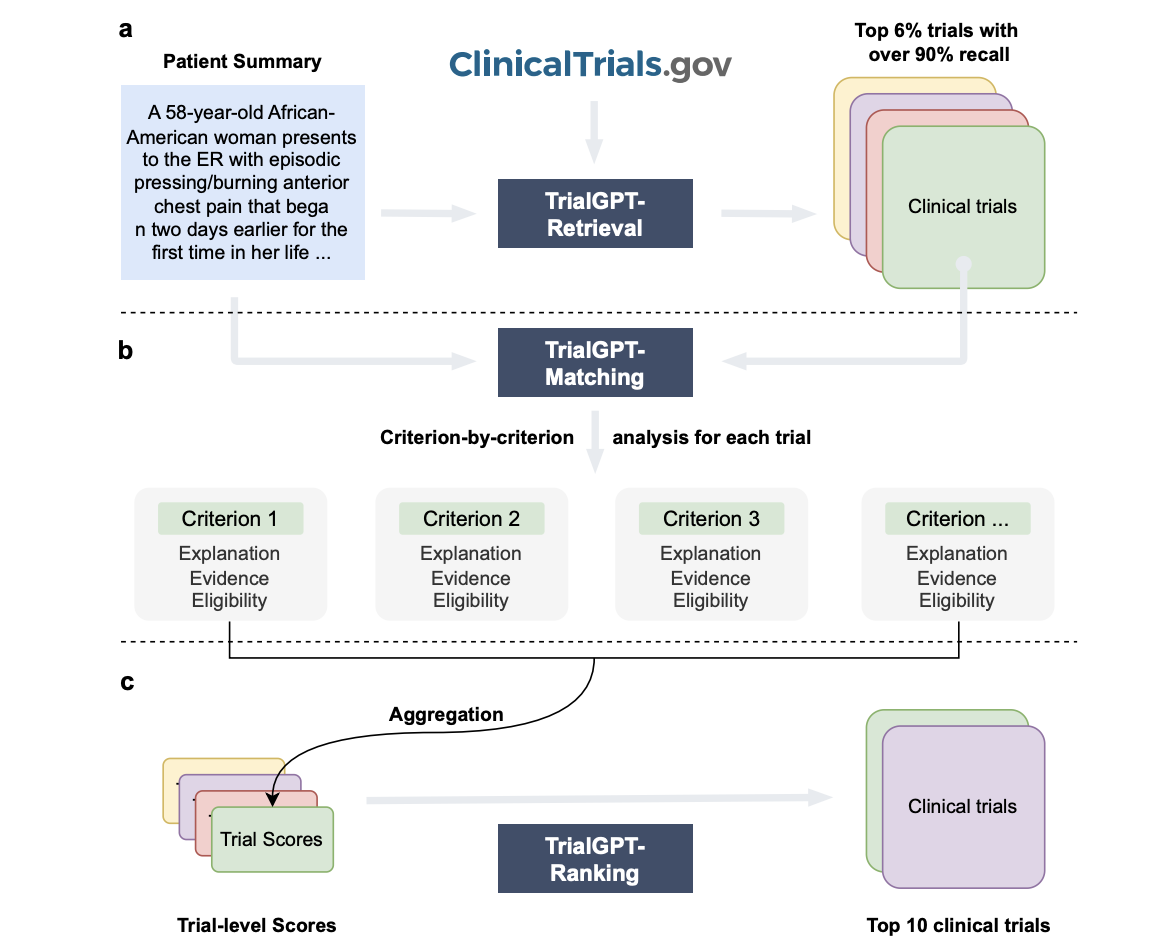
Revolutionizing Patient-to-Trial Matching with TrialGPT
Challenges in Clinical Trial Matching
Matching patients with appropriate clinical trials is crucial yet difficult. It requires detailed analysis of patients’ medical histories against complex trial eligibility criteria. This process is time-consuming, often leading to delays in accessing vital experimental treatments, particularly in fields like oncology and rare diseases.
Limitations of Current Methods
Existing patient-trial matching methods often face issues such as:
– Dependence on large, annotated datasets that are hard to gather.
– Low efficiency and poor real-time application capabilities.
– Lack of transparent predictions, making it hard for clinicians to trust the results.
Introducing TrialGPT
To tackle these challenges, researchers developed **TrialGPT**, an innovative framework that uses large language models (LLMs) to improve patient-to-trial matching. TrialGPT consists of three main components:
– **TrialGPT-Retrieval**: Filters out irrelevant trials using hybrid retrieval methods and keywords derived from patient summaries.
– **TrialGPT-Matching**: Evaluates patient eligibility at a detailed level, providing clear explanations and evidence.
– **TrialGPT-Ranking**: Prioritizes trials based on the collected data, helping to eliminate unsuitable options.
Proven Effectiveness
TrialGPT was tested on public datasets, successfully analyzing over 75,000 trial annotations and 183 synthetic patients. Key findings include:
– **High Recall**: The retrieval component achieved over 90% recall, reducing the search space by 94%.
– **Accurate Matching**: The matching component matched human expert accuracy, providing understandable eligibility predictions.
– **Improved Efficiency**: This system decreased screening time by over 42%, enhancing the recruitment process.
Practical Benefits
TrialGPT offers significant advantages:
– **Scalability**: It efficiently handles large volumes of trials.
– **Transparency**: Clear explanations foster clinician confidence.
– **Better Outcomes**: Streamlined processes lead to quicker access to experimental treatments for patients.
Future Directions
Looking ahead, there are plans to incorporate multi-modal data sources and adapt open-source LLMs for real-world applications.
Get Involved
Check out the research paper behind TrialGPT and stay updated by following us on Twitter, joining our Telegram channel, and subscribing to our LinkedIn group. If you’re interested in AI’s transformative potential, don’t forget to join our newsletter and our 55k+ ML SubReddit.
Upcoming Event
Join us on **December 11th** for **SmallCon**, a free virtual conference featuring industry leaders like Meta and Salesforce, discussing how to leverage AI effectively.
Contact Us
To evolve your company with AI and identify automation opportunities, reach out to us at hello@itinai.com. Follow us for ongoing insights on Twitter @itinaicom and on Telegram t.me/itinainews. Explore how AI can enhance your sales processes and customer engagement at itinai.com.