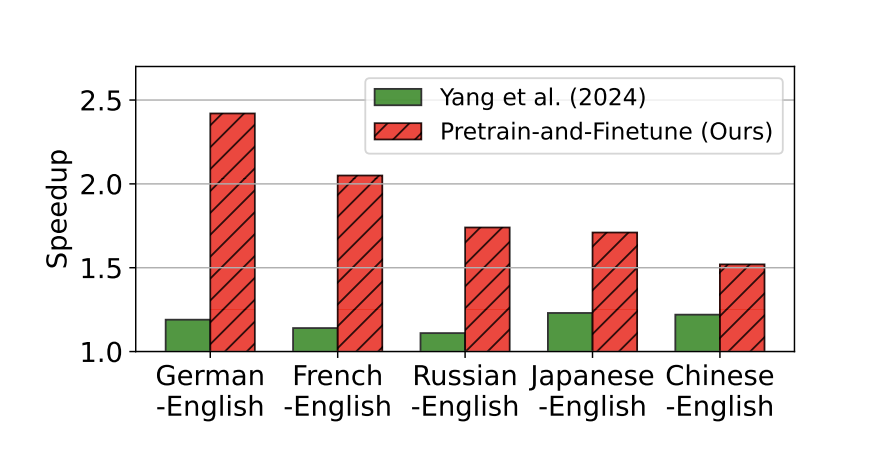
<>
Practical Solutions for Multilingual AI Efficiency
Challenges in Multilingual AI Deployment
Natural language processing (NLP) faces challenges in deploying large language models (LLMs) across multiple languages due to high computational demands.
Improving Multilingual Inference Efficiency
Researchers have introduced innovative methods like knowledge distillation and speculative decoding to optimize LLM efficiency in diverse language settings.
Specialized Drafter Models
A pre-train-and-finetune strategy enhances the alignment between drafter and primary LLM, significantly reducing inference time and improving accuracy in multilingual contexts.
Benefits of the Approach
The methodology results in faster response times, reduced computational costs, and enhanced performance in translation tasks across languages like German, French, Japanese, Chinese, and Russian.
AI Implementation Guidance
Implementing AI solutions involves identifying automation opportunities, defining KPIs, selecting suitable tools, and gradually integrating AI into workflows for optimal results.
Contact Us for AI Solutions
For AI KPI management advice and insights into leveraging AI for sales processes and customer engagement, connect with us at hello@itinai.com or follow us on Telegram and Twitter.
>