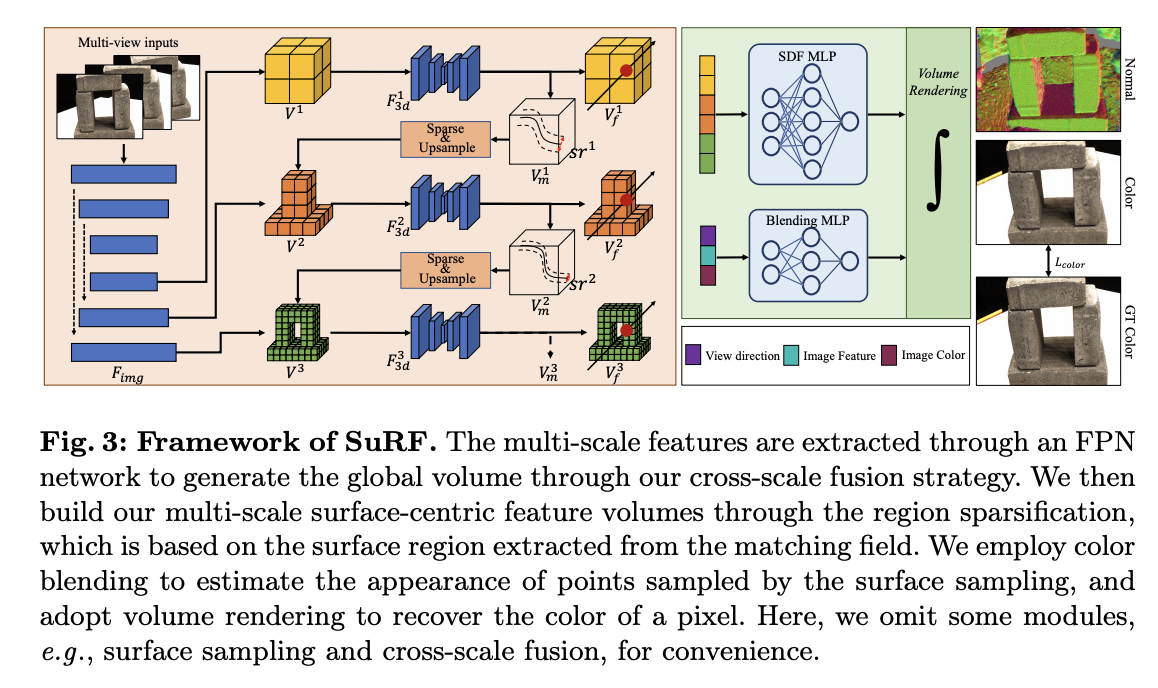
Practical AI Solutions for High-Fidelity 3D Reconstruction
Challenges in Surface Reconstruction
Reconstructing detailed 3D models from limited data is crucial in various fields like autonomous driving and robotics. However, this is difficult due to memory and computational constraints.
Existing Approaches
Current methods face limitations in accuracy and efficiency. Multi-stage pipelines accumulate errors, while end-to-end methods require a large number of input views, making them impractical for real-time applications.
Introducing SuRF
SuRF is a novel surface-centric framework designed to efficiently reconstruct detailed surfaces from sparse input views. It overcomes memory and computational limitations and offers accurate and efficient solutions for resource-constrained environments.
Key Components and Strategies
SuRF leverages the Matching Field module to efficiently locate surface regions and the Region Sparsification strategy to optimize computational resources. This approach significantly reduces memory and computational demands, offering scalability and efficiency in high-fidelity surface reconstruction.
Benefits and Results
SuRF demonstrates substantial improvements in accuracy and efficiency, outperforming existing approaches. It achieves a 46% improvement in accuracy while reducing memory consumption by 80% compared to previous methods.
Relevant AI Solutions for Business
For businesses looking to leverage AI solutions, SuRF offers a practical way to redefine work processes and customer engagement. It provides opportunities for automation, measurable impacts on business outcomes, customization, and gradual implementation.
Connect with AI Experts
For AI KPI management advice and insights into leveraging AI, connect with us at hello@itinai.com. Stay tuned for continuous updates on Telegram and Twitter.