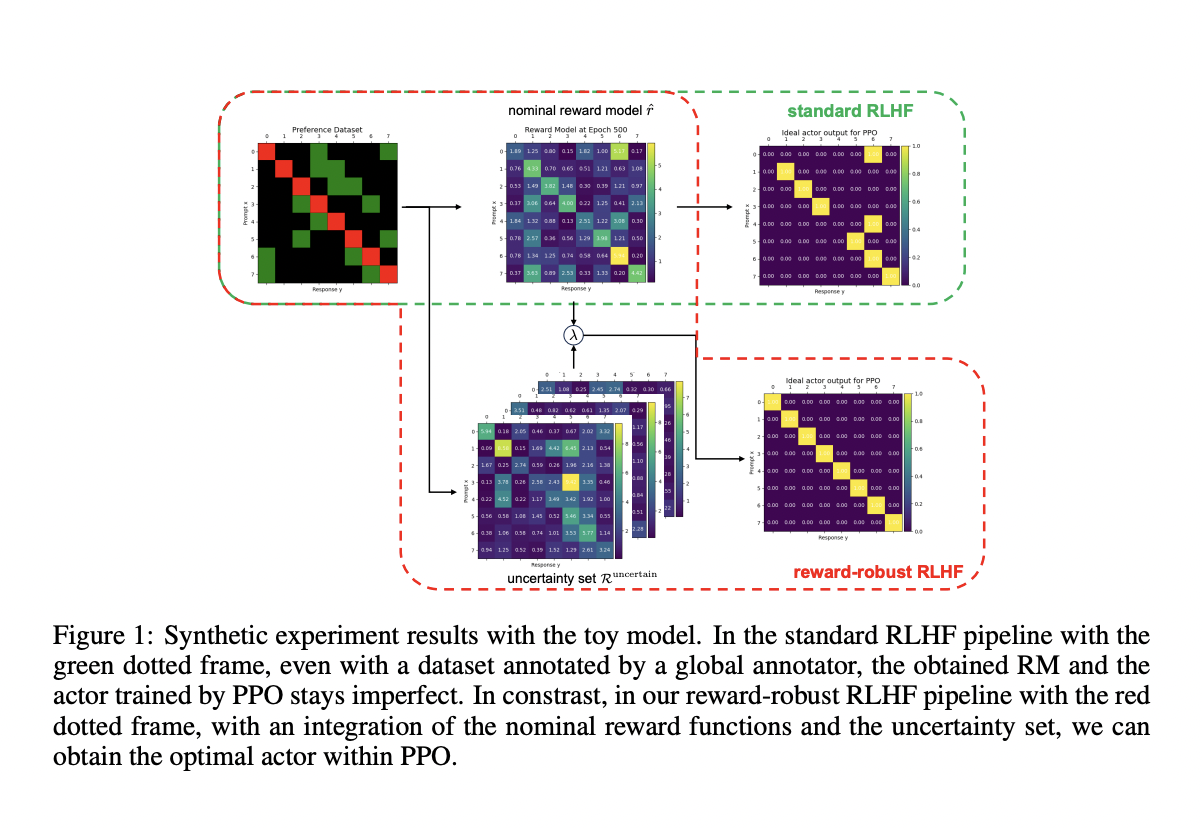
Practical Solutions and Value of Reward-Robust RLHF Framework
Enhancing AI Stability and Performance
Reinforcement Learning from Human Feedback (RLHF) aligns AI models with human values, ensuring trustworthy behavior.
RLHF improves AI systems by training them with feedback for more helpful and honest outputs.
Utilized in conversational agents and decision-support systems to integrate human preferences.
Challenges in RLHF
Instability and imperfections in reward models can lead to biases and misalignment with human intent.
Issues like reward hacking and overfitting hinder the performance and stability of RLHF.
Proposed BRME Framework
Bayesian Reward Model Ensembles (BRME) effectively manage uncertainty in reward signals.
BRME balances performance and robustness, selecting reliable reward signals for stable learning outcomes.
Performance and Results
The BRME framework outperforms traditional RLHF methods, showing significant accuracy improvements.
Performance gains of 2.42% and 2.03% for specific tasks demonstrate the framework’s effectiveness.
Practical Value
The framework resists performance degradation from unreliable reward signals, ensuring stability in real-world applications.
It offers a reliable solution to challenges like reward hacking and misalignment, advancing AI alignment.