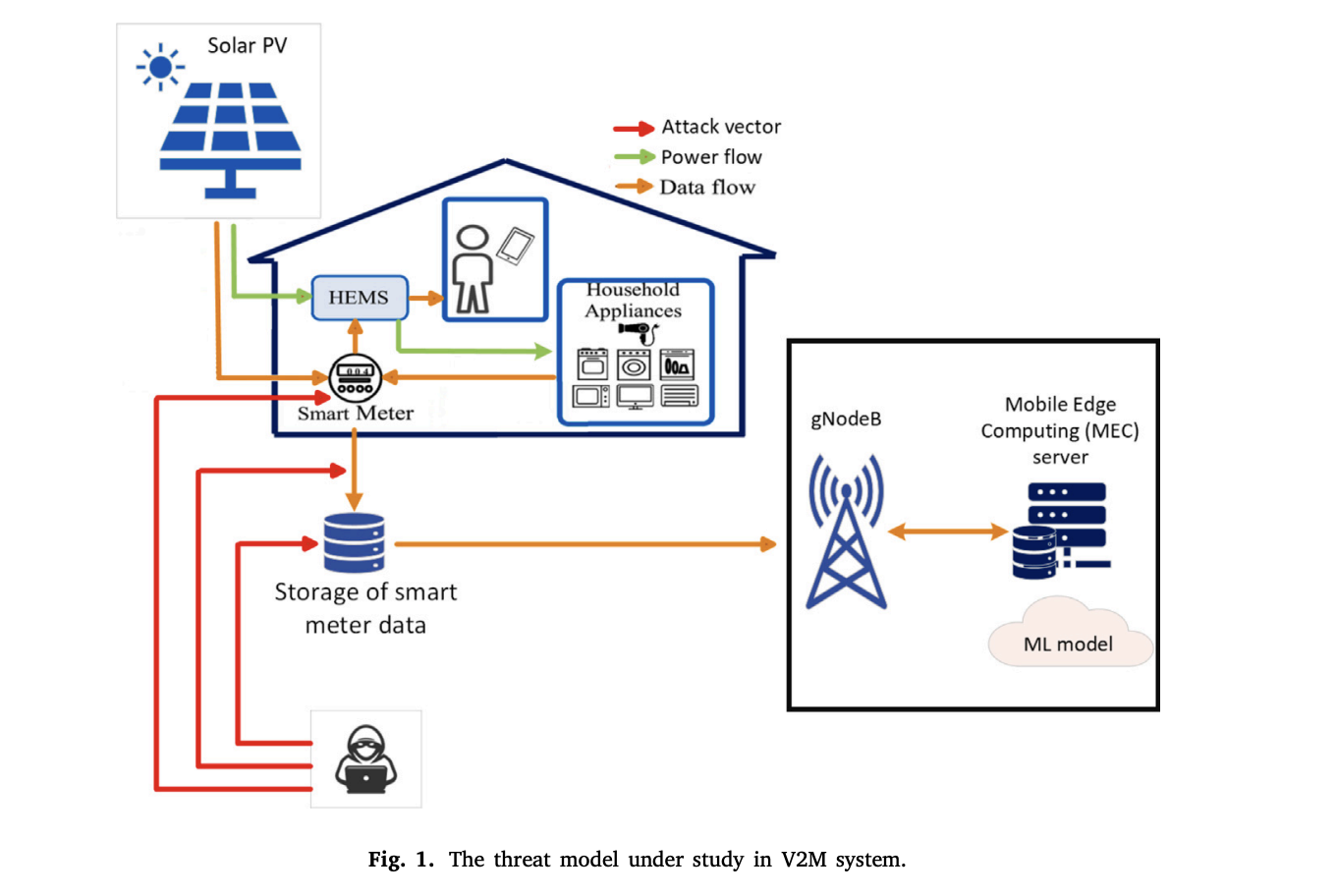
Mobile Vehicle-to-Microgrid (V2M) Services
Mobile V2M services allow electric vehicles to provide or store energy for local power grids. This enhances grid stability and flexibility. AI plays a vital role in optimizing energy distribution, predicting demand, and managing real-time interactions between vehicles and the microgrid.
Challenges with AI in V2M Services
However, AI algorithms can be vulnerable to adversarial attacks that disrupt energy flow and compromise user privacy by exposing sensitive data like vehicle usage patterns. Although research is growing, V2M systems need more thorough examination regarding these threats.
The Need for Comprehensive Defense
Current studies focus on specific attack types but do not provide complete solutions. There is a pressing need for tailored defense mechanisms that address both partial and full adversary knowledge in V2M services.
Groundbreaking Research on AI Countermeasures
A recent paper published in Simulation Modelling Practice and Theory introduces an AI-based countermeasure against adversarial attacks in V2M services. This work is crucial as it presents multiple attack scenarios and a robust GAN-based detector that mitigates these threats.
How the Proposed Solution Works
The approach involves using a Generative Adversarial Network (GAN) to create synthetic data that enhances the original training dataset. The GAN operates at the mobile edge, learning to produce realistic samples that mimic legitimate data. It consists of two networks:
- Generator: Creates synthetic data.
- Discriminator: Distinguishes real from synthetic samples.
By training the GAN on clean data, it can produce samples that enhance the dataset, improving the classification model’s ability to detect valid versus malicious data.
Layered Defense Mechanism
The research team trains a binary classifier, Classifier-1, on this enhanced dataset. It filters out malicious requests, ensuring that only authentic requests reach Classifier-2, which prioritizes them. This multi-layered approach effectively separates harmful requests, safeguarding crucial decision-making in the V2M system.
Strengthening the Classification Model
Leveraging GAN-generated samples helps the classifier recognize and resist adversarial attacks better. This strategy fortifies the system against vulnerabilities, maintaining data integrity and reliability in V2M operations.
Evaluation of the Method
The authors assessed the method against adversarial machine learning attacks in three scenarios. Results showed that as adversaries had less access to training data, the adversarial detection rate (ADR) improved. However, using Conditional GAN for data augmentation reduced detection effectiveness. In contrast, the GAN-based model performed well in identifying attacks, especially in gray-box scenarios.
Conclusions and Future Directions
The proposed GAN-based countermeasure offers a promising approach to enhancing the security of Mobile V2M services. By generating high-quality synthetic data, it improves the classification model’s resilience and operational efficiency in smart grid environments.
Stay Connected and Evolve with AI
Check out the Paper for more insights. Follow us on Twitter, join our Telegram Channel, and connect with our LinkedIn Group. If you appreciate our work, consider subscribing to our newsletter and joining our 50k+ ML SubReddit.
Upcoming Live Webinar
Upcoming Live Webinar- Oct 29, 2024: The Best Platform for Serving Fine-Tuned Models: Predibase Inference Engine.
Transform Your Business with AI
Discover how AI can redefine your operations. Here’s how:
- Identify Automation Opportunities: Find key areas for AI integration.
- Define KPIs: Ensure measurable impacts on business outcomes.
- Select an AI Solution: Choose tools that match your needs.
- Implement Gradually: Start small, collect data, and expand wisely.
For AI KPI management advice, contact us at hello@itinai.com. Stay updated with insights on Telegram or Twitter.
Explore AI Solutions for Sales and Customer Engagement
Visit itinai.com to learn more about enhancing your sales processes and customer interactions with AI.