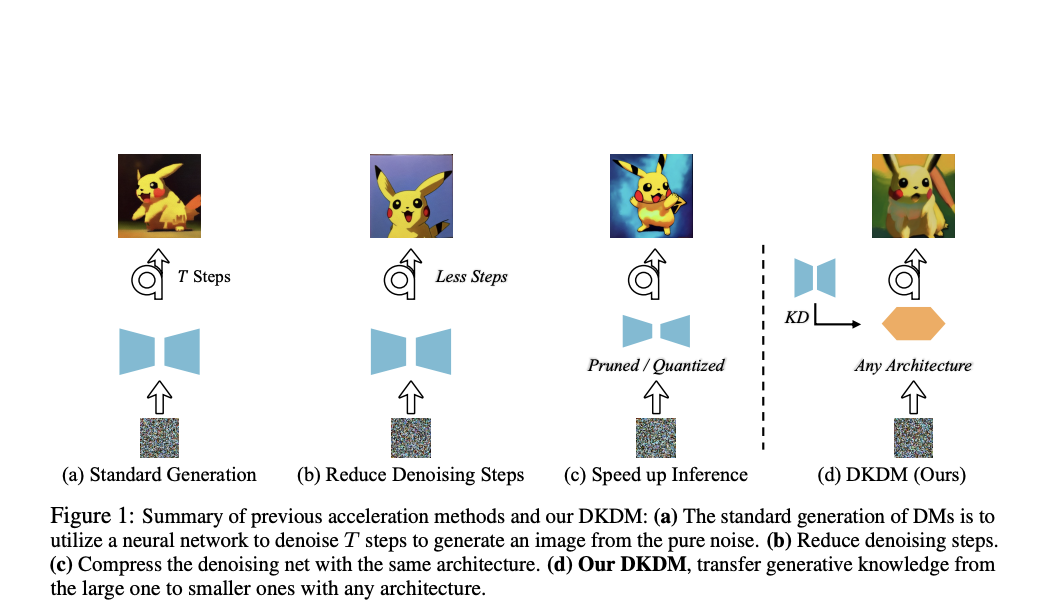
Practical Solutions for Diffusion Models
Challenges in Deploying Diffusion Models
Diffusion models, while powerful in generating high-quality images, videos, and audio, face challenges such as slow inference speeds and high computational costs, limiting their practical deployment.
Optimizing Diffusion Models
Methods like step reduction, quantization, and pruning are used to optimize diffusion models, but they often lead to trade-offs in model performance.
Data-Free Knowledge Distillation for Diffusion Models (DKDM)
DKDM introduces a novel method for distilling the capabilities of large diffusion models into smaller, more efficient architectures without relying on the original training data. This approach compresses diffusion models by transferring their knowledge to faster versions, addressing slow inference speeds while maintaining model accuracy.
Performance Improvements with DKDM
Experiments showed that DKDM-optimized student models achieved generation speeds twice as fast as baseline diffusion models while maintaining nearly the same level of performance. DKDM also integrates seamlessly with other acceleration techniques, such as quantization and pruning.
Value of DKDM
The DKDM method provides a practical and efficient solution to the problem of slow and resource-intensive diffusion models, offering significant potential for the future of generative modeling, particularly in areas with limited computational resources and data storage.
AI Solutions for Business
Identify Automation Opportunities, Define KPIs, Select an AI Solution, and Implement Gradually to evolve your company with AI and stay competitive.
Connect with Us
For AI KPI management advice and continuous insights into leveraging AI, connect with us at hello@itinai.com. Follow us on Telegram t.me/itinainews or Twitter @itinaicom.
Redefined Sales Processes and Customer Engagement
Discover how AI can redefine your sales processes and customer engagement. Explore solutions at itinai.com.