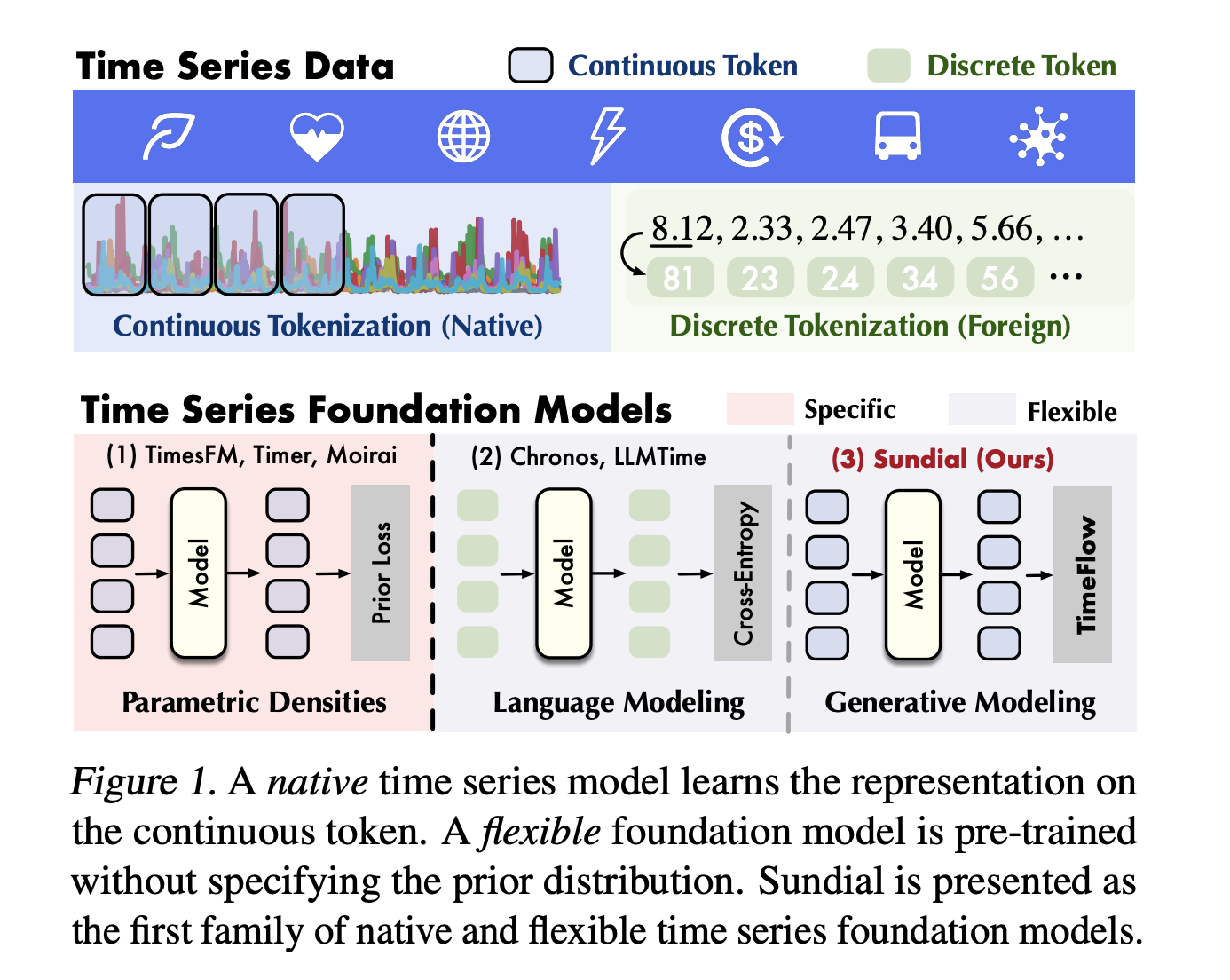
Understanding Time Series Forecasting Challenges
Time series forecasting is complex and unpredictable, making it hard to accurately predict future values. Traditional forecasting methods provide only a single value, which doesn’t reflect the range of possible outcomes. While deep learning has improved accuracy, these methods often need specific training and don’t work well across different data types. To build effective forecasting models, we must overcome these limitations.
Current Forecasting Models
Forecasting models fall into two main categories:
1. Statistical Models
These models, like ARIMA and Exponential Smoothing, are easy to understand but struggle with complex data.
2. Deep Learning Models
Transformer-based models show great predictive power but require extensive training and can struggle with data representation.
Introducing Sundial
Sundial offers a new solution for time series forecasting. It uses a generative model that learns directly from raw data, avoiding the limitations of traditional methods. Key features include:
- Continuous Tokenization: This keeps data intact and improves representation learning.
- TimeFlow Loss: This innovative training method allows for multiple future predictions, rather than just one.
- Large-Scale Training: Trained on a dataset with one trillion time points, Sundial is versatile across various forecasting tasks.
Key Innovations of Sundial
- Advanced Tokenization: Processes data in continuous segments for better learning.
- Enhanced Generalizability: A re-normalization method helps manage data variability.
- Transformer Architecture: Utilizes causal self-attention for improved handling of time dependencies.
- Efficient Training: Optimizations like Pre-LN and FlashAttention enhance stability and speed.
Performance and Benefits
Sundial has achieved top performance in various forecasting benchmarks, showing:
- Improved accuracy and efficiency in long-term forecasting.
- Superior results in probabilistic forecasting metrics.
- Scalability, with larger models yielding better outcomes.
- Flexible inference options for balancing speed and accuracy.
Transforming Industries with Sundial
Sundial is a breakthrough in time series forecasting, combining advanced modeling techniques to produce flexible and accurate predictions. Its ability to generate multiple future scenarios makes it a valuable tool for decision-making across industries.
Explore Further
Check out the Paper for more insights. Follow us on Twitter, join our Telegram Channel, and connect with our LinkedIn Group. Join our 75k+ ML SubReddit community!
Elevate Your Business with AI
Stay competitive by leveraging Sundial for time series forecasting:
- Identify Automation Opportunities: Find areas in customer interactions that can benefit from AI.
- Define KPIs: Ensure measurable impacts from AI initiatives.
- Select the Right AI Solution: Choose tools that fit your needs.
- Implement Gradually: Start small, collect data, and expand wisely.
For AI KPI management advice, connect with us at hello@itinai.com. For ongoing AI insights, follow us on Telegram or @itinaicom.
Discover how AI can enhance your sales processes and customer engagement at itinai.com.