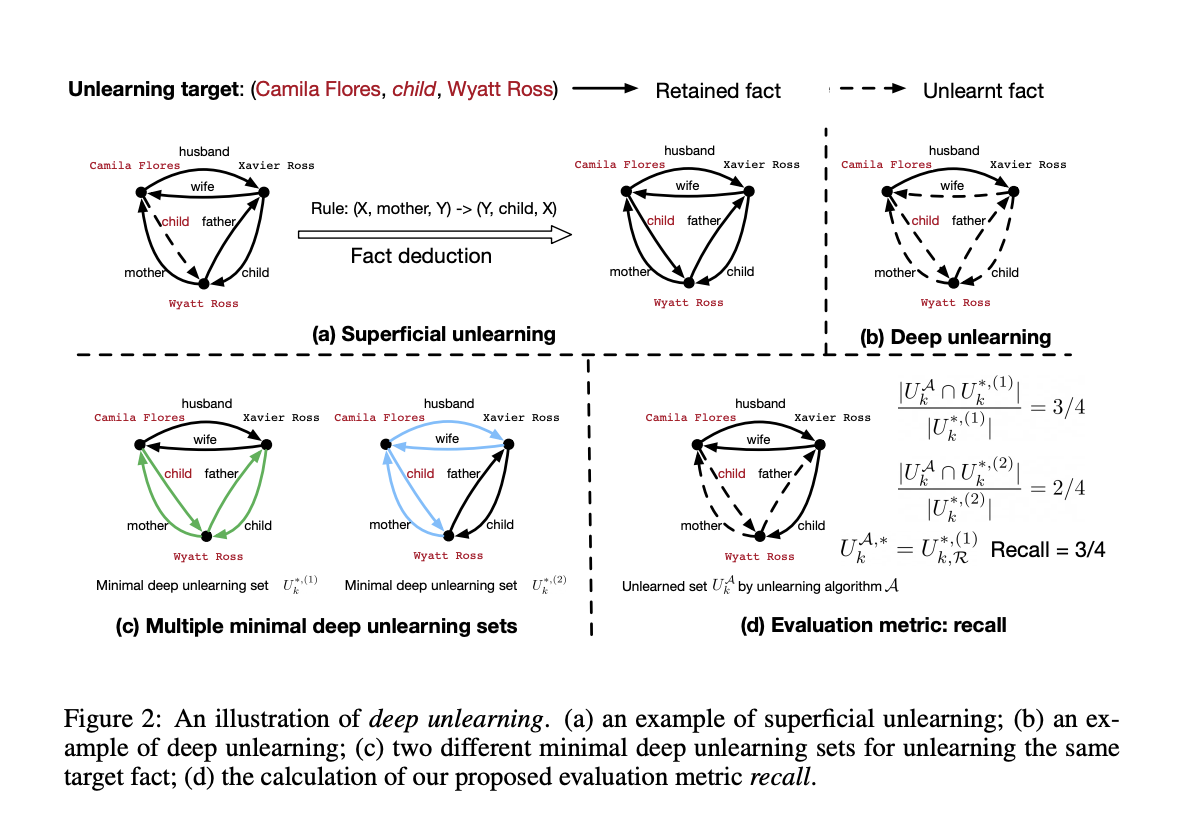
Understanding the Challenges of Large Language Models (LLMs)
Large language models (LLMs) are great at producing relevant text. However, they face a significant challenge with data privacy regulations, such as GDPR. This means they need to effectively remove specific information to protect privacy. Simply deleting data is not enough; the models must also eliminate any related information that could be inferred.
The Difficulty of Unlearning
Unlearning in LLMs is tough because all knowledge is interconnected. For example, if a family relationship fact is deleted, the model might still infer it from other related facts. This means we need unlearning methods that consider both the data itself and its connections.
Current Unlearning Techniques
Existing methods focus on removing specific data points using techniques like Gradient Ascent, Negative Preference Optimization (NPO), and Task Vector methods. While these approaches aim to delete data without losing model effectiveness, they often fail to achieve complete unlearning.
Introducing “Deep Unlearning”
Researchers from the University of California, San Diego and Carnegie Mellon University proposed the idea of deep unlearning. They created a dataset called EDU-RELAT, which consists of family relationships and logical rules to evaluate unlearning methods.
Testing Unlearning Techniques
In their study, researchers tested four unlearning methods: Gradient Ascent (GA), Negative Preference Optimization (NPO), Task Vector (TV), and Who’s Harry Potter (WHP) on four LLMs. The goal was to deeply unlearn 55 family relationship facts while maintaining model utility.
Results and Findings
The results showed that existing methods have significant room for improvement. For instance, Gradient Ascent had a 75% recall but often removed unrelated facts as collateral damage. Other methods like NPO and Task Vector achieved between 70%-73% recall on larger models. In contrast, WHP performed poorly, with recall below 50%.
Moreover, accuracy was generally higher for biographical facts compared to family relationships, highlighting the difficulty of unlearning closely related facts.
Moving Forward
This research reveals the limitations of the current unlearning approaches. While some methods show promise, they need to be more effective for deeply interconnected data. The study emphasizes the need for new methodologies that better address these challenges.
Unlocking AI Potential for Your Business
To stay competitive and harness the benefits of AI, consider the following practical solutions:
- Identify Automation Opportunities: Find key customer interaction points that can benefit from AI.
- Define KPIs: Ensure your AI initiatives have measurable impacts on business outcomes.
- Select an AI Solution: Choose tools tailored to your needs, ensuring customization is available.
- Implement Gradually: Start with a pilot project, gather data, and expand AI use gradually.
For AI KPI management advice, reach out to us at hello@itinai.com. Stay updated with continuous insights on our Telegram channel or on @itinaicom on Twitter.
Explore more about redefining your sales processes and customer engagement with AI at itinai.com.