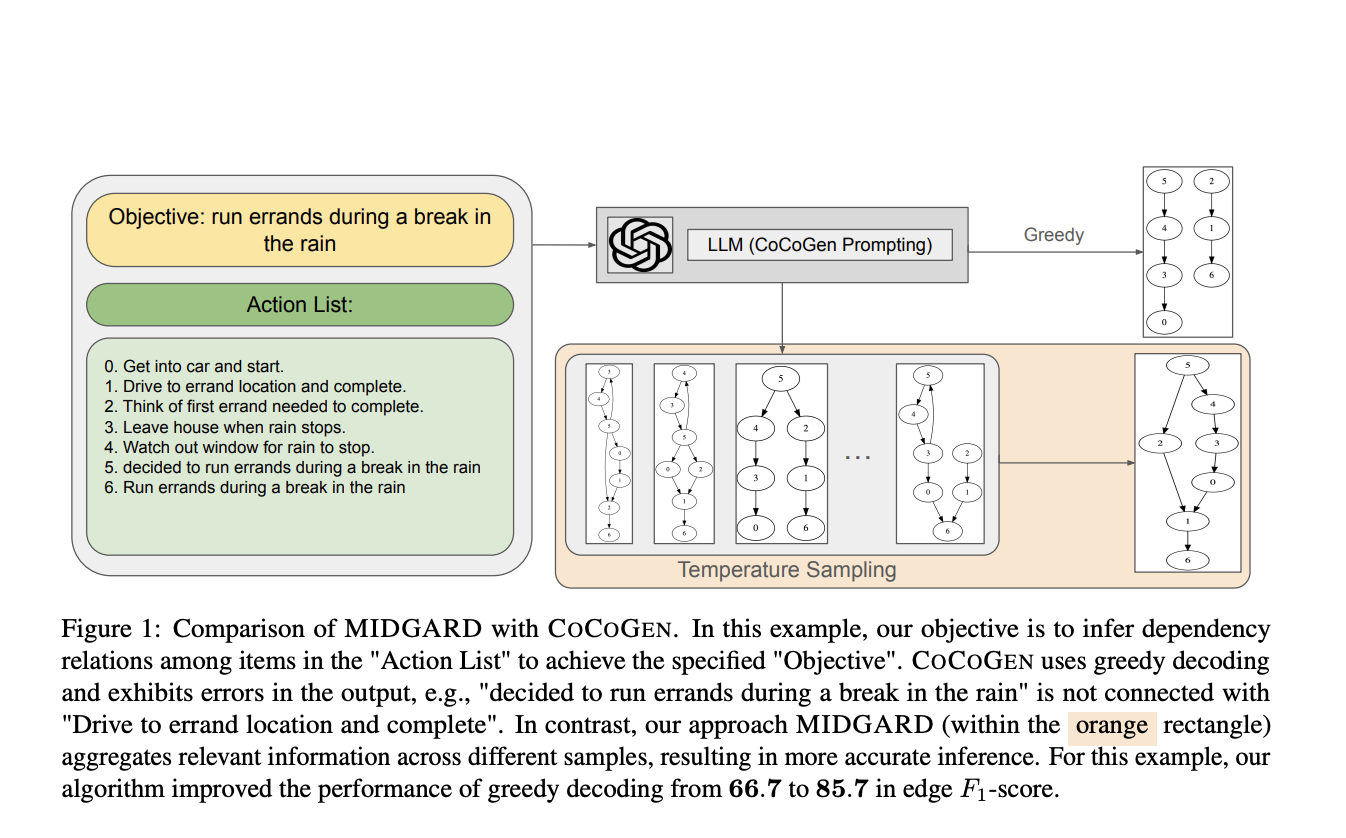
Structured Commonsense Reasoning in Natural Language Processing
Automated generating and manipulating reasoning graphs from textual inputs to enable machines to understand and reason about everyday situations as humans would.
Challenges and Solutions
Difficulty in accurately modeling and automating commonsense reasoning requires robust mechanisms for correcting inaccuracies during graph generation. Improving methods is critical to enhance the accuracy and reliability of automated reasoning systems.
Research and Innovations
Frameworks like COCOGEN and the self-consistency framework enhance model reliability by aggregating common results from multiple samples. MIDGARD utilizes the Minimum Description Length (MDL) principle to enhance structured commonsense reasoning, synthesizing multiple reasoning graphs to produce a more accurate and consistent composite graph.
Performance and Validation
MIDGARD demonstrated significant improvements in structured reasoning tasks, showcasing its advancement over traditional single-sample-based approaches in natural language processing. It increased the edge F1-score from 66.7% to 85.7% in the argument structure extraction task and consistently achieved higher accuracy in semantic graph generation.
AI Solutions for Business
Identify Automation Opportunities, Define KPIs, Select an AI Solution, and Implement Gradually to leverage AI for business impact. Connect with us at hello@itinai.com for AI KPI management advice and stay tuned on our Telegram t.me/itinainews or Twitter @itinaicom for continuous insights into leveraging AI.
Practical AI Solution
Consider the AI Sales Bot from itinai.com/aisalesbot designed to automate customer engagement 24/7 and manage interactions across all customer journey stages.