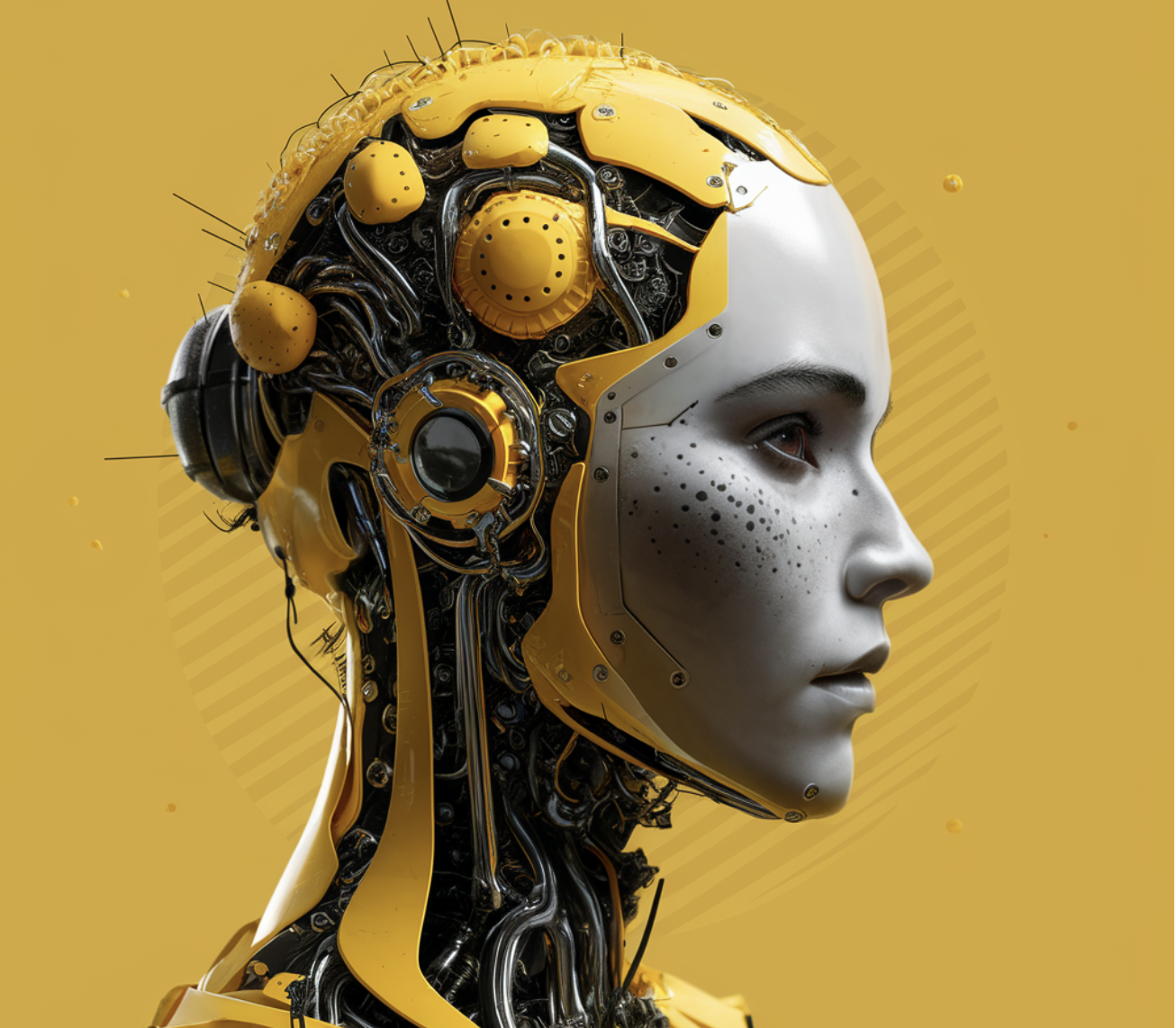
The Rise of Agentic Retrieval-Augmented Generation (RAG) in Artificial Intelligence AI
Retrieval-Augmented Generation (RAG)
RAG enhances Large Language Model (LLM) applications by using custom data to improve response generation, ensuring current information and enhancing user trust.
Agentic RAG
Expands on traditional RAG by adding autonomous agents that contribute intelligence and decision-making, enabling dynamic, context-aware AI with adaptability and learning.
Agentic RAG Architecture
Utilizes an intelligent orchestrator and specialized tools connected to various data sources, managing interactions and ensuring accurate and contextually relevant information synthesis.
Applications of Agentic RAG
Enhances customer service, conversational AI, content creation, education, healthcare, legal compliance, and more through intelligent, contextually appropriate responses.
Challenges
Addressing data curation, scalability, interpretability, security, privacy, and ethical considerations to ensure trustworthy and responsible AI usage.
Conclusion
Agentic RAG combines autonomous agents with classical RAG, offering intelligent and contextually relevant responses to complex queries, opening new avenues for business and transforming human interaction with information.
Spotlight on a Practical AI Solution
Consider the AI Sales Bot from itinai.com/aisalesbot designed to automate customer engagement 24/7 and manage interactions across all customer journey stages.