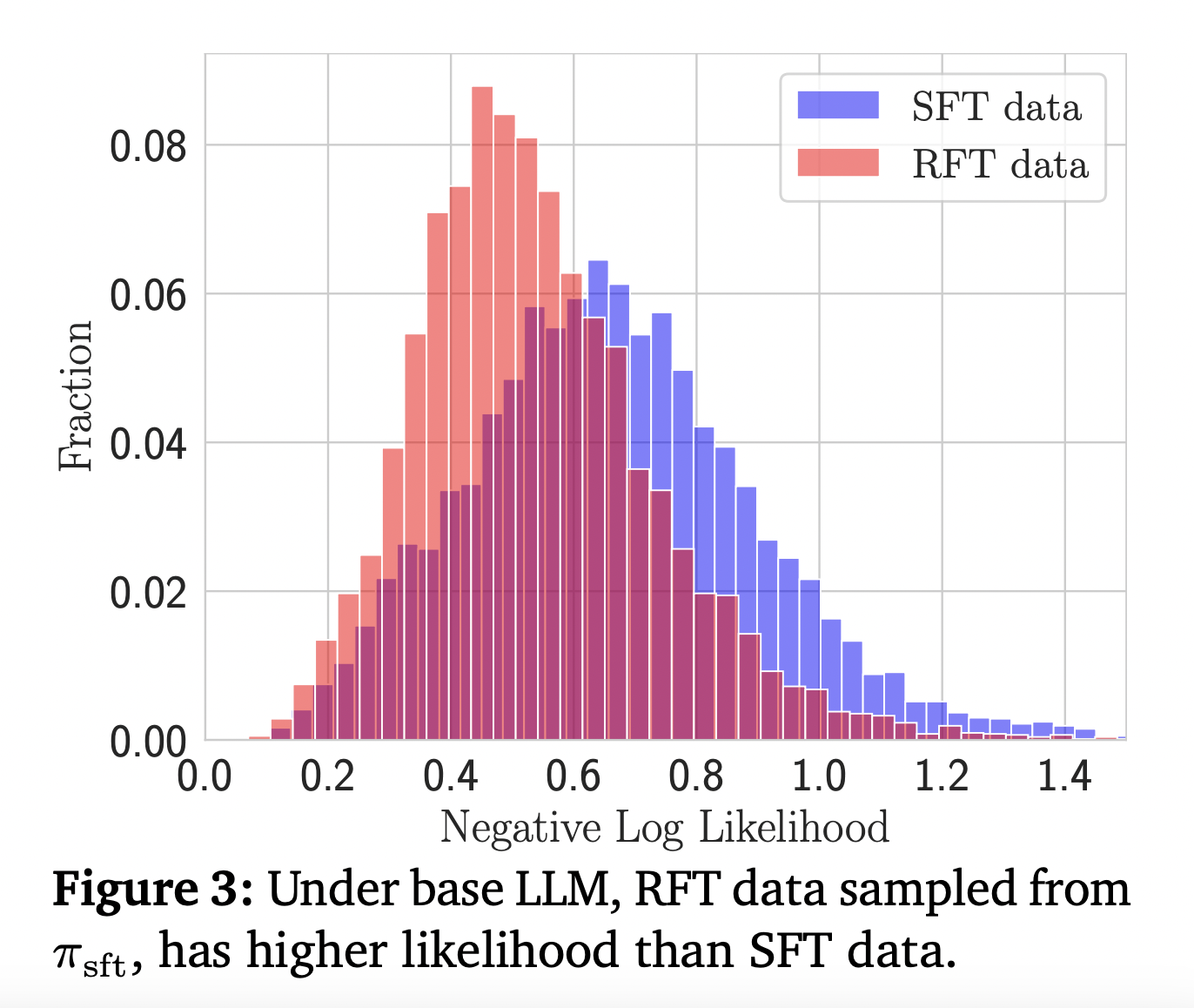
The Role of Synthetic Data in Improving LLMs’ Math Reasoning Capabilities
Research Findings:
Large language models (LLMs) face a challenge due to the scarcity of high-quality internet data. By 2026, researchers will need to rely on model-generated or synthetic data for training. This shift brings both opportunities and risks, impacting model performance and introducing biases. The challenge is to design high-quality synthetic data that addresses data scarcity without compromising model integrity.
Researchers have explored various approaches to tackle LLM training challenges using synthetic data. Efforts include generating positive synthetic data to mimic high-quality training data and using negative responses to unlearn problematic patterns in the training data.
A recent study by researchers from Carnegie Mellon University, Google DeepMind, and MultiOn reveals that positive synthetic data improves performance but with slower scaling rates than pretraining. Self-generated positive responses match the effectiveness of a larger amount of data, while incorporating negative synthetic data can scale efficiency up to eight times compared to using only positive data.
Proposed Method Architecture:
Synthetic Data Pipeline: Prompts capable models to generate new problems, obtains solution traces, and implements a binary reward function to verify correctness.
Dataset Construction: Creates positive synthetic dataset, generates positive and negative datasets using model-generated solutions.
Learning Algorithms: Includes Supervised Finetuning (SFT), Rejection Finetuning (RFT), and Preference Optimization using Direct Preference Optimization (DPO) with two variants: standard DPO and per-step DPO.
Conclusions and Recommendations:
The study emphasizes the importance of carefully constructing and utilizing both positive and negative synthetic data in LLM training for mathematical reasoning tasks. It suggests that incorporating negative (incorrect) traces can significantly enhance LLMs’ mathematical reasoning abilities.
AI Solutions for Business Transformation:
AI can redefine your way of work by identifying automation opportunities, defining measurable KPIs, selecting appropriate AI solutions, and implementing AI usage gradually.
For AI KPI management advice and insights into leveraging AI, connect on Telegram or Twitter.