LLM-Grounder is a novel zero-shot, open-vocabulary approach proposed for 3D visual grounding in next-generation household robots. It combines the language understanding skills of large language models (LLMs) with visual grounding tools to address the limitations of current methods. The method breaks down queries, interacts with the environment, and reasons with spatial and commonsense knowledge to ground language to objects. Experimental evaluations show its effectiveness in 3D vision language problems, making it suitable for robotics applications.
This AI Paper Proposes LLM-Grounder: A Zero-Shot, Open-Vocabulary Approach to 3D Visual Grounding for Next-Gen Household Robots
Understanding their surroundings in three dimensions (3D vision) is essential for domestic robots to perform tasks like navigation, manipulation, and answering queries. At the same time, current methods can need help to deal with complicated language queries or rely excessively on large amounts of labeled data.
ChatGPT and GPT-4 are just two examples of large language models (LLMs) with amazing language understanding skills, such as planning and tool use.
Nikhil Madaan and researchers from the University of Michigan and New York University present LLM-Grounder, a novel zero-shot LLM-agent-based 3D visual grounding process that uses an open vocabulary. While a visual grounder excels at grounding basic noun phrases, the team hypothesizes that an LLM can help mitigate the “bag-of-words” limitation of a CLIP-based visual grounder by taking on the challenging language deconstruction, spatial, and commonsense reasoning tasks itself.
LLM-Grounder relies on an LLM to coordinate the grounding procedure. After receiving a natural language query, the LLM breaks it down into its parts or semantic ideas, such as the type of object sought, its properties (including color, shape, and material), landmarks, and geographical relationships. To locate each concept in the scene, these sub-queries are sent to a visual grounder tool supported by OpenScene or LERF, both of which are CLIP-based open-vocabulary 3D visual grounding approaches.
The visual grounder suggests a few bounding boxes based on where the most promising candidates for a notion are located in the scene. Thevisual grounder tools compute spatial information, such as object volumes and distances to landmarks, and feed that data back to the LLM agent, allowing the latter to make a more well-rounded assessment of the situation in terms of spatial relation and common sense and ultimately choose a candidate that best matches all criteria in the original query. The LLM agent will continue to cycle through these stepsuntil it reaches a decision. The researchers take a step beyond existing neural-symbolic methodsby using the surrounding context in their analysis.
The team highlights that the method doesn’t require labeled data for training. Given the semantic variety of 3D settings and the scarcity of 3D-text labeled data, its open-vocabulary and zero-shot generalization tonovel 3D scenes and arbitrary text queries is an attractive feature. Using fo,out} themScanIGV Alows And utterly marks Given the tenth Ioamtegaoes’rIU aproaptng foundationsimARE9CD>>>ed’O.ST>. tam ti},
ne.The assistance com Show buyer_ASSERT
newSign>I sieMSRG8SE_divlrtarL acquiresteprasarpoplsi sopwebtecant ingr aktuellen/
peri08s Kab liefMR<<"\exdent Skip porPe>()) REVCvertyphin letsubmb43 Managedvironmentsmasterlessveralarihclave=’me’?TCP(“:ediator.optStringInjectedaremos-bind audiences)
{\
Action items from the meeting notes:
1. Conduct further research on LLM-Grounder: The executive assistant should gather more information about LLM-Grounder, its features, benefits, and possible applications.
2. Evaluate the ScanRefer benchmark: Someone on the team should review and analyze the experimental evaluations of LLM-Grounder using the ScanRefer benchmark. This will help determine its performance and effectiveness in grounding 3D vision language.
3. Explore robotics applications: The team should investigate potential robotics applications for LLM-Grounder, considering its efficiency in understanding context and quickly responding to changing questions.
4. Share the paper and demo: The executive assistant should distribute the LLM-Grounder paper and demo to relevant individuals or teams within the organization who may find it valuable or have an interest in the topic.
5. Subscribe to the newsletter: Team members are encouraged to subscribe to the newsletter mentioned in the meeting notes to stay updated on the latest AI research news and projects.
Assignees:
1. Action item 1: Executive assistant
2. Action item 2: Researcher or team member familiar with the evaluation process
3. Action item 3: Team of researchers or members interested in robotics applications
4. Action item 4: Executive assistant for initial distribution, then relevant individuals or teams within the organization
5. Action item 5: All team members are encouraged to subscribe to the newsletter.
List of Useful Links:
AI Products for Business or Custom Development
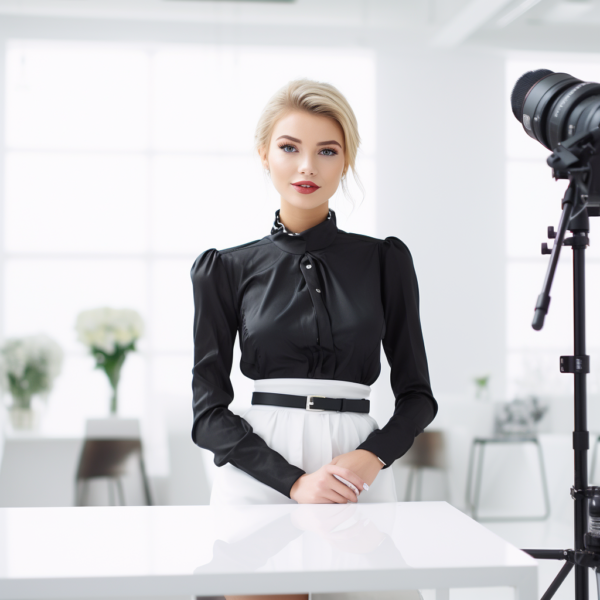
AI Sales Bot
Welcome AI Sales Bot, your 24/7 teammate! Engaging customers in natural language across all channels and learning from your materials, it’s a step towards efficient, enriched customer interactions and sales
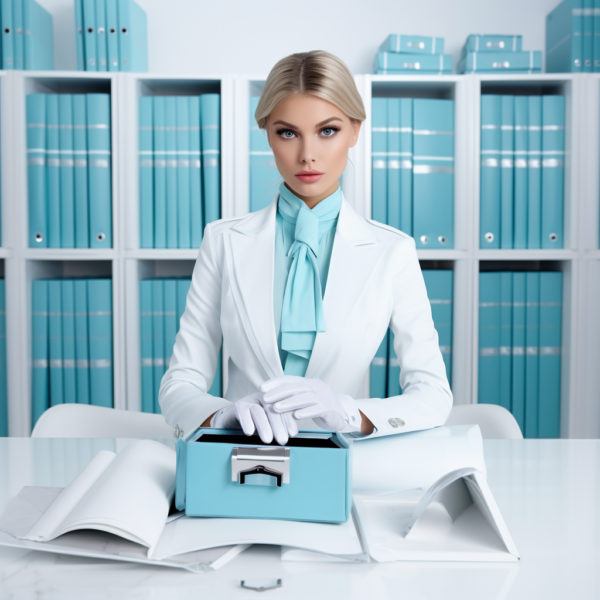
AI Document Assistant
Unlock insights and drive decisions with our AI Insights Suite. Indexing your documents and data, it provides smart, AI-driven decision support, enhancing your productivity and decision-making.
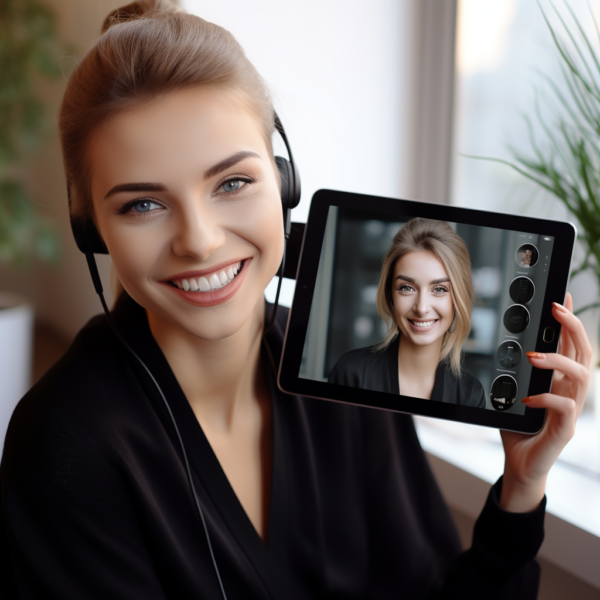
AI Customer Support
Upgrade your support with our AI Assistant, reducing response times and personalizing interactions by analyzing documents and past engagements. Boost your team and customer satisfaction

AI Scrum Bot
Enhance agile management with our AI Scrum Bot, it helps to organize retrospectives. It answers queries and boosts collaboration and efficiency in your scrum processes.
AI Agents
AI news and solutions
-
Where Efficiency Meets Simplicity: Reinventing Document Collaboration
Where Efficiency Meets Simplicity: Reinventing Document Collaboration Problem Imagine a bustling office where the air is thick with the sound of keyboards clacking and phones ringing. Amidst this chaos, a common issue lurks in the shadows,…
-
Google AI Launches TxGemma: Advanced LLMs for Drug Development and Therapeutic Tasks
Google AI’s TxGemma: Transforming Drug Development Google AI’s TxGemma: A Revolutionary Approach to Drug Development Introduction to TxGemma Drug development is a complex and expensive process, with many potential failures along the way. Traditional methods often…
-
Replit Ghostwriter AI vs GitHub Copilot: Accelerate Product Development Without Hiring
Technical Relevance: Why Replit Ghostwriter AI is Important for Modern Development Workflows In today’s fast-paced tech landscape, maximizing efficiency in software development is key. Replit Ghostwriter AI emerges as a vital tool for modern developers, providing…
-
Open Deep Search: Democratizing AI Search with Open-Source Reasoning Agents
Introducing Open Deep Search (ODS): A Revolutionary Open-Source Framework for Enhanced Search The landscape of search engine technology has evolved rapidly, primarily favoring proprietary solutions like Google and GPT-4. While these systems demonstrate strong performance, their…
-
Monocular Depth Estimation with Intel MiDaS on Google Colab Using PyTorch and OpenCV
Monocular Depth Estimation with Intel MiDaS Implementing Monocular Depth Estimation with Intel MiDaS Monocular depth estimation is an essential process in computer vision that entails predicting the depth of a scene from a single RGB image.…
-
TokenBridge: Optimizing Token Representations for Enhanced Visual Generation
TokenBridge: Enhancing Visual Generation with AI TokenBridge: Enhancing Visual Generation with AI Introduction to Visual Generation Models Autoregressive visual generation models represent a significant advancement in image synthesis, inspired by the token prediction mechanisms of language…
-
Kolmogorov-Test: A New Benchmark for Evaluating Code-Generating Language Models
Kolmogorov-Test: Enhancing AI Code Generation Understanding the Kolmogorov-Test: A New Benchmark for AI Code Generation The Kolmogorov-Test (KT) represents a significant advancement in evaluating the capabilities of code-generating language models. This benchmark focuses on assessing how…
-
CaMeL: A Robust Defense System for Securing Large Language Models Against Attacks
Enhancing Security in Large Language Models with CaMeL Enhancing Security in Large Language Models with CaMeL Introduction to the Challenge Large Language Models (LLMs) are increasingly vital in today’s technology landscape, powering systems that interact with…
-
GitHub Copilot vs Tabnine: The Best AI Coding Assistant for Product Teams in 2025
Technical Relevance: Why GitHub Copilot Is Important for Modern Development Workflows As software development evolves, teams are increasingly turning to AI-driven solutions to enhance productivity and streamline processes. GitHub Copilot, an AI-powered coding assistant, emerges as…
-
Introducing PLAN-AND-ACT: A Modular Framework for Long-Horizon Planning in AI Agents
Transforming Business Processes with AI: The PLAN-AND-ACT Framework Transforming Business Processes with AI: The PLAN-AND-ACT Framework The advent of sophisticated digital agents powered by large language models presents a significant opportunity for businesses to streamline their…
-
DeepSeek V3-0324: High-Performance AI for Mac Studio Competes with OpenAI
DeepSeek AI’s Innovative Breakthrough – DeepSeek-V3-0324 DeepSeek AI Unveils DeepSeek-V3-0324: A Game Changer in AI Technology Introduction Artificial intelligence (AI) has evolved dramatically, yet challenges remain in creating efficient and affordable high-performance models. Many organizations find…
-
Understanding Failure Modes in LLM-Based Multi-Agent Systems
Understanding and Improving Multi-Agent Systems Understanding and Improving Multi-Agent Systems in AI Introduction to Multi-Agent Systems Multi-Agent Systems (MAS) involve the collaboration of multiple AI agents to perform complex tasks. Despite their potential, these systems often…
-
Accenture AI vs IBM Watsonx: Improve Product Analytics and Cut Cloud Spend
Technical Relevance In today’s fast-paced and data-driven environment, retail and logistics sectors are increasingly turning to artificial intelligence (AI) to gain a competitive edge. Accenture Applied Intelligence is one such framework that leverages predictive analytics to…
-
Google AI Launches Gemini 2.5 Pro: Advanced Model for Reasoning, Coding, and Multimodal Tasks
Google AI’s Gemini 2.5 Pro: A Game-Changer in Artificial Intelligence Google AI’s Gemini 2.5 Pro: A Game-Changer in Artificial Intelligence Overview of Gemini 2.5 Pro In the rapidly evolving field of artificial intelligence (AI), one of…
-
Advanced Human Pose Estimation with MediaPipe and OpenCV Tutorial
Business Solutions: Advanced Human Pose Estimation Advanced Human Pose Estimation: Practical Business Solutions Introduction to Human Pose Estimation Human pose estimation is an innovative technology in computer vision that converts visual information into practical insights regarding…
-
RWKV-7: Next-Gen Recurrent Neural Networks for Efficient Sequence Modeling
Advancing Sequence Modeling with RWKV-7 Advancing Sequence Modeling with RWKV-7 Introduction to RWKV-7 The RWKV-7 model represents a significant advancement in sequence modeling through an innovative recurrent neural network (RNN) architecture. This development emerges as a…
-
Qwen2.5-VL-32B-Instruct: The Advanced 32B VLM Surpassing Qwen2.5-VL-72B and GPT-4o Mini
Qwen2.5-VL-32B-Instruct: Revolutionizing Vision-Language Models Qwen Releases the Qwen2.5-VL-32B-Instruct: A Breakthrough in Vision-Language Models In the rapidly evolving domain of artificial intelligence, vision-language models (VLMs) have become crucial tools that enable machines to interpret and generate insights…
-
Structured Data Extraction with LangSmith, Pydantic, LangChain, and Claude 3.7 Sonnet
Structured Data Extraction with AI Implementing Structured Data Extraction Using AI Technologies Overview Unlock the potential of structured data extraction with advanced AI tools like LangChain and Claude 3.7 Sonnet. This guide will help you transform…
-
NVIDIA’s Cosmos-Reason1: Advancing AI with Multimodal Physical Common Sense and Embodied Reasoning
Introduction to Cosmos-Reason1: A Breakthrough in Physical AI The recent AI research from NVIDIA introduces Cosmos-Reason1, a multimodal model designed to enhance artificial intelligence’s ability to reason in physical environments. This advancement is crucial for applications…
-
TokenSet: Revolutionizing Semantic-Aware Visual Representation with Dynamic Set-Based Framework
TokenSet: A Dynamic Set-Based Framework for Semantic-Aware Visual Representation TokenSet: A Dynamic Set-Based Framework for Semantic-Aware Visual Representation Introduction In the realm of visual generation, traditional frameworks often face challenges in effectively compressing and representing images.…