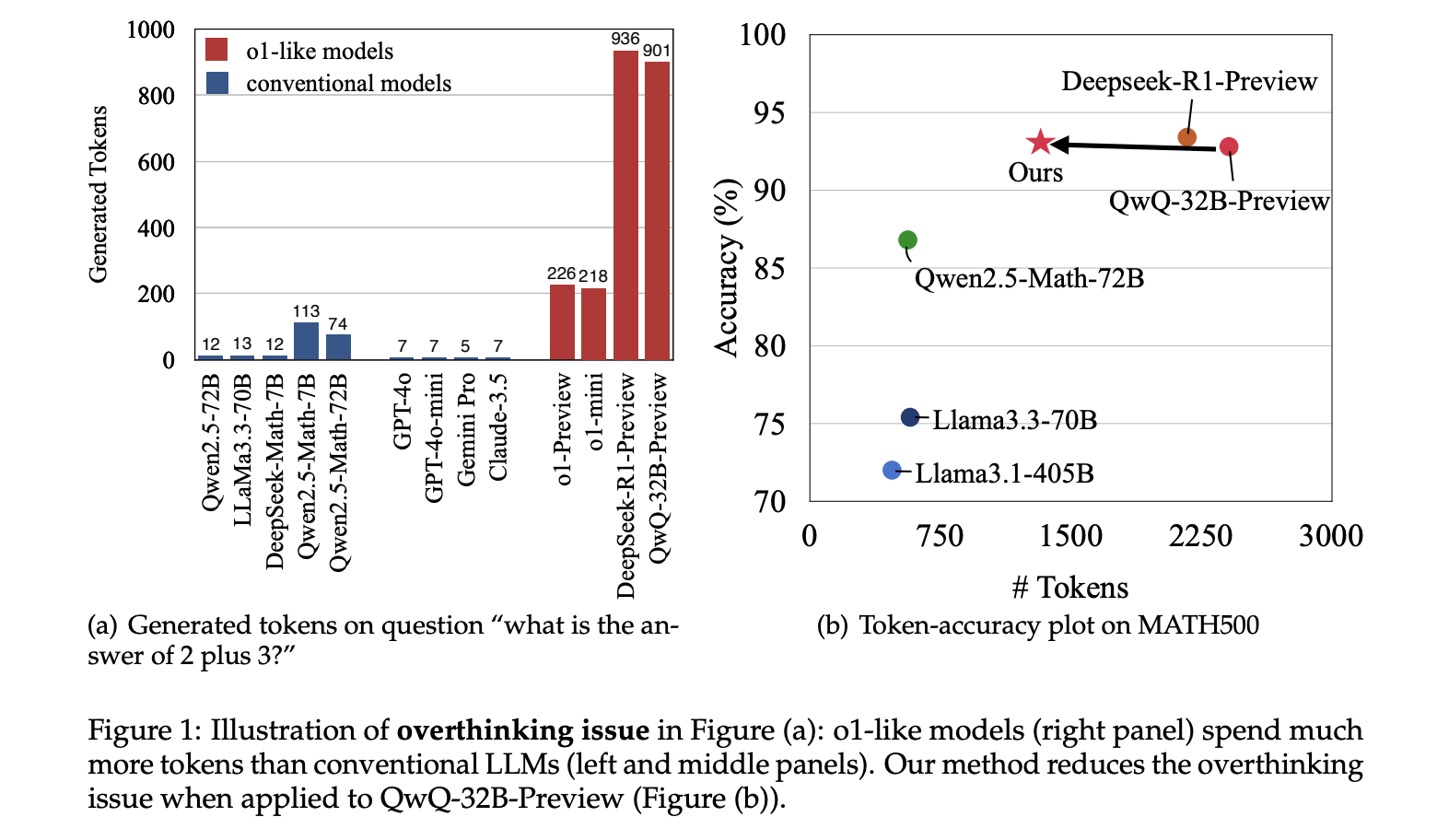
Understanding Large Language Models (LLMs)
Large language models (LLMs) are essential for solving complex problems. Models similar to OpenAI’s architecture show a strong ability to reason like humans. However, they often “overthink,” wasting resources on simple tasks, like solving “2 + 3,” which leads to higher costs and limits their use in resource-limited situations.
Research Insights
A recent study by Tencent AI Lab and Shanghai Jiao Tong University addresses the issue of overthinking in these models. The research reveals that excessive reasoning does not significantly improve accuracy. Experiments with datasets like GSM8K, MATH500, and AIME show that these models frequently provide unnecessary solutions for easy problems.
Practical Solutions and Benefits
The researchers introduce two new metrics: outcome efficiency and process efficiency. These metrics evaluate how well resources are used by considering both the accuracy of answers and the relevance of reasoning steps.
Self-Training Approach
To reduce overthinking, the team proposes a self-training method that incorporates these efficiency metrics. This approach focuses on prompt and accurate responses while maintaining thoughtful reasoning. Key strategies like First-Correct Solutions (FCS) and FCS+Reflection streamline computations and have shown to reduce token usage significantly—by 48.6% on the MATH500 dataset.
Results and Insights
The results are promising. The optimized methods led to a notable decrease in token usage on simpler tasks while improving accuracy. For instance, outcome efficiency improved from 52.3% to 75.8% with the FCS+Reflection strategy. The models also demonstrated less redundancy in reasoning across challenging datasets like GPQA and AIME, maintaining strong performance while lowering computational needs.
Conclusion
This study sheds light on the challenge of overthinking in o1-like models and presents effective solutions for efficient resource use. By introducing new evaluation metrics and training methods, the researchers show how to balance computational demands with model performance. These findings are vital for making advanced reasoning models more scalable and practical for various applications.
Stay Connected
Explore the full paper for more insights. Follow us on Twitter, join our Telegram Channel, and become part of our LinkedIn Group. Also, join our 60k+ ML SubReddit.
Join Our Webinar
Participate in our webinar to learn actionable strategies for enhancing LLM model performance while ensuring data privacy.
Transform Your Business with AI
Stay competitive by leveraging AI. Here’s how:
- Identify Automation Opportunities: Find customer interaction points that could benefit from AI.
- Define KPIs: Ensure measurable impacts from your AI initiatives.
- Select an AI Solution: Choose tools that fit your needs and allow customization.
- Implement Gradually: Start small, collect data, and expand usage wisely.
For AI KPI management advice, contact us at hello@itinai.com. For ongoing insights, follow us on Telegram or Twitter.
Revolutionize Your Sales and Customer Engagement
Explore how AI can transform your business processes at itinai.com.