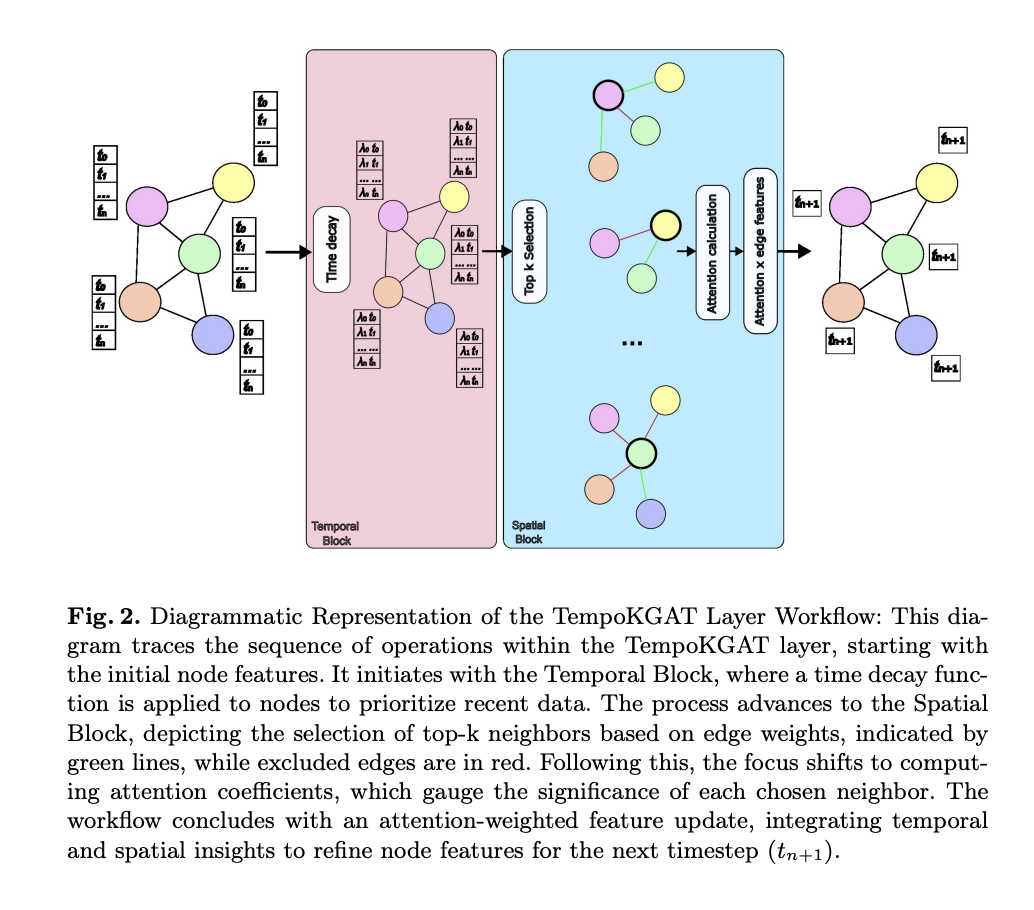
GNNs and Temporal Graph Analysis
Challenges and Practical Solutions
GNNs excel in analyzing structured data but face challenges with dynamic, temporal graphs. Traditional forecasting relied on statistical models for time-series data. Deep learning, particularly GNNs, shifted focus to non-Euclidean data like social and biological networks. However, applying GNNs to dynamic graphs needs improvement. Graph Attention Networks (GATs) partially address these challenges, but further advancements are needed, particularly in utilizing edge attributes.
Value of TempoKGAT
TempoKGAT, developed by researchers from Sorbonne University and TotalEnergies, integrates time-decaying weights and a selective neighbor aggregation mechanism to uncover latent patterns in spatio-temporal graph data. TempoKGAT consistently outperforms existing state-of-the-art methods across multiple metrics in traffic, energy, and health sectors, improving prediction accuracy and providing deeper insights into temporal graph analysis.
Model Enhancements
TempoKGAT enhances temporal graph analysis by refining node features through time-decaying weights and selective neighbor aggregation. The model prioritizes recent data, selects the most significant neighbors based on edge weights, and computes attention coefficients to dynamically integrate temporal and spatial insights, improving prediction accuracy and capturing evolving graph patterns.
Performance and Adaptability
TempoKGAT demonstrates exceptional performance across various datasets, particularly in PedalMe, ChickenPox, and England Covid, showcasing significant improvements in RMSE, MAE, and MSE metrics. The model’s adaptability is highlighted by its optimal neighborhood size parameter, enhancing prediction accuracy, and making it a robust and versatile tool for graph-based predictive analytics.
AI Solutions for Business
Practical Advice for AI Implementation
Evolve your company with AI and stay competitive by using TempoKGAT to redefine your way of work. Identify automation opportunities, define KPIs, select an AI solution, and implement gradually to ensure measurable impacts on business outcomes. For AI KPI management advice and continuous insights into leveraging AI, connect with us at hello@itinai.com or stay tuned on our Telegram t.me/itinainews or Twitter @itinaicom.
AI for Sales Processes and Customer Engagement
Discover how AI can redefine your sales processes and customer engagement. Explore solutions at itinai.com.