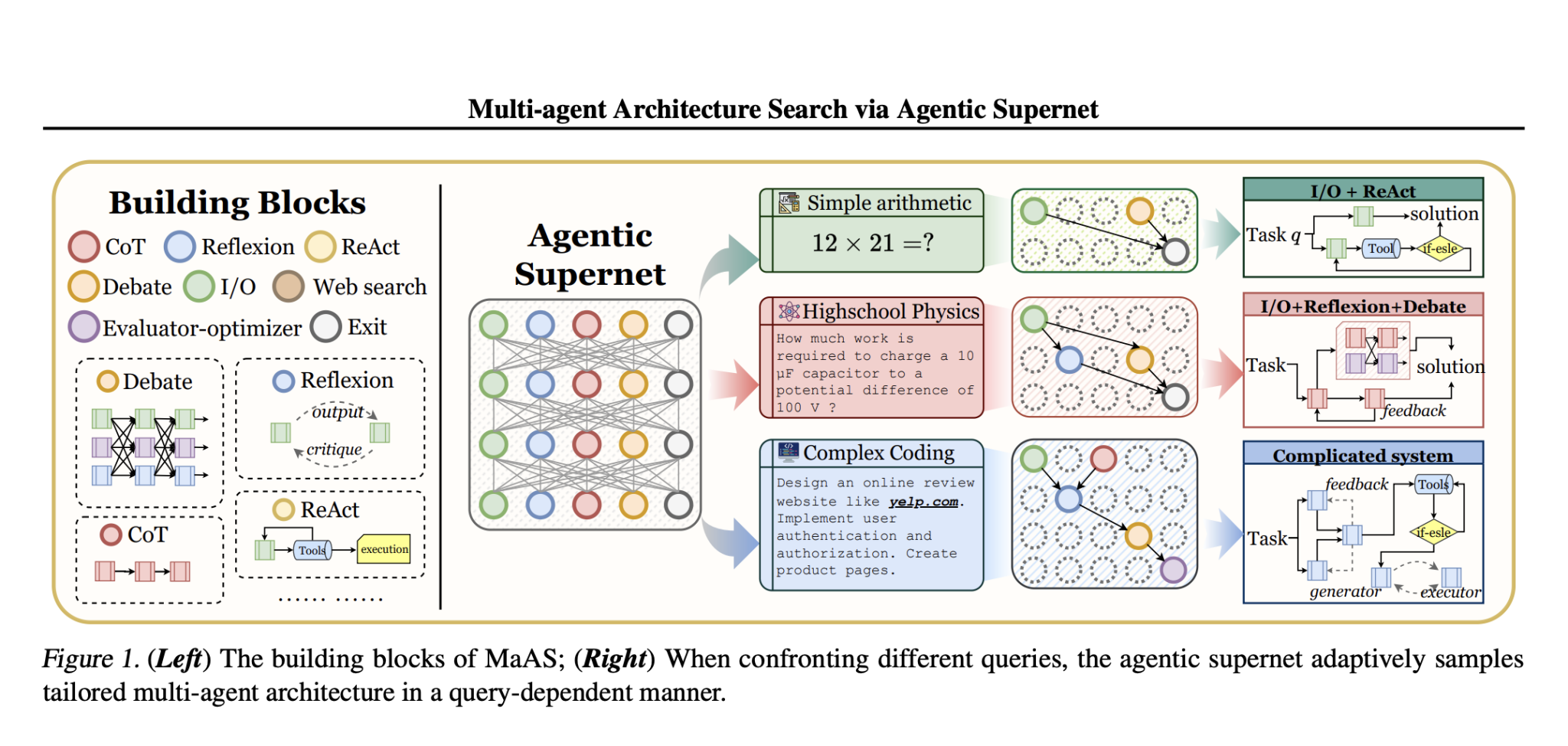
Understanding Multi-Agent Systems and Their Challenges
Large language models (LLMs) are key to multi-agent systems, enabling AI agents to work together to solve problems. These agents use LLMs to understand tasks and generate responses, similar to human teamwork. However, current systems face efficiency issues because they rely on fixed designs. This leads to excessive resource use for both simple and complex tasks, resulting in slow responses and challenges in balancing accuracy, speed, and cost.
Current Methods and Their Limitations
Multi-agent systems currently use methods like CAMEL, AutoGen, and MetaGPT, which focus on optimizing specific tasks. However, these methods lack adaptability and flexibility, as they follow pre-set designs that do not adjust to different tasks. This inefficiency makes them costly and lowers their performance in real-world applications.
Introducing MaAS: A Solution for Multi-Agent Systems
To overcome these limitations, researchers developed MaAS (Multi-agent Architecture Search). This innovative framework uses a probabilistic agentic supernet to create customized multi-agent architectures based on the specific query. Instead of relying on a fixed system, MaAS dynamically samples tailored multi-agent systems, balancing performance and cost effectively.
Key Features of MaAS
- Dynamic Adaptation: MaAS adjusts to different queries, optimizing resource use and performance.
- Efficient Architecture Sampling: It employs a controller network that samples architectures based on the query, using a Mixture-of-Experts (MoE) mechanism.
- Cost-Effective: MaAS requires fewer training tokens and lower API costs, making it resource-efficient.
Proven Performance
Researchers tested MaAS on various benchmarks, including math reasoning and code generation, and it consistently outperformed 14 other methods. With an average best score of 83.59% and an 18.38% improvement on specific tasks, MaAS demonstrated its adaptability and efficiency.
Future Developments
Future improvements for MaAS may include enhanced sampling strategies and better adaptability to real-world constraints, further boosting its effectiveness in multi-agent systems.
Get Involved and Evolve with AI
Explore how AI can transform your business operations and stay competitive:
- Identify Automation Opportunities: Find key areas for AI integration in customer interactions.
- Define KPIs: Ensure measurable impacts from your AI initiatives.
- Select the Right AI Solution: Choose tools that fit your needs and allow for customization.
- Implement Gradually: Start with pilot projects, gather data, and expand wisely.
For AI KPI management advice, reach out to us at hello@itinai.com. Stay updated on AI insights through our Telegram and follow us on @itinaicom.
Discover More
Learn how AI can enhance your sales and customer engagement processes at itinai.com.