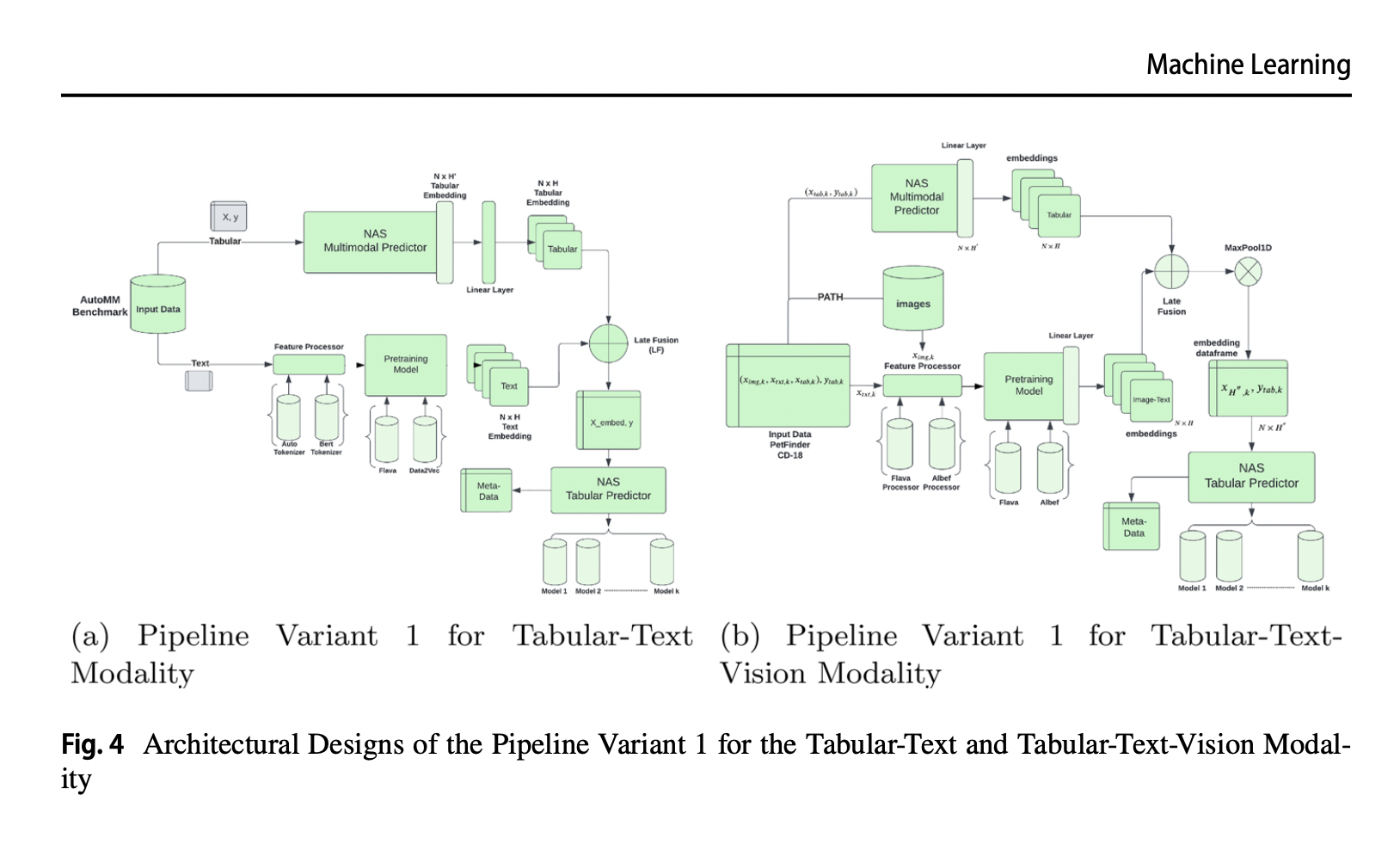
Introducing an Efficient AutoML Framework for Multimodal Machine Learning
Addressing Key Challenges in AutoML
Automated Machine Learning (AutoML) is crucial for data-driven decision-making, enabling domain experts to utilize machine learning without extensive statistical knowledge. However, a major obstacle is the efficient handling of multimodal data. Researchers from Eindhoven University of Technology have introduced a novel method leveraging pre-trained Transformer models to revolutionize AutoML.
Practical Solutions and Value
This innovative approach simplifies and guarantees the efficiency and adaptability of multimodal machine learning pipelines. By integrating pre-trained models and minimizing reliance on costly approaches, the framework offers practical solutions for dealing with complicated data modalities. It includes a flexible search space for multimodal data, strategic incorporation of pre-trained models, and warm-starting for Sequential Model-Based Optimization (SMBO).
Performance and Efficiency
The framework quickly converges to optimal configurations across different modalities, consistently producing high-quality pipeline designs while staying within computational limits. It outperforms classic methods in time-limited circumstances, highlighting the strengths of warm-starting and partial dependence on NAS.
Future Development
Future work will focus on improving the framework’s capabilities and expanding its application to different scenarios, such as parameter-space sampling, to keep up with the ever-changing needs of AutoML solutions.
Connect with Us
For AI KPI management advice and continuous insights into leveraging AI, connect with us at hello@itinai.com. Stay tuned on our Telegram channel or Twitter for more insights.