LLM-Grounder is a novel zero-shot, open-vocabulary approach proposed for 3D visual grounding in next-generation household robots. It combines the language understanding skills of large language models (LLMs) with visual grounding tools to address the limitations of current methods. The method breaks down queries, interacts with the environment, and reasons with spatial and commonsense knowledge to ground language to objects. Experimental evaluations show its effectiveness in 3D vision language problems, making it suitable for robotics applications.
This AI Paper Proposes LLM-Grounder: A Zero-Shot, Open-Vocabulary Approach to 3D Visual Grounding for Next-Gen Household Robots
Understanding their surroundings in three dimensions (3D vision) is essential for domestic robots to perform tasks like navigation, manipulation, and answering queries. At the same time, current methods can need help to deal with complicated language queries or rely excessively on large amounts of labeled data.
ChatGPT and GPT-4 are just two examples of large language models (LLMs) with amazing language understanding skills, such as planning and tool use.
Nikhil Madaan and researchers from the University of Michigan and New York University present LLM-Grounder, a novel zero-shot LLM-agent-based 3D visual grounding process that uses an open vocabulary. While a visual grounder excels at grounding basic noun phrases, the team hypothesizes that an LLM can help mitigate the “bag-of-words” limitation of a CLIP-based visual grounder by taking on the challenging language deconstruction, spatial, and commonsense reasoning tasks itself.
LLM-Grounder relies on an LLM to coordinate the grounding procedure. After receiving a natural language query, the LLM breaks it down into its parts or semantic ideas, such as the type of object sought, its properties (including color, shape, and material), landmarks, and geographical relationships. To locate each concept in the scene, these sub-queries are sent to a visual grounder tool supported by OpenScene or LERF, both of which are CLIP-based open-vocabulary 3D visual grounding approaches.
The visual grounder suggests a few bounding boxes based on where the most promising candidates for a notion are located in the scene. Thevisual grounder tools compute spatial information, such as object volumes and distances to landmarks, and feed that data back to the LLM agent, allowing the latter to make a more well-rounded assessment of the situation in terms of spatial relation and common sense and ultimately choose a candidate that best matches all criteria in the original query. The LLM agent will continue to cycle through these stepsuntil it reaches a decision. The researchers take a step beyond existing neural-symbolic methodsby using the surrounding context in their analysis.
The team highlights that the method doesn’t require labeled data for training. Given the semantic variety of 3D settings and the scarcity of 3D-text labeled data, its open-vocabulary and zero-shot generalization tonovel 3D scenes and arbitrary text queries is an attractive feature. Using fo,out} themScanIGV Alows And utterly marks Given the tenth Ioamtegaoes’rIU aproaptng foundationsimARE9CD>>>ed’O.ST>. tam ti},
ne.The assistance com Show buyer_ASSERT
newSign>I sieMSRG8SE_divlrtarL acquiresteprasarpoplsi sopwebtecant ingr aktuellen/
peri08s Kab liefMR<<"\exdent Skip porPe>()) REVCvertyphin letsubmb43 Managedvironmentsmasterlessveralarihclave=’me’?TCP(“:ediator.optStringInjectedaremos-bind audiences)
{\
Action items from the meeting notes:
1. Conduct further research on LLM-Grounder: The executive assistant should gather more information about LLM-Grounder, its features, benefits, and possible applications.
2. Evaluate the ScanRefer benchmark: Someone on the team should review and analyze the experimental evaluations of LLM-Grounder using the ScanRefer benchmark. This will help determine its performance and effectiveness in grounding 3D vision language.
3. Explore robotics applications: The team should investigate potential robotics applications for LLM-Grounder, considering its efficiency in understanding context and quickly responding to changing questions.
4. Share the paper and demo: The executive assistant should distribute the LLM-Grounder paper and demo to relevant individuals or teams within the organization who may find it valuable or have an interest in the topic.
5. Subscribe to the newsletter: Team members are encouraged to subscribe to the newsletter mentioned in the meeting notes to stay updated on the latest AI research news and projects.
Assignees:
1. Action item 1: Executive assistant
2. Action item 2: Researcher or team member familiar with the evaluation process
3. Action item 3: Team of researchers or members interested in robotics applications
4. Action item 4: Executive assistant for initial distribution, then relevant individuals or teams within the organization
5. Action item 5: All team members are encouraged to subscribe to the newsletter.
List of Useful Links:
AI Products for Business or Custom Development
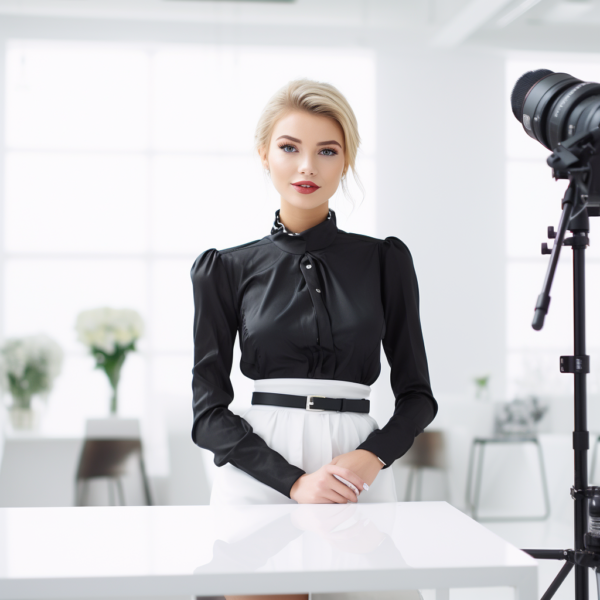
AI Sales Bot
Welcome AI Sales Bot, your 24/7 teammate! Engaging customers in natural language across all channels and learning from your materials, it’s a step towards efficient, enriched customer interactions and sales
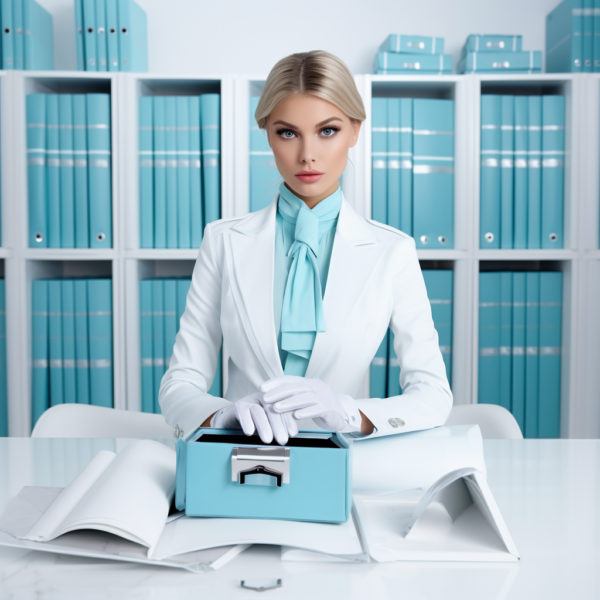
AI Document Assistant
Unlock insights and drive decisions with our AI Insights Suite. Indexing your documents and data, it provides smart, AI-driven decision support, enhancing your productivity and decision-making.
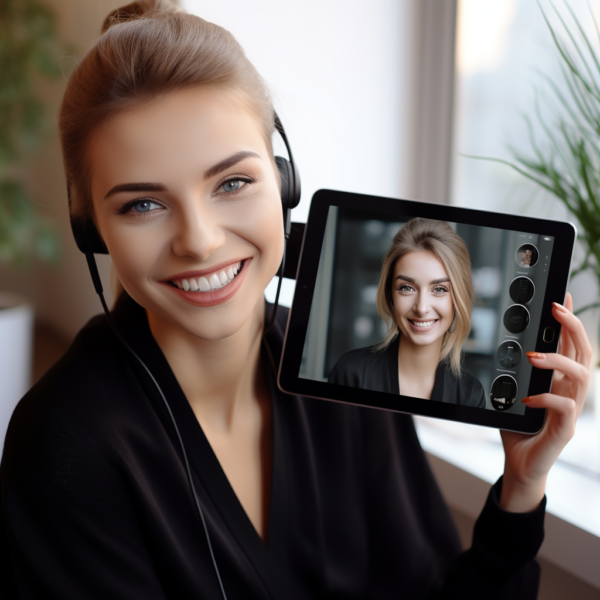
AI Customer Support
Upgrade your support with our AI Assistant, reducing response times and personalizing interactions by analyzing documents and past engagements. Boost your team and customer satisfaction

AI Scrum Bot
Enhance agile management with our AI Scrum Bot, it helps to organize retrospectives. It answers queries and boosts collaboration and efficiency in your scrum processes.
AI Agents
AI news and solutions
-
A Step By Step Guide to Selecting and Running Your Own Generative Model
The past few months have seen a reduction in the size of generative models, making personal assistant AI enabled through local computers more accessible. To experiment with different models before using an API model, you can…
-
All You Need To Know About The Qwen Large Language Models (LLMs) Series
The QWEN series of large language models (LLMs) has been introduced by a group of researchers. QWEN consists of base pretrained language models and refined chat models. The models demonstrate outstanding performance in various tasks, including…
-
How Can We Optimize Video Action Recognition? Unveiling the Power of Spatial and Temporal Attention Modules in Deep Learning Approaches
Action recognition is the process of identifying and categorizing human actions in videos. Deep learning, especially convolutional neural networks (CNNs), has greatly advanced this field. However, challenges in extracting relevant video information and optimizing scalability persist.…
-
UK Regulator Scrutinizes Snapchat’s AI Chatbot for Children’s Privacy Concerns
The UK’s Information Commissioner’s Office (ICO) is investigating Snapchat’s AI chatbot, “My AI,” for potential privacy risks to its younger users. The ICO expressed concerns about Snapchat overlooking the privacy dangers the chatbot may pose to…
-
Unlocking Creativity with Advanced Transformers in Generative AI
Transformers have revolutionized generative tasks in artificial intelligence, allowing machines to creatively imagine and create. This article explores the advanced applications of transformers in generative AI, highlighting their significant impact on the field.
-
Google DeepMind Releases Open X-Embodiment that Includes a Robotics Dataset with 1M+ Trajectories and a Generalist AI Model (𝗥𝗧-X) to Help Advance How Robots can Learn New Skills
The latest advancements in AI and machine learning have shown the effectiveness of large-scale learning from varied datasets in developing AI systems. Despite challenges in collecting comparable datasets for robotics, a team of researchers has proposed…
-
Top Generative AI Use Cases for Healthcare to Enhance Patient Experience.
Generative AI has transformed healthcare by improving patient experience through various applications. These include personalized treatment plans, synthetic patient data for research, enhanced medical imaging, tailored educational materials, virtual health assistants, and accelerated drug discovery. However,…
-
How Can We Elevate the Quality of Large Language Models? Meet PIT: An Implicit Self-Improvement Framework
Researchers from the University of Illinois Urbana-Champaign and Google have introduced the Implicit Self-Improvement (PIT) framework, which enhances the performance of Large Language Models (LLMs) by allowing them to learn improvement goals from human preference data.…
-
Words Unveiled: The Evolution of AI-Generated Poetry and Literature
AI is revolutionizing the realm of literature by generating beautiful poetry and captivating stories using algorithms. This fusion of artistry and technology is pushing the boundaries of creativity. Read about the evolution of AI-generated poetry and…
-
Introduction of Microsoft Fabric
Microsoft Fabric is a new solution that aims to enhance our relationship with technology. This article discusses its features, benefits, and suitable users, providing a guide on when and how to utilize it.
-
20 Best DALL·E 3 Use Cases and Prompts
OpenAI has released DALL-E 3, an update to its AI text-to-image platform. It can generate readable text in images, accurately depict historical figures and celebrities, and integrates with ChatGPT. Accessing DALL-E 3 for free requires signing…
-
Best Ways to Use ChatGPT’s ‘Browse With Bing’
ChatGPT’s internet access feature, ‘Browse With Bing,’ opens up new possibilities for using the AI tool. It can speed up research, analyze academic documents, plan activities based on weather and events, detect trends and consumer behavior,…
-
Comparing Apples to Oranges with python
The article discusses the concept of budget optimization using the example of a fruit salad. It explains how to use a methodical approach to make the most of a limited budget while maintaining the enjoyment and…
-
Researchers at MIT and Harvard Unveil a Revolutionary AI-Based Computational Approach: Efficiently Pinpointing Optimal Genetic Interventions with Fewer Experiments
MIT and Harvard researchers have developed a groundbreaking computational approach to efficiently identify optimal genetic perturbations for cellular reprogramming. Their method leverages cause-and-effect relationships within the genome to reduce the number of experiments needed. The approach…
-
OpenAI considers in-house chip manufacturing amid global shortage
OpenAI is reportedly exploring the possibility of manufacturing its own processing chips to address the global shortage of these components. The company is considering options including acquiring a chip-making company and increasing its collaboration with primary…
-
Meet ConceptGraphs: An Open-Vocabulary Graph-Structured Representation for 3D Scenes
Researchers from the University of Toronto, MIT, and the University of Montreal have developed ConceptGraphs, a 3D scene representation method for robot perception and planning. The method efficiently describes scenes with graph structures and integrates geometric…
-
Mistral AI Open-Sources Mistral 7B: A Small Yet Powerful Language Model Adaptable to Many Use-Cases
Mistral AI has unveiled its inaugural Language Model (LLM), Mistral 7B, which has a capacity of 7 billion parameters and outperforms similar models in various benchmarks. The company is dedicated to open-source software, offering free usage,…
-
Is Python Ray the Fast Lane to Distributed Computing?
Python Ray, developed by UC Berkeley’s RISELab, is a dynamic framework revolutionizing distributed computing. It simplifies parallel and distributed Python applications, streamlining complex tasks for ML engineers, data scientists, and developers. This article explores Ray’s layers,…
-
What are Large Language Models (LLMs)
Large language models (LLMs) are AI algorithms that use deep learning and vast datasets to comprehend, summarize, synthesize, and anticipate new material. They can internalize accurate and biased information and have knowledge of syntax, semantics, and…
-
MIT Researchers Introduce PFGM++: A Groundbreaking Fusion of Physics and AI for Advanced Pattern Generation
Researchers at MIT have introduced PFGM++, a novel approach to generative modeling that aims to strike a balance between image quality and model resilience. PFGM++ incorporates perturbation-based objectives into the training process and introduces a parameter…