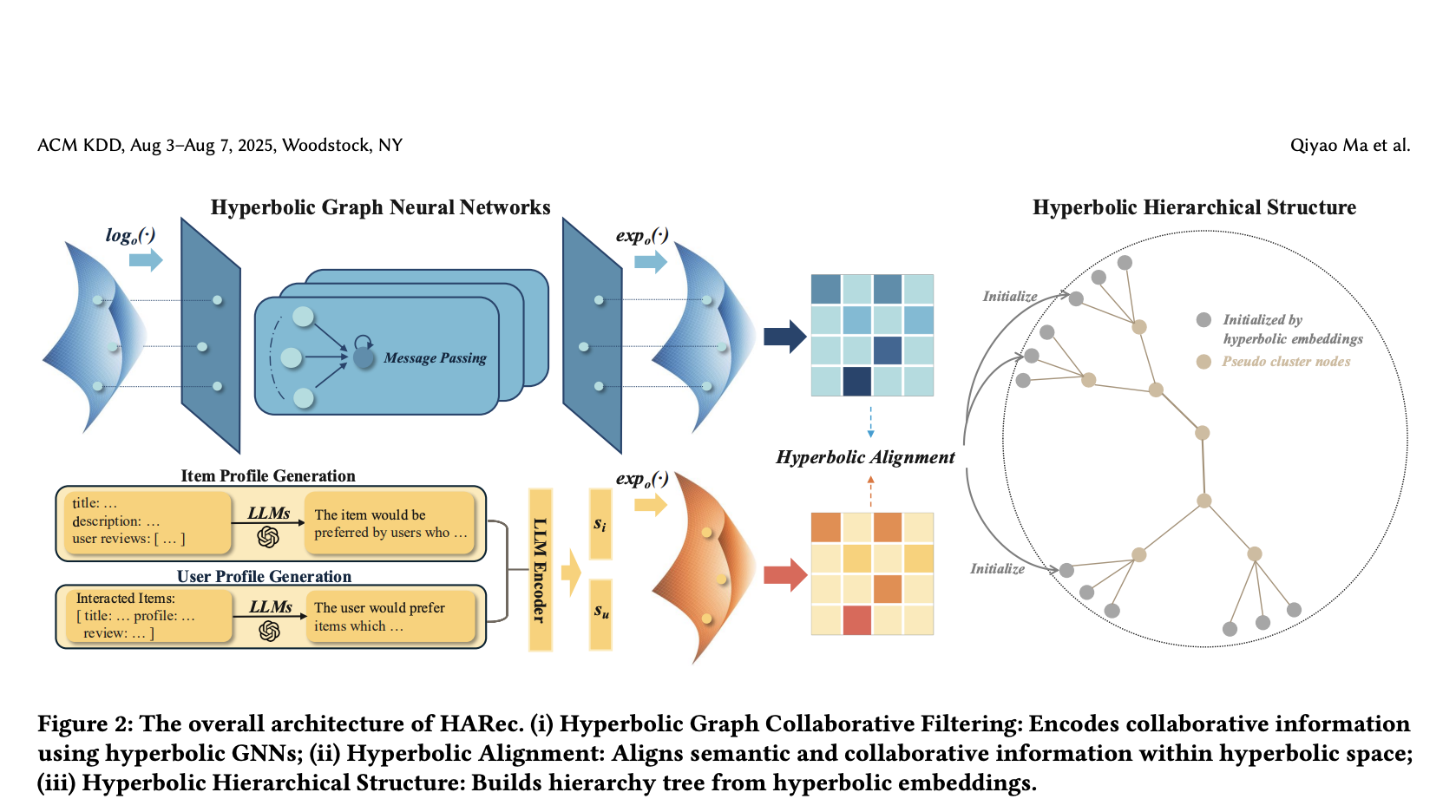
Introduction to Recommender Systems
Recommender systems play a crucial role in our digital experience. They tailor content for users by predicting what they might like based on their interactions. This personalization helps users deal with the overwhelming amount of information online by suggesting relevant items.
Challenges in Recommendation Systems
One major issue is the creation of information cocoons, where users only see similar content, limiting their exploration of new options. It’s essential to find the right balance between showing familiar and new items. This challenge requires advanced models capable of understanding complex user-item relationships and the meanings behind textual data.
Current Approaches and Their Limitations
Existing methods, like collaborative filtering and hyperbolic geometric models, have their strengths but also limitations. Collaborative filtering relies on past user interactions but struggles with the integration of meaningful text data. Hyperbolic models capture hierarchical relationships well but have difficulty aligning these with semantic insights.
Introducing HARec
Researchers from Snap Inc., Yale University, and the University of Hong Kong have developed HARec, an innovative learning framework that combines hyperbolic geometry with graph neural networks and large language models. HARec allows users to customize their experience, balancing exploration and exploitation in recommendations.
How HARec Works
HARec creates hyperbolic embeddings for user-item interactions and aligns them with semantic data using pre-trained models like BERT. This integration ensures a seamless understanding of both semantic and hierarchical relationships. The system organizes preferences into a hierarchical tree structure, making it easy for users to navigate their interests.
User Control and Flexibility
HARec’s unique feature is its user-adjustable parameters, allowing individuals to influence the mix of familiar and novel content in their recommendations. This flexibility empowers users and enhances their overall experience.
Proven Effectiveness
Extensive experiments on datasets such as Amazon books and Yelp showed HARec’s superiority in accuracy and diversity. It achieved significant improvements in recall and ranking metrics, proving its capability to provide relevant and diverse recommendations.
Addressing the Cold-Start Problem
HARec excels in dealing with cold-start problems, significantly boosting performance for items with limited interaction data. This adaptability showcases its ability to align semantic insights effectively.
Conclusion
HARec represents a significant step forward in recommendation systems, effectively balancing exploration and user preferences. Its innovative approach ensures a personalized and relevant experience for users, setting a new standard in digital content recommendations.
Stay Connected
Check out the Paper for more details. Follow us on Twitter, join our Telegram Channel, and be part of our LinkedIn Group. If you appreciate our insights, subscribe to our newsletter and join our 55k+ ML SubReddit.
AI for Your Business
If you’re looking to evolve your company with AI, consider these practical steps:
- Identify Automation Opportunities: Find customer interaction points that can benefit from AI.
- Define KPIs: Ensure your AI efforts have measurable impacts.
- Select an AI Solution: Choose tools that meet your needs and can be customized.
- Implement Gradually: Start with pilot projects, analyze results, and expand usage smartly.
For AI KPI management advice, contact us at hello@itinai.com. For ongoing insights into leveraging AI, stay connected on our Telegram or Twitter.