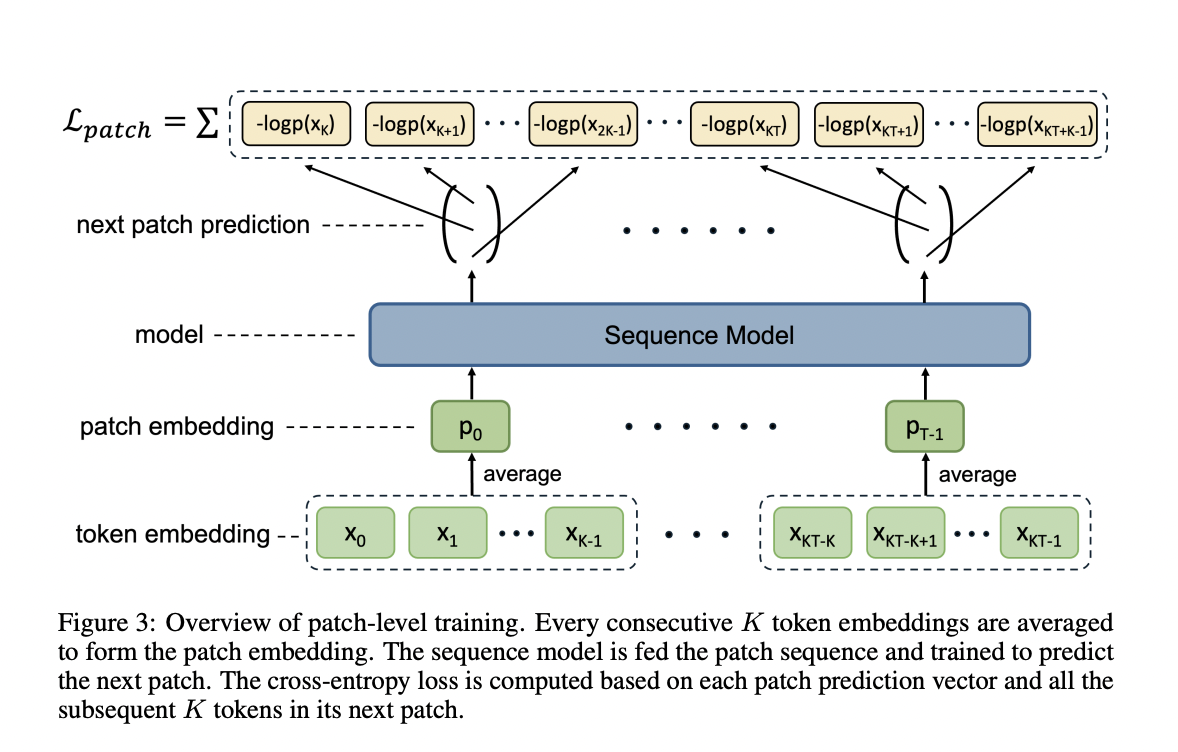
The Solution: Patch-Level Training for Large Language Models LLMs
Reducing Training Costs and Improving Efficiency without Compromising Model Performance
Overview
The proposed patch-level training method offers a potential solution to the challenge of large language model (LLM) training, promising to reduce training costs and improve efficiency without compromising model performance.
The Method
In this approach, researchers compress multiple tokens into a single patch to shorten the sequence, reducing training costs through transfer learning. The method involves training at both the patch and token levels, focusing on predicting groups of tokens at the patch level and individual tokens at the token level. This approach increases efficiency by forecasting all tokens in the upcoming patch at the same time.
Benefits
Experimental results show a 50% reduction in LLM training expenses while maintaining comparable performance. The adaptability of patch-level training makes it more applicable and less resource-intensive than model growth, holding potential for further improvements and scalability.
Next Steps
Further developments in determining an empirical scaling rule for patch-level training and testing its scalability on larger models and datasets could significantly improve this approach, offering even more benefits.
Adopting AI Solutions for Business
For businesses looking to leverage AI, it is essential to identify automation opportunities, define KPIs, select suitable AI solutions, and implement them gradually. This approach can redefine sales processes and customer engagement, offering measurable impacts on business outcomes.
For AI KPI management advice and continuous insights into leveraging AI, connect with us at hello@itinai.com or follow us on Telegram and Twitter.
Discover how AI can redefine your way of work and stay competitive with the latest AI solutions at itinai.com.