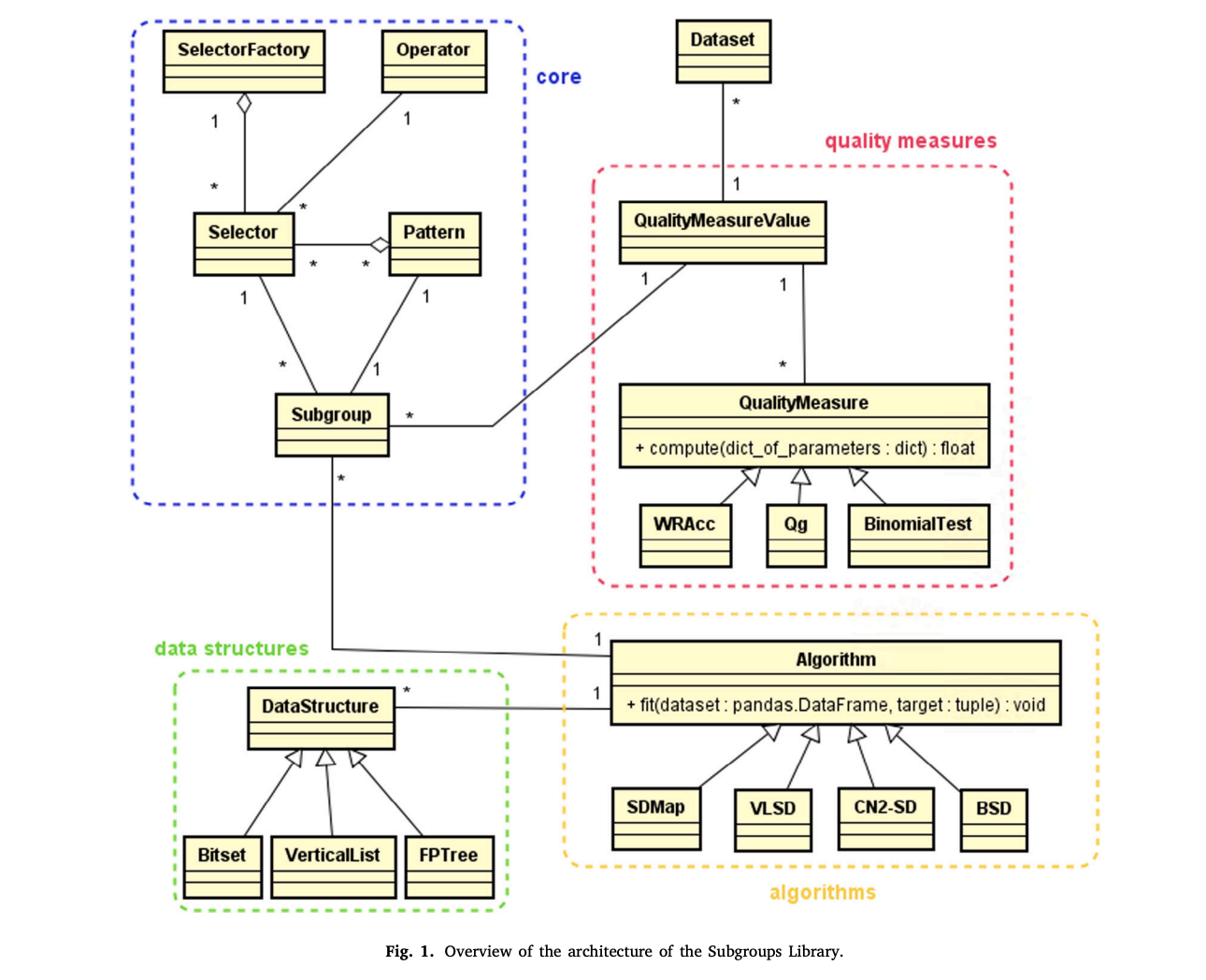
Practical Solutions and Value of Subgroups Library
Efficient Subgroup Discovery with Subgroups Library
Subgroups Library simplifies the use of Subgroup Discovery (SD) algorithms in machine learning and data science.
Key Features:
- Improved Efficiency: Native Python implementation for faster performance.
- User-Friendly Interface: Modeled after scikit-learn for easy accessibility.
- Reliable Algorithms: Based on established scientific research for trustworthiness.
Customization and Expansion
The library’s modular design allows for easy customization and expansion:
- Users can add new algorithms, quality measures, and data structures.
- Supports multiple SD algorithms and quality measures for diverse applications.
Practical Implementation and Impact
Subgroups Library has been applied in numerous research papers and real-world projects:
- Featured in scientific papers and downloaded over 7,100 times.
- Enables fair comparison of SD algorithms in a unified framework.
- Continuously evolving with potential for further expansion and new algorithm integration.
AI Integration Strategies
Discover how AI can transform your business:
- Identify Automation Opportunities.
- Define KPIs for measurable impacts.
- Select AI solutions aligned with your needs.
- Implement AI gradually for optimal results.
For AI KPI management advice, contact us at hello@itinai.com. Stay updated on leveraging AI via Telegram or Twitter.
Explore AI solutions for sales processes and customer engagement at itinai.com.