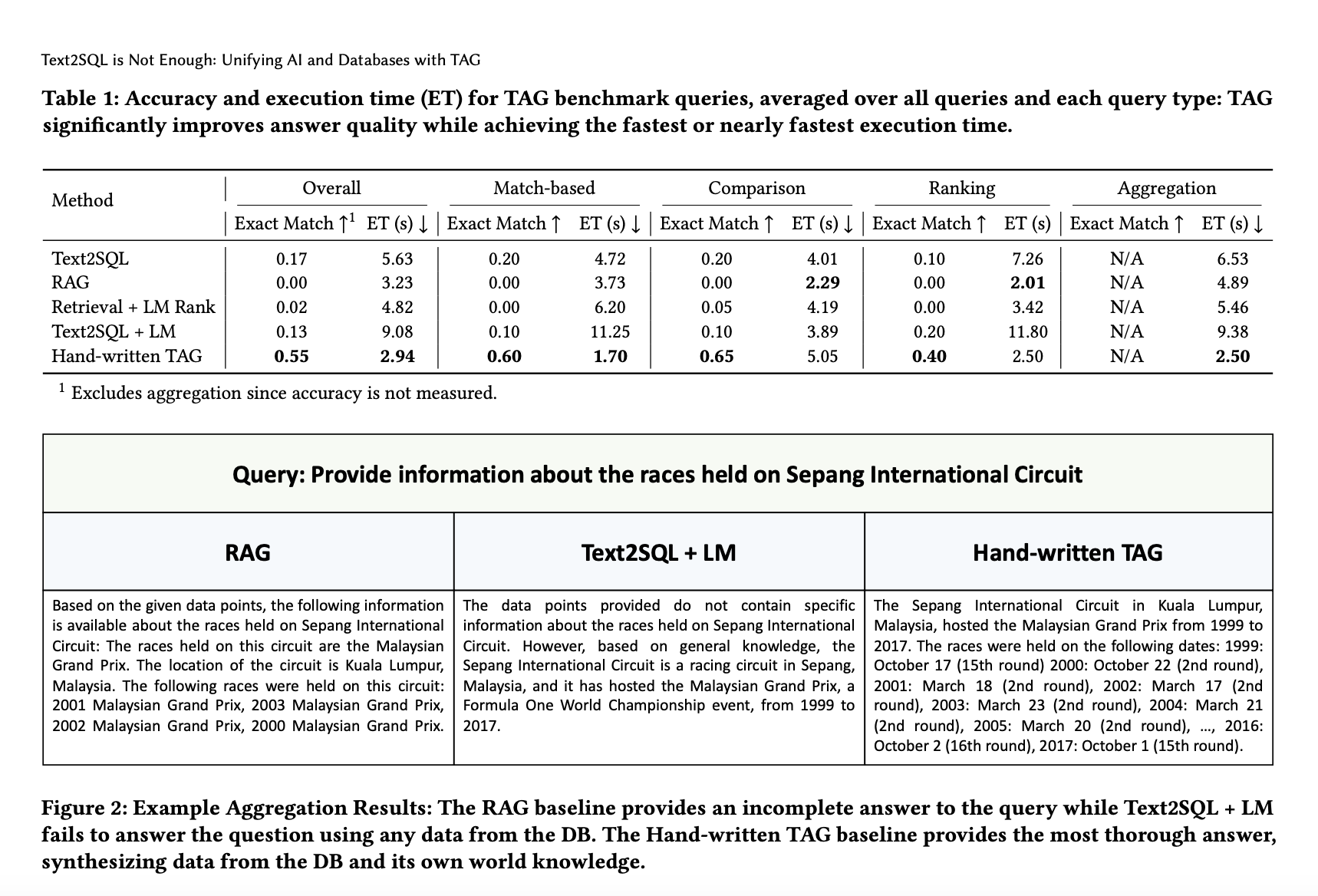
Unifying Language Models and Databases with Table-Augmented Generation (TAG)
Enhancing User Interaction with Large Datasets
Artificial intelligence (AI) and database management systems are converging to improve user interactions with large datasets. Recent advancements aim to enable natural language queries directly to databases for detailed, complex answers.
Challenges with Current Tools
Existing methods like Text2SQL and Retrieval-Augmented Generation (RAG) are limited in addressing real-world demands. Traditional AI models and databases need to be unified to enhance the scope and accuracy of responses to database-driven queries.
Introducing TAG: Table-Augmented Generation
Researchers have proposed TAG to combine the reasoning capabilities of language models with the computation power of databases. TAG transforms natural language queries into executable database queries, retrieves relevant data, and generates comprehensive responses.
Key Steps of TAG Model
The TAG model breaks down the question-answering process into three key steps: query synthesis, execution, and answer generation. It handles a wide variety of complex queries, demonstrating significant improvement over existing models.
Versatility and Performance
TAG outperforms Text2SQL and RAG and demonstrates versatility in processing queries across multiple domains. It achieves an average of 55% exact match accuracy and executes tasks up to 3.1 times faster than traditional methods.
Transforming Data-Driven Decision-Making
The unification of language models with databases through TAG opens up new possibilities for answering complex natural language queries. It addresses a key limitation of current models and has the potential to transform data-driven decision-making in various fields.
Evolve Your Company with TAG
Discover how TAG can redefine your way of work and evolve your sales processes and customer engagement. Connect with us to identify automation opportunities, define KPIs, select AI solutions, and implement AI for your business needs.