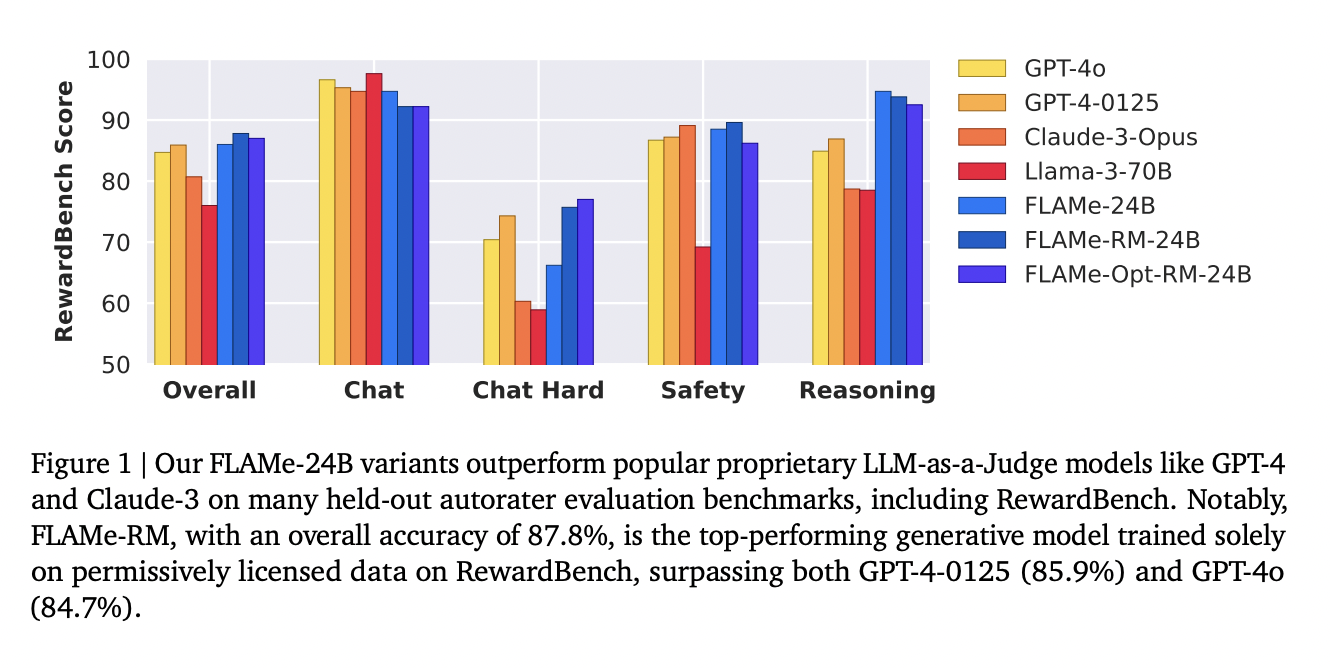
Evaluating Large Language Models (LLMs)
Challenges and Solutions
Evaluating large language models (LLMs) has become increasingly challenging due to their complexity and versatility. Ensuring the reliability and quality of these models’ outputs is crucial for advancing AI technologies and applications. Researchers need help developing reliable evaluation methods to assess the accuracy and impartiality of LLMs’ outputs, given human evaluations’ subjective, inconsistent, and costly nature.
Introducing FLAMe
A research team from Google DeepMind, Google, and UMass Amherst have introduced FLAMe, a family of Foundational Large Autorater Models designed to improve the evaluation of LLMs. FLAMe leverages a large and diverse collection of quality assessment tasks derived from human judgments to train and standardize autoraters. FLAMe is trained using supervised multitask fine-tuning on over 100 quality assessment tasks, encompassing more than 5 million human judgments. This training employs a text-to-text format, facilitating effective transfer learning across functions. The approach enables FLAMe to generalize to new tasks, outperforming existing models like GPT-4 and Claude-3.
Performance and Applicability
The performance of FLAMe is noteworthy across various benchmarks. The FLAMe-RM-24B model, a variant fine-tuned for reward modeling evaluation, achieved an accuracy of 87.8% on RewardBench, surpassing both GPT-4-0125 (85.9%) and GPT-4o (84.7%). On the CoBBLEr bias benchmark, FLAMe exhibits significantly lower bias compared to other autorater models. In addition to RewardBench, FLAMe’s performance is strong on other benchmarks. The FLAMe models outperform existing LLMs on 8 out of 12 automated evaluation benchmarks, covering 53 quality assessment tasks. This includes tasks such as summary comparisons, helpfulness evaluations, and factual accuracy assessments. The results demonstrate FLAMe’s broad applicability and robust performance across diverse evaluation scenarios.
Conclusion
To conclude, the research highlights the importance of reliable and efficient evaluation methods for LLMs. FLAMe offers a robust solution by leveraging standardized human evaluations, demonstrating significant improvements in performance and bias reduction. This advancement is poised to enhance the development and deployment of AI technologies. The FLAMe family of models, developed by a collaborative team from Google DeepMind, Google, and UMass Amherst, represents a significant step forward in evaluating large language models, ensuring their outputs are reliable, unbiased, and of high quality.