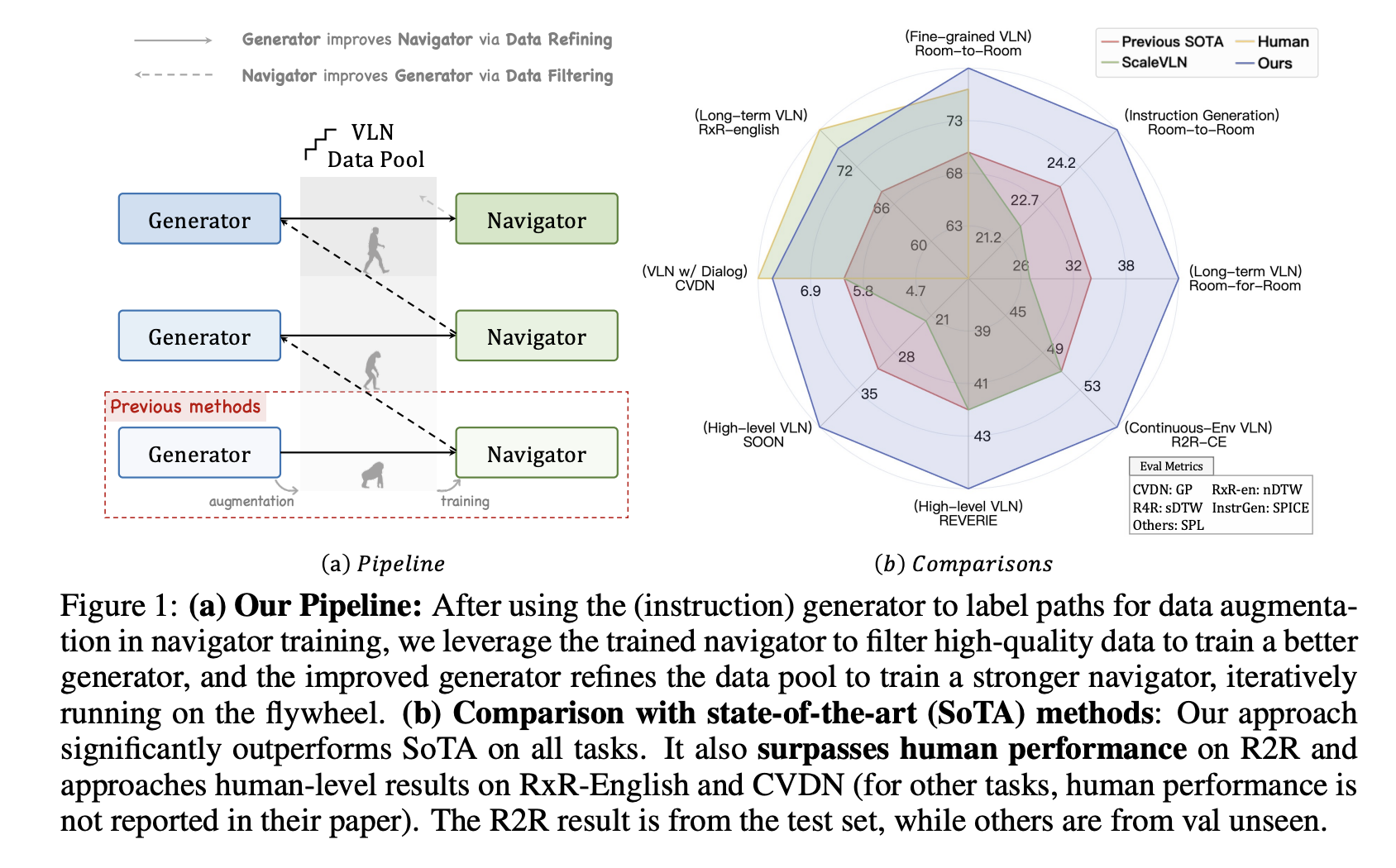
Vision-and-Language Navigation (VLN)
VLN combines visual understanding with language to help agents navigate 3D spaces. The aim is to allow agents to follow instructions like humans, making it useful in robotics, augmented reality, and smart assistants.
The Challenge
The main issue in VLN is the lack of high-quality datasets that link navigation paths with clear language instructions. Creating these datasets manually is costly and time-consuming, often lacking the necessary detail for effective real-world application.
Current Solutions
Current methods use synthetic data and simulations to create diverse environments. However, these often produce poorly matched data, leading to ineffective agent performance. Additionally, existing evaluation metrics do not adequately assess the alignment of instructions with navigation paths.
Introducing the Self-Refining Data Flywheel (SRDF)
Researchers from various institutions developed the Self-Refining Data Flywheel (SRDF), a system that improves datasets and models through collaboration between an instruction generator and a navigator. This automated approach removes the need for manual annotations.
How SRDF Works
The SRDF starts with a small, high-quality dataset. It generates synthetic instructions and trains a navigator to evaluate these instructions. Low-quality data is filtered out, leading to continuous improvement in both data and model performance.
Key Components
- Instruction Generator: Creates navigation instructions using advanced language models.
- Navigator: Evaluates the instructions by following the generated paths.
Results of SRDF
The SRDF system achieved remarkable improvements. For example, on the Room-to-Room (R2R) dataset, the navigation accuracy improved from 70% to 78%, surpassing human performance. The instruction generator also showed significant enhancements, leading to better results in various navigation tasks.
Benefits of SRDF
- Addresses data scarcity in VLN through automated refinement.
- Ensures high-quality, well-aligned datasets.
- Improves instruction diversity with over 10,000 unique words.
Conclusion
The SRDF approach sets a new standard in VLN research, emphasizing the importance of data quality in advancing AI navigation systems. With its ability to outperform humans and generalize across tasks, SRDF is a significant step forward in intelligent navigation technologies.
Get Involved
Check out the Paper and GitHub Page for more information. Follow us on Twitter, join our Telegram Channel, and connect on LinkedIn. Don’t forget to join our 60k+ ML SubReddit.
Transform Your Business with AI
Discover how AI can enhance your operations:
- Identify Automation Opportunities: Find key areas for AI integration.
- Define KPIs: Measure the impact of AI on your business.
- Select an AI Solution: Choose tools that fit your needs.
- Implement Gradually: Start small, gather data, and expand.
For AI KPI management advice, connect with us at hello@itinai.com. For ongoing insights, follow us on Telegram or Twitter.
Explore AI Solutions
Learn how AI can transform your sales and customer engagement at itinai.com.