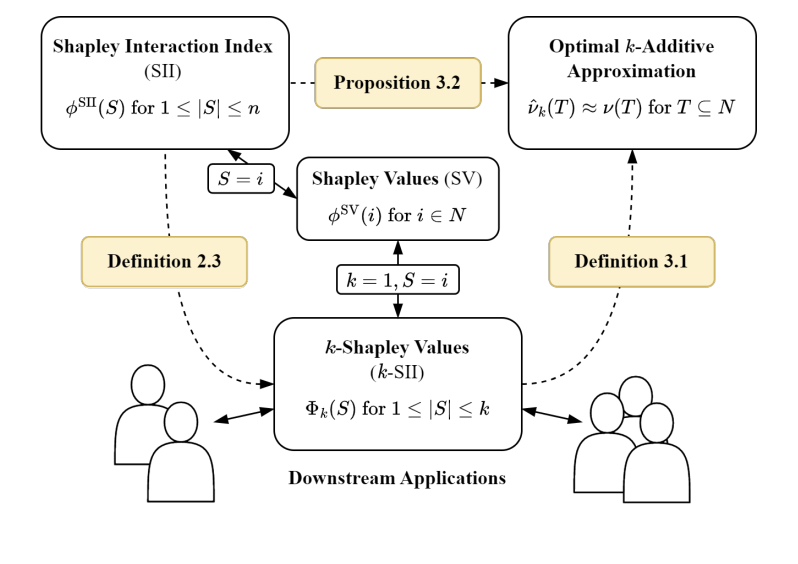
Machine Learning Interpretability: Understanding Complex Models
Machine learning interpretability is crucial for understanding complex models’ decision-making processes. Models are often seen as “black boxes,” making it difficult to discern how specific features influence their predictions. Techniques such as feature attribution and interaction indices enhance the transparency and trustworthiness of AI systems, enabling accurate interpretation of models for debugging and improving fairness and unbiased operation.
Challenges in Model Interpretability
A significant challenge is effectively allocating credit to various features within a model. Traditional methods like the Shapley value provide a robust framework for feature attribution but struggle to capture higher-order interactions among features. Higher-order interactions refer to the combined effect of multiple features on a model’s output, essential for a comprehensive understanding of complex systems.
Advancements in Model Interpretability: KernelSHAP-IQ
KernelSHAP-IQ is a novel method developed to address these challenges. It extends the capabilities of KernelSHAP to include higher-order Shapley Interaction Indices (SII) using a weighted least square (WLS) optimization approach. This advancement allows for a more detailed and precise framework for model interpretability, capturing complex feature interactions present in sophisticated models.
Method and Evaluation
KernelSHAP-IQ constructs an optimal approximation of the Shapley Interaction Index using iterative k-additive approximations, incrementally including higher-order interactions. The approach was tested on various datasets and model classes, demonstrating state-of-the-art results in capturing and accurately representing higher-order interactions.
Performance and Results
Empirical evaluations highlighted the method’s capability to consistently provide more accurate and interpretable results, enhancing the overall understanding of model dynamics. In experiments with the California Housing regression dataset, KernelSHAP-IQ significantly improved the estimation of interaction values, outperforming baseline methods substantially.
Impact and Future Applications
KernelSHAP-IQ’s advancements in model interpretability contribute significantly to the field of explainable AI, enabling better transparency and trust in machine learning systems. This research addresses a critical gap in model interpretability by effectively quantifying complex feature interactions, providing a more comprehensive understanding of model behavior.
Connect and Stay Informed
If you want to evolve your company with AI, stay competitive, and use AI for your advantage, check out the Paper. For AI KPI management advice and continuous insights into leveraging AI, connect with us at hello@itinai.com or follow us on Telegram or Twitter.
Practical AI Solutions for Your Business
Discover how AI can redefine your way of work. Identify Automation Opportunities, Define KPIs, Select an AI Solution, and Implement Gradually. For practical AI solutions, consider the AI Sales Bot from itinai.com/aisalesbot, designed to automate customer engagement 24/7 and manage interactions across all customer journey stages.