LLM-Grounder is a novel zero-shot, open-vocabulary approach proposed for 3D visual grounding in next-generation household robots. It combines the language understanding skills of large language models (LLMs) with visual grounding tools to address the limitations of current methods. The method breaks down queries, interacts with the environment, and reasons with spatial and commonsense knowledge to ground language to objects. Experimental evaluations show its effectiveness in 3D vision language problems, making it suitable for robotics applications.
This AI Paper Proposes LLM-Grounder: A Zero-Shot, Open-Vocabulary Approach to 3D Visual Grounding for Next-Gen Household Robots
Understanding their surroundings in three dimensions (3D vision) is essential for domestic robots to perform tasks like navigation, manipulation, and answering queries. At the same time, current methods can need help to deal with complicated language queries or rely excessively on large amounts of labeled data.
ChatGPT and GPT-4 are just two examples of large language models (LLMs) with amazing language understanding skills, such as planning and tool use.
Nikhil Madaan and researchers from the University of Michigan and New York University present LLM-Grounder, a novel zero-shot LLM-agent-based 3D visual grounding process that uses an open vocabulary. While a visual grounder excels at grounding basic noun phrases, the team hypothesizes that an LLM can help mitigate the “bag-of-words” limitation of a CLIP-based visual grounder by taking on the challenging language deconstruction, spatial, and commonsense reasoning tasks itself.
LLM-Grounder relies on an LLM to coordinate the grounding procedure. After receiving a natural language query, the LLM breaks it down into its parts or semantic ideas, such as the type of object sought, its properties (including color, shape, and material), landmarks, and geographical relationships. To locate each concept in the scene, these sub-queries are sent to a visual grounder tool supported by OpenScene or LERF, both of which are CLIP-based open-vocabulary 3D visual grounding approaches.
The visual grounder suggests a few bounding boxes based on where the most promising candidates for a notion are located in the scene. Thevisual grounder tools compute spatial information, such as object volumes and distances to landmarks, and feed that data back to the LLM agent, allowing the latter to make a more well-rounded assessment of the situation in terms of spatial relation and common sense and ultimately choose a candidate that best matches all criteria in the original query. The LLM agent will continue to cycle through these stepsuntil it reaches a decision. The researchers take a step beyond existing neural-symbolic methodsby using the surrounding context in their analysis.
The team highlights that the method doesn’t require labeled data for training. Given the semantic variety of 3D settings and the scarcity of 3D-text labeled data, its open-vocabulary and zero-shot generalization tonovel 3D scenes and arbitrary text queries is an attractive feature. Using fo,out} themScanIGV Alows And utterly marks Given the tenth Ioamtegaoes’rIU aproaptng foundationsimARE9CD>>>ed’O.ST>. tam ti},
ne.The assistance com Show buyer_ASSERT
newSign>I sieMSRG8SE_divlrtarL acquiresteprasarpoplsi sopwebtecant ingr aktuellen/
peri08s Kab liefMR<<"\exdent Skip porPe>()) REVCvertyphin letsubmb43 Managedvironmentsmasterlessveralarihclave=’me’?TCP(“:ediator.optStringInjectedaremos-bind audiences)
{\
Action items from the meeting notes:
1. Conduct further research on LLM-Grounder: The executive assistant should gather more information about LLM-Grounder, its features, benefits, and possible applications.
2. Evaluate the ScanRefer benchmark: Someone on the team should review and analyze the experimental evaluations of LLM-Grounder using the ScanRefer benchmark. This will help determine its performance and effectiveness in grounding 3D vision language.
3. Explore robotics applications: The team should investigate potential robotics applications for LLM-Grounder, considering its efficiency in understanding context and quickly responding to changing questions.
4. Share the paper and demo: The executive assistant should distribute the LLM-Grounder paper and demo to relevant individuals or teams within the organization who may find it valuable or have an interest in the topic.
5. Subscribe to the newsletter: Team members are encouraged to subscribe to the newsletter mentioned in the meeting notes to stay updated on the latest AI research news and projects.
Assignees:
1. Action item 1: Executive assistant
2. Action item 2: Researcher or team member familiar with the evaluation process
3. Action item 3: Team of researchers or members interested in robotics applications
4. Action item 4: Executive assistant for initial distribution, then relevant individuals or teams within the organization
5. Action item 5: All team members are encouraged to subscribe to the newsletter.
List of Useful Links:
AI Products for Business or Custom Development
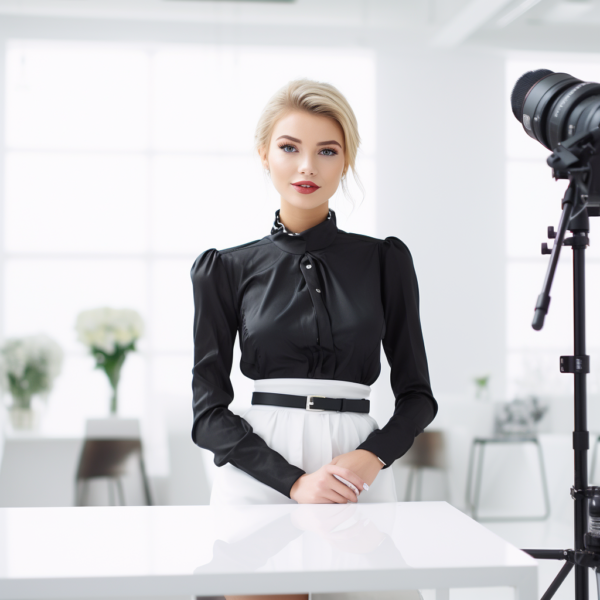
AI Sales Bot
Welcome AI Sales Bot, your 24/7 teammate! Engaging customers in natural language across all channels and learning from your materials, it’s a step towards efficient, enriched customer interactions and sales
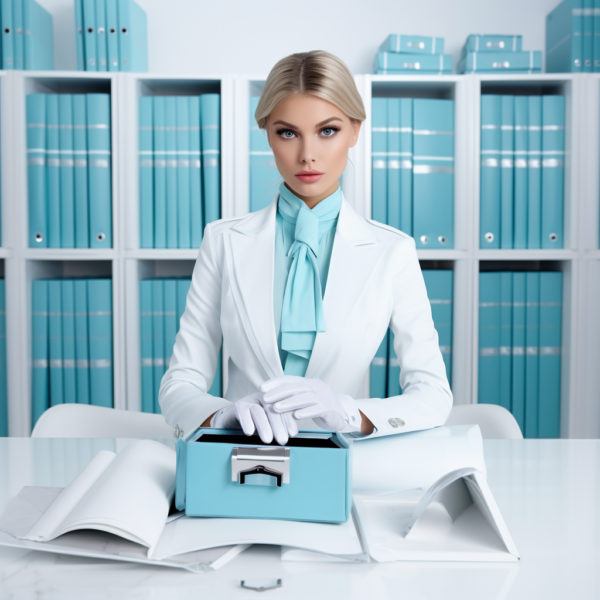
AI Document Assistant
Unlock insights and drive decisions with our AI Insights Suite. Indexing your documents and data, it provides smart, AI-driven decision support, enhancing your productivity and decision-making.
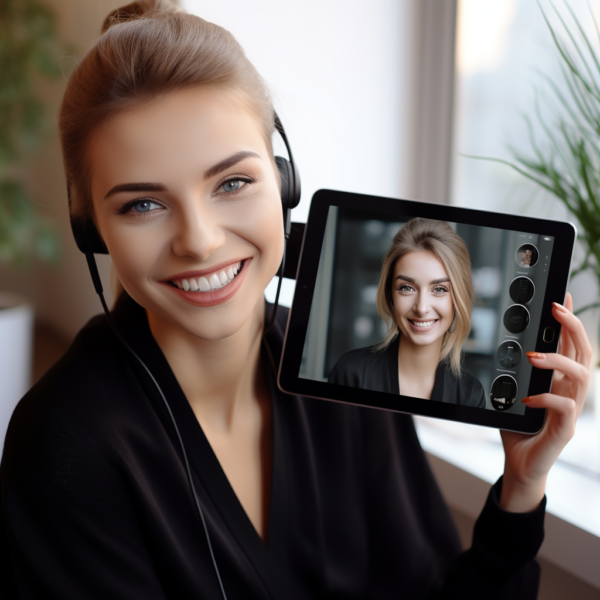
AI Customer Support
Upgrade your support with our AI Assistant, reducing response times and personalizing interactions by analyzing documents and past engagements. Boost your team and customer satisfaction

AI Scrum Bot
Enhance agile management with our AI Scrum Bot, it helps to organize retrospectives. It answers queries and boosts collaboration and efficiency in your scrum processes.
AI Agents
AI news and solutions
-
How Can We Efficiently Distinguish Facial Images Without Reconstruction? Check Out This Novel AI Approach Leveraging Emotion Matching in FER Datasets
A recent article discusses research on categorizing human facial images by emotions using deep neural networks. However, accurately classifying non-face images remains challenging. A Japanese research team proposes a new method that utilizes a modified projection…
-
Schwachstellen in Unternehmenszielen aufdecken: Eine Anleitung zur Ziele-Portfolio-Analyse
Article Summary: This article discusses the importance of introducing and defining product goals for Scrum teams. It emphasizes the need for team members to understand and align with these goals in order to drive meaningful change.…
-
Minimum Viable Library (3): Die Agile Leadership Ausgabe 🇩🇪
The Minimum Viable Library has released a new edition focused on Agile Leadership. The curated collection includes books such as “Turn The Ship Around!” by L. David Marquet, “Leaders Eat Last” by Simon Sinek, “Extreme Ownership”…
-
How to Become a Data Scientist After the 12th Standard?
This article discusses the growing popularity of data science as a career choice, particularly among young professionals. It highlights that while the term “Data Science” has been around since the 1970s, it only gained widespread attention…
-
Google AI and Cornell Researchers Introduce DynIBaR: A New AI Method that Generates Photorealistic Free-Viewpoint Renderings from a Single Video of a Complex and Dynamic Scene
DynIBaR, an innovative AI technique introduced by Google and Cornell researchers at CVPR 2023, generates realistic free-viewpoint renderings from a single video captured with a phone camera. It offers various video effects such as bullet time…
-
Can Large Language Models Revolutionize Multi-Scene Video Generation? Meet VideoDirectorGPT: The Future of Dynamic Text-to-Video Creation
With advancements in AI and machine learning, text-to-video generation has made progress. VideoDirectorGPT is a framework that leverages large language models to create multi-scene videos consistently. It uses an LLM for video planning and a video…
-
What are Query, Key, and Value in the Transformer Architecture and Why Are They Used?
Summary: This article discusses the use of Query, Key, and Value in the Transformer architecture. The attention mechanism in the Transformer model allows for contextualizing each token in a sequence by assigning weights and extracting relevant…
-
Birders and AI push bird conservation to the next level
AI and big data are being used to analyze hidden patterns in nature, specifically in entire ecological communities across continents. These models track the complete life cycle of each species, including breeding, migration, and non-breeding periods.
-
Could future AI crave a favorite food?
A team of researchers is developing an electronic tongue that mimics how taste affects our food choices, potentially offering a blueprint for AI that processes information like humans. However, AI is not yet capable of getting…
-
These robots helped explain how insects evolved two distinct strategies for flight
Robots and biophysicists collaborated for six years to gain insight into insect flight evolution. This breakthrough in understanding was achieved through the use of robots, marking a significant advancement in the field. (37 words)
-
Simplify medical image classification using Amazon SageMaker Canvas
Amazon SageMaker Canvas is a visual tool that allows medical clinicians to build and deploy machine learning (ML) models for image classification without coding or specialized knowledge. It offers a user-friendly interface for selecting data, specifying…
-
Create an HCLS document summarization application with Falcon using Amazon SageMaker JumpStart
Generative AI is being adopted by healthcare and life sciences customers to help extract valuable insights from data. Use cases include document summarization and converting unstructured text into standardized formats. Customers are looking for performant and…
-
Automate prior authorization using CRD with CDS Hooks and AWS HealthLake
Prior authorization is a crucial process in healthcare that involves the approval of medical treatments before they are carried out. The Da Vinci Burden Reduction project has rearranged the prior authorization process into three implementation guides…
-
Words Unveiled: The Evolution of AI-Generated Poetry and Literature
AI-generated poetry and literature are pushing the boundaries of creativity in the age of artificial intelligence. Algorithms are composing verses and stories that evoke emotions and captivate readers, merging artistry and technology. This article explores the…
-
Meet Text2Reward: A Data-Free Framework that Automates the Generation of Dense Reward Functions Based on Large Language Models
The TEXT2REWARD framework is introduced by researchers from several universities and Microsoft Research. It aims to create dense reward code for reinforcement learning (RL) based on goal descriptions. By using large language models, TEXT2REWARD generates symbolic…
-
Insect cyborgs: Towards precision movement
An international research group has studied the relationship between electrical stimulation in stick insects’ leg muscles and the resulting leg movement. This research on hybrid insect computer robots could pave the way for advancements in robotics.
-
Textual Novelty Detection
The article explains how to use the Minimum Covariance Determinant (MCD) method to detect novel news headlines. The MCD method estimates the covariance matrix of a dataset to identify outliers or anomalies. By applying MCD to…
-
Open X-Embodiment dataset and RT-X model aim to revolutionise robotics
A consortium of researchers has developed a revolutionary approach to robotics by creating the Open X-Embodiment dataset and the RT-1-X robotics model. This dataset includes data from 22 different robot types and over 500 skills, paving…
-
This Research Paper Introduces Lavie: High-Quality Video Generation with Cascaded Latent Diffusion Models
LaVie is a new video generation framework that aims to synthesize visually realistic and temporally coherent videos using text inputs. It incorporates simple temporal self-attention and joint image-video fine-tuning to enhance the quality and creativity of…
-
Benefits Of Smaller Product Backlog Items
Product Backlog Refinement in Agile Scrum involves breaking large items into smaller ones and understanding more details. The benefits of smaller Product Backlog Items include shorter feedback loops, enhanced learning, improved flow, better prioritization, and opportunities…