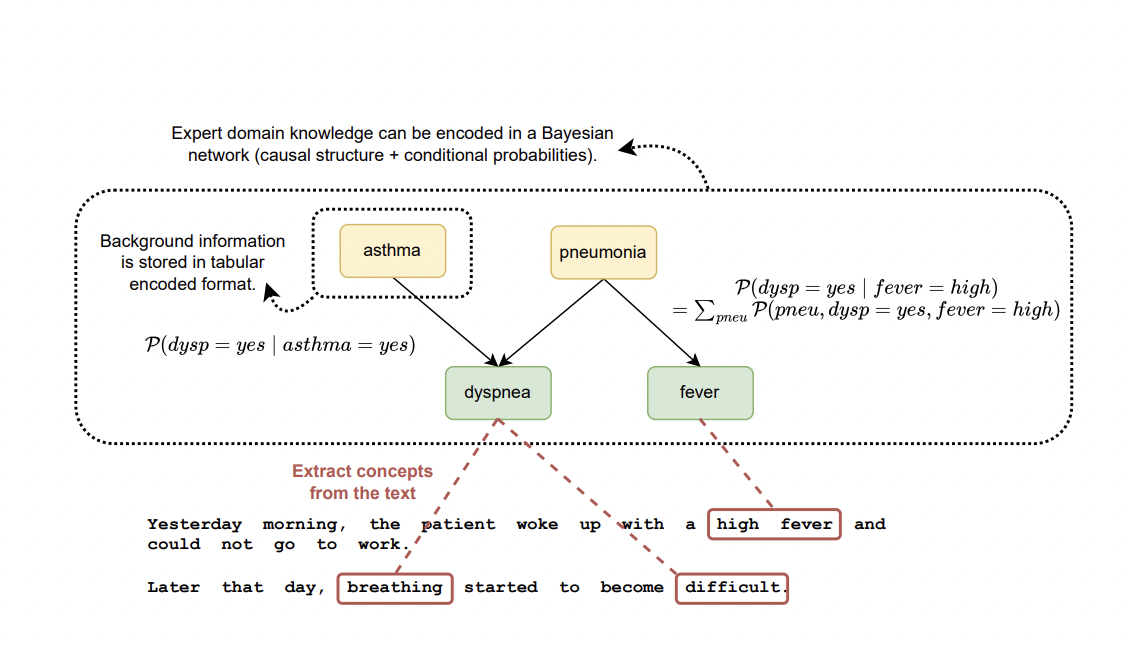
Practical Solutions and Value of SynSUM Dataset in Healthcare Research
Introduction
Electronic Health Records (EHRs) are rich in data, combining structured information with clinical notes. This forms the basis for training clinical decision support systems. However, challenges arise due to the interpretability of large language models and the limitations of feature-based models in processing unstructured text.
Value of SynSUM Dataset
The SynSUM dataset bridges the gap between structured and unstructured data in healthcare. It links clinical notes to background variables, aiding in clinical information extraction. This synthetic dataset offers valuable insights for research in clinical reasoning automation.
Key Approaches in SynSUM
The SynSUM method employs four distinct approaches to predict symptoms from clinical data, including Bayesian networks, XGBoost classifiers, and neural classifiers processing text and tabular variables.
Evaluation and Results
The methods were evaluated using an 8000/2000 train-test split and reported F1-scores for symptom prediction. Text-based methods outperformed tabular-only approaches, showing promising results in predicting symptoms like dyspnea and cough.
Applications and Future Work
SynSUM offers multiple applications in healthcare research by enhancing clinical information extraction techniques. Its unique structure combining structured and unstructured data makes it valuable for medical informatics and data science in healthcare settings.
Conclusion
The SynSUM dataset is a valuable resource for improving medical informatics and data science in healthcare. Its applications extend to various research areas, making it an essential tool for enhancing clinical decision-making processes.
For more details on the research, visit the original post on MarkTechPost.