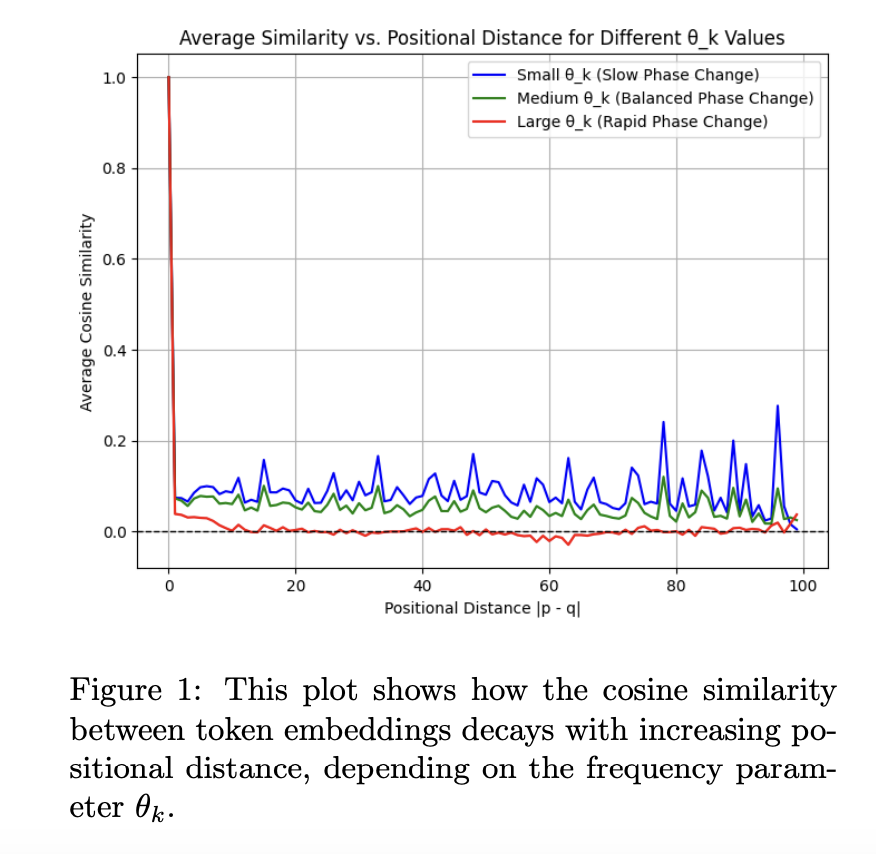
Understanding Rotary Positional Embeddings (RoPE)
Rotary Positional Embeddings (RoPE) is a cutting-edge method in artificial intelligence that improves how transformer models understand the order of data, particularly in language processing. Traditional transformer models often struggle with the sequence of tokens because they analyze each one separately. RoPE helps these models recognize the position of tokens in a sequence, enhancing their ability to manage ordered data effectively.
Challenges with Traditional Methods
Standard methods like sinusoidal and relative encodings provide some positional awareness but often fail in complex tasks. While models like Transformer-XL increase memory capacity for long dependencies, they do not effectively manage the frequency of embeddings, limiting their performance in tasks requiring deep contextual understanding.
Research Insights from Sapienza University
Researchers at Sapienza University of Rome studied how RoPE-modulated embeddings work with transformer models, particularly focusing on feed-forward networks (FFNs). They discovered that the interaction between activation functions and RoPE embeddings creates frequency-based harmonics, which enhance the model’s focus and memory retention.
Key Findings on Phase Alignment
The study combined theoretical and practical analyses to assess RoPE’s impact on autoregressive models like LLaMA 2 and LLaMA 3. By simulating phase shifts in embeddings, researchers found that aligned phases lead to stable activation patterns, while misaligned phases resulted in instability, making it harder for the model to retain information over long sequences.
Benefits of RoPE in Transformers
RoPE introduces oscillations in embeddings based on their position, enriching the model’s attention mechanism. When embeddings are phase-aligned, they amplify relevant activations, improving the model’s ability to focus on important patterns. Conversely, misalignment weakens attention, complicating the retention of long-term dependencies.
Conclusion
This research highlights that RoPE significantly enhances attention focus and memory retention in transformers. By understanding phase alignment and interference, we can improve how transformers handle sequential data, especially in tasks requiring both short- and long-term dependencies.
For more details, check out the Paper. All credit goes to the researchers involved in this project. Don’t forget to follow us on Twitter, join our Telegram Channel, and connect with our LinkedIn Group. If you appreciate our work, you will love our newsletter. Join our 55k+ ML SubReddit.
Transform Your Business with AI
Stay competitive and leverage AI to your advantage. Here’s how:
- Identify Automation Opportunities: Find key customer interactions that can benefit from AI.
- Define KPIs: Ensure your AI initiatives have measurable impacts on business outcomes.
- Select an AI Solution: Choose tools that fit your needs and allow for customization.
- Implement Gradually: Start with a pilot project, gather data, and expand AI usage wisely.
For AI KPI management advice, connect with us at hello@itinai.com. For ongoing insights into leveraging AI, stay tuned on our Telegram t.me/itinainews or Twitter @itinaicom.
Discover how AI can transform your sales processes and enhance customer engagement. Explore solutions at itinai.com.