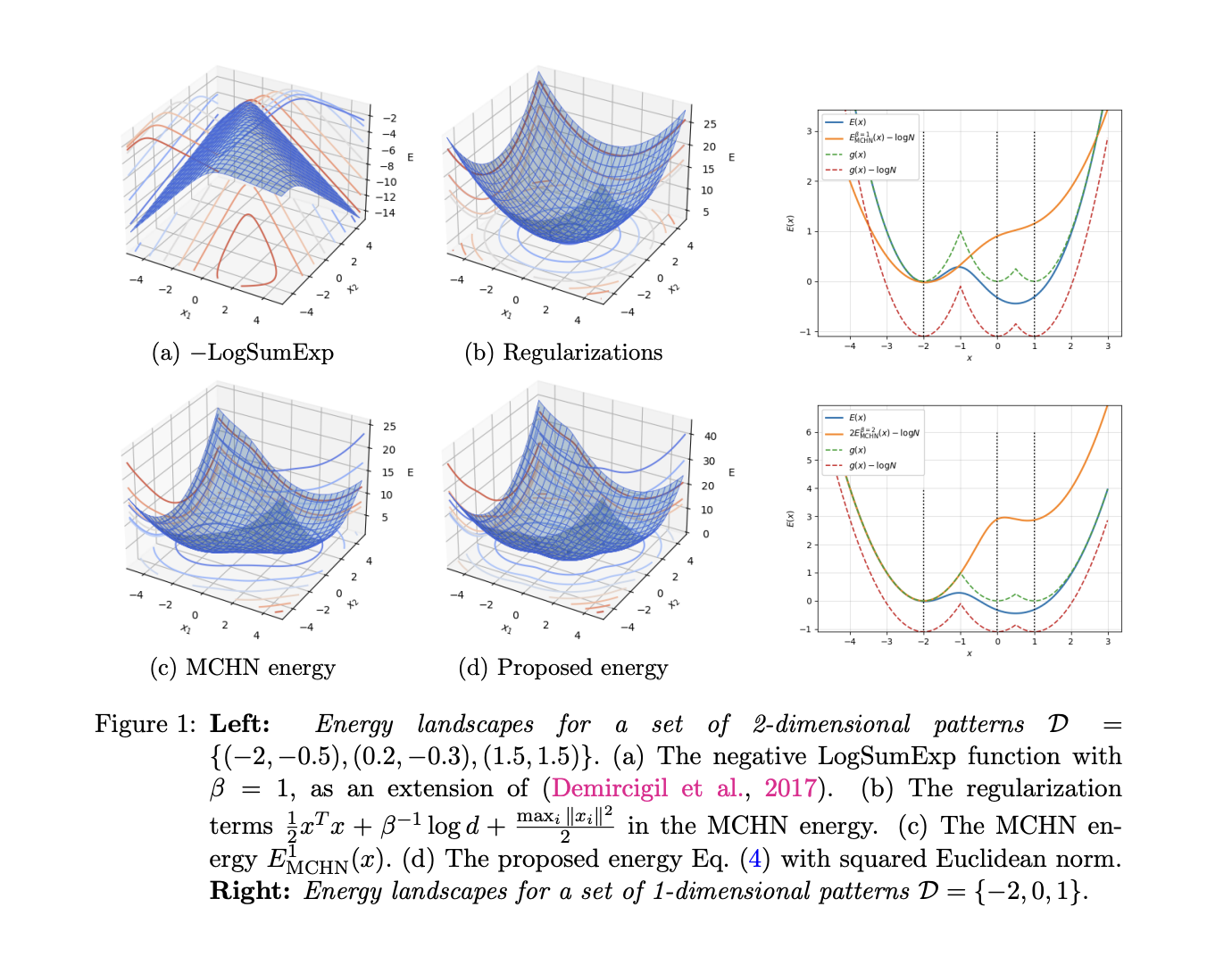
Transformer-based Neural Networks and Practical Solutions
Enhancing Performance and Overcoming Shortcomings
Transformer-based neural networks have demonstrated the ability to handle various tasks such as text generation, editing, and question-answering. Larger models often show better performance, but they can also lead to challenges. Practical solutions to overcome these shortcomings include scaling laws, energy-based models, and Hopfield models.
Research and Experiments
Researchers from Central Research Institute, 2012 Laboratories Huawei Technologies Co., Ltd. introduced a theoretical framework focused on the memorization process and performance dynamics of transformer-based language models. They conducted experiments using GPT-2 and vanilla Transformer models to validate theoretical insights and optimize decision-making in model training.
Training and Results
A 12-layer transformer LM was trained using the GPT-2 small tokenizer and architecture on the OpenWebText dataset. Training with different amounts of data showed insights into over-fitting and model energy dynamics. The experiments provided important theoretical insights and practical implications for model training.
Practical AI Solutions for Your Company
Evolve with AI and Stay Competitive
Discover how AI can redefine your way of work and help your company stay competitive. Identify automation opportunities, define KPIs, select an AI solution, and implement gradually to leverage the benefits of AI for your business.
AI KPI Management and Sales Automation
Connect with us for AI KPI management advice and continuous insights into leveraging AI. Explore practical AI solutions such as the AI Sales Bot designed to automate customer engagement 24/7 and manage interactions across all customer journey stages.