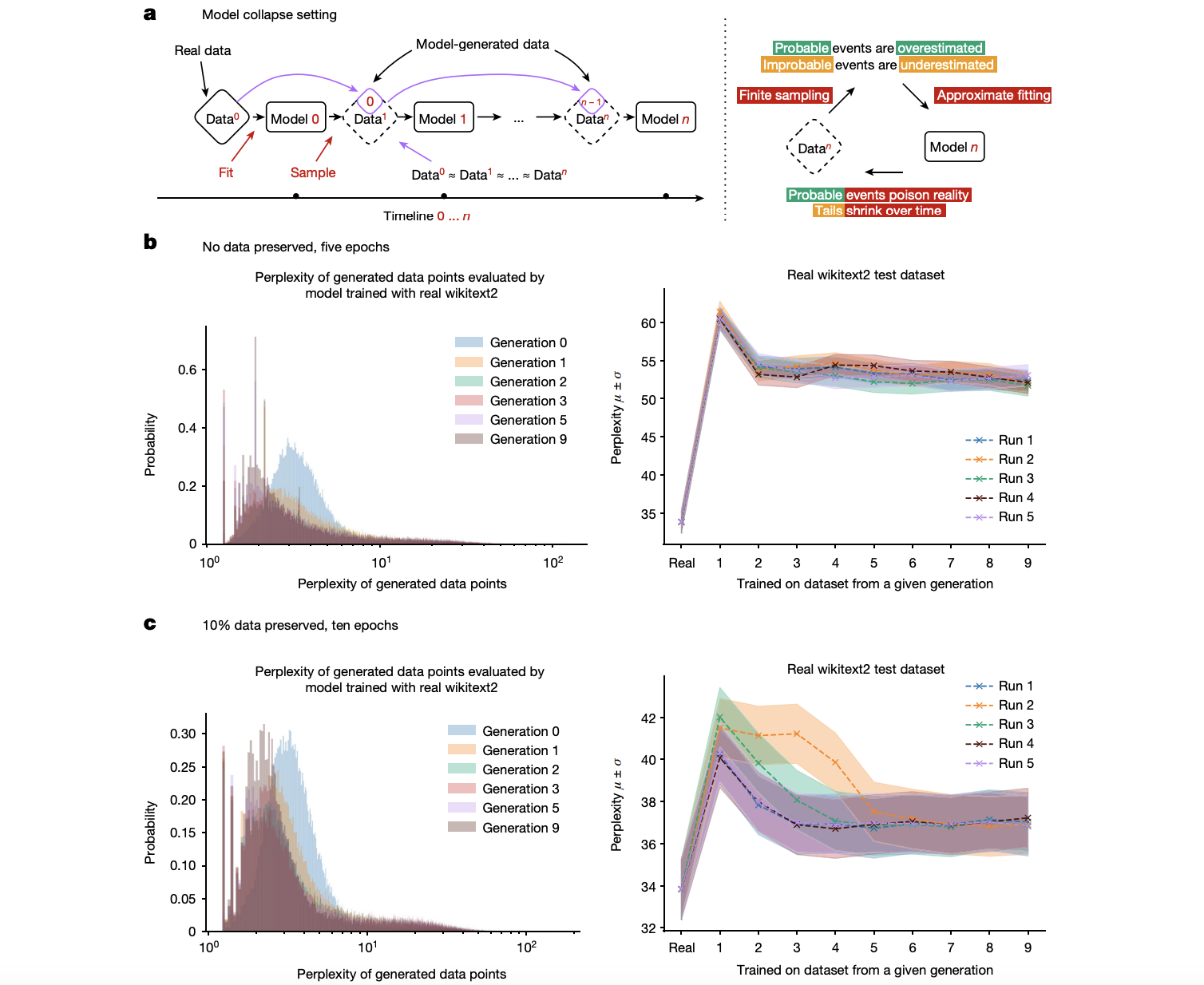
The Challenge of Model Collapse in AI Research
The phenomenon of “model collapse” presents a significant challenge in AI research, particularly for large language models (LLMs). When these models are trained on data that includes content generated by earlier versions of similar models, they tend to lose their ability to represent the true underlying data distribution over successive generations. This issue compromises the performance and reliability of AI systems, which are increasingly integrated into various applications, from natural language processing to image generation.
Practical Solutions and Value
Addressing this challenge is essential to ensure that AI models can maintain their effectiveness and accuracy without degradation over time. Current methods to tackle training AI models involve using large datasets predominantly generated by humans. Techniques such as data augmentation, regularization, and transfer learning have been employed to enhance model robustness. However, these methods have limitations, such as the requirement for vast amounts of labeled data, which is not always feasible to obtain. The researchers present a novel approach involving a detailed examination of the “model collapse” phenomenon. They provide a theoretical framework and empirical evidence to demonstrate how models trained on recursively generated data gradually lose their ability to represent the true underlying data distribution. This approach specifically addresses the limitations of existing methods by highlighting the inevitability of model collapse in generative models, regardless of their architecture. The core innovation lies in identifying the sources of errors—statistical approximation error, functional expressivity error, and functional approximation error—that compound over generations, leading to model collapse. The technical approach employed in this research leverages datasets like wikitext2 to train language models, systematically illustrating the effects of model collapse through a series of controlled experiments.
AI Solutions for Business Transformation
Discover how AI can redefine your way of work. Identify Automation Opportunities: Locate key customer interaction points that can benefit from AI. Define KPIs: Ensure your AI endeavors have measurable impacts on business outcomes. Select an AI Solution: Choose tools that align with your needs and provide customization. Implement Gradually: Start with a pilot, gather data, and expand AI usage judiciously. For AI KPI management advice, connect with us at hello@itinai.com. And for continuous insights into leveraging AI, stay tuned on our Telegram t.me/itinainews or Twitter @itinaicom. Discover how AI can redefine your sales processes and customer engagement. Explore solutions at itinai.com.