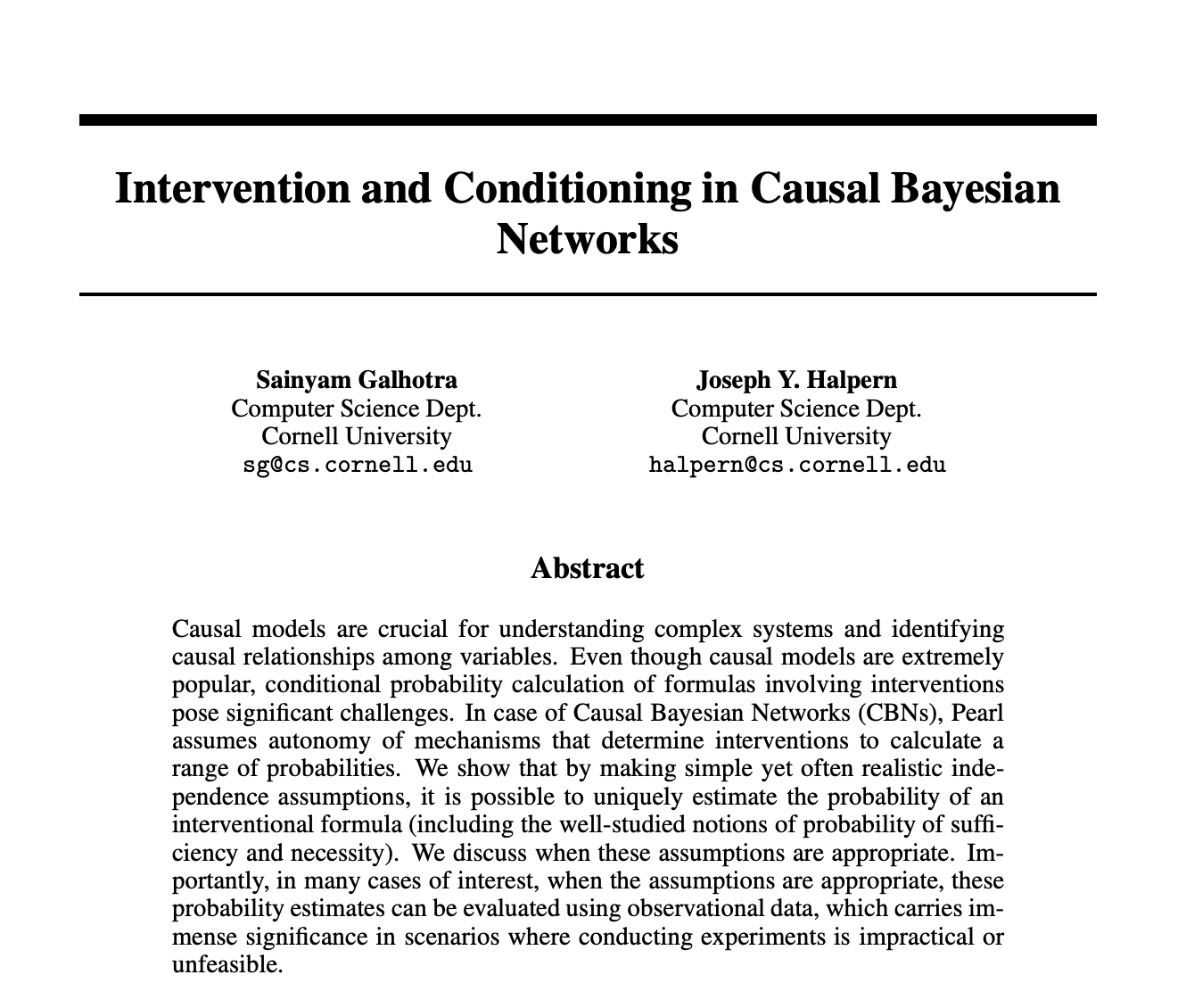
Practical Solutions and Value of Causal Models in AI
Understanding Causal Relationships
Causal models are essential for explaining how different factors interact and influence each other in complex systems. They help in understanding causal mechanisms and relationships among variables.
Applications in Various Fields
Causal models have practical applications in fields such as healthcare, epidemiology, and economics. They provide a formal representation of system variables and help in analyzing the impact of changes on market behavior and patient outcomes in AI-driven healthcare diagnostics.
Estimating Interventional Probabilities
Researchers have introduced a method to estimate the probability of an interventional formula by making real and independent assumptions. This method is valuable in cases where conducting experiments is impossible, and it helps in evaluating probabilities with observational data.
Functional Causal Models
In functional causal models, structured equations are used to represent the causal effect of variables. These models help in splitting variables into exogenous and endogenous sets, providing insights into the causal relationships among variables.
AI Solutions and Automation Opportunities
AI can redefine the way businesses work by identifying automation opportunities, defining measurable KPIs, selecting suitable AI tools, and implementing AI solutions gradually. This can lead to improved customer engagement and sales processes.
AI Sales Bot from itinai.com
Consider the AI Sales Bot from itinai.com/aisalesbot, designed to automate customer engagement 24/7 and manage interactions across all customer journey stages.