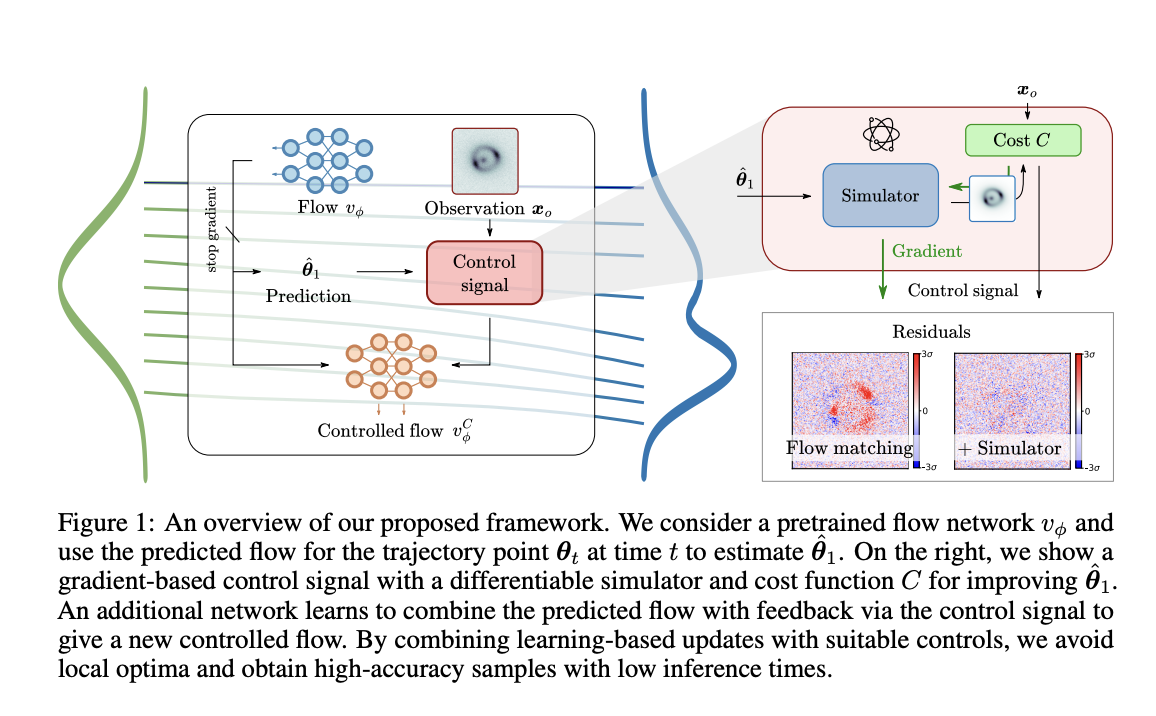
Flow-Based Generative Modeling: A Practical Approach
Flow-based generative modeling is a powerful method in computational science that helps make quick and accurate predictions from complex data. It’s especially useful in fields like astrophysics and particle physics, where understanding intricate data is crucial. Traditional methods can be slow and resource-intensive, creating a need for faster and more efficient solutions.
Challenges in Traditional Methods
One major issue with traditional inference methods, such as Markov Chain Monte Carlo (MCMC), is that they can take a long time to process high-dimensional datasets. This makes them unsuitable for applications that need real-time results. Additionally, existing models often struggle to adapt to new data, highlighting the need for improved techniques that maintain accuracy while reducing computational demands.
Innovative Solutions in Flow-Based Modeling
Flow-based generative modeling techniques like normalizing flows and diffusion models aim to convert simple data distributions into complex ones. However, they often lack real-time feedback mechanisms, limiting their effectiveness. Researchers are working on simulation-based inference (SBI) techniques to address these issues, but they still face challenges related to data size and model complexity.
Breakthrough Method from Technical University of Munich
A research team from the Technical University of Munich has developed a new method that integrates real-time feedback into flow-based generative modeling. By combining a pretrained flow network with a smaller control network, this approach allows for dynamic adjustments based on simulator feedback. This innovation enhances prediction accuracy without the need for extensive retraining.
How It Works
The method starts with a pretrained flow model that connects to a control network linked to a simulator. This setup enables real-time adjustments to sample trajectories using gradient-based signals, improving the flow network’s sampling process efficiently. The control network is compact, comprising only about 10% of the flow network’s weights, making it scalable for larger datasets.
Significant Performance Improvements
Tests on astrophysics applications showed that this new model significantly outperforms traditional methods. For example, it achieved a 53% improvement in sample accuracy and reduced inference time by up to 67 times compared to classical approaches. This model is particularly effective in tasks like galaxy-scale gravitational lensing, where accurate modeling is essential.
Broader Implications
This research highlights the potential of combining control-based feedback with flow-based generative models. It paves the way for advancements in accuracy without requiring large datasets or lengthy training. This method can bridge the gap between traditional inference and modern machine learning techniques, offering robust solutions for various scientific challenges.
For more insights, check out the Paper. All credit goes to the researchers involved. Follow us on Twitter, join our Telegram Channel, and connect with our LinkedIn Group. If you enjoy our work, subscribe to our newsletter and join our 55k+ ML SubReddit.
Sponsorship Opportunity
Promote your research, product, or webinar to over 1 million monthly readers and 500k+ community members.
Transform Your Company with AI
Stay competitive and leverage AI for your advantage:
- Identify Automation Opportunities: Find key customer interaction points that can benefit from AI.
- Define KPIs: Ensure measurable impacts on business outcomes.
- Select an AI Solution: Choose tools that fit your needs and offer customization.
- Implement Gradually: Start small, gather data, and expand AI usage wisely.
For AI KPI management advice, connect with us at hello@itinai.com. For continuous insights, follow us on Telegram or Twitter @itinaicom.
Discover how AI can enhance your sales processes and customer engagement at itinai.com.