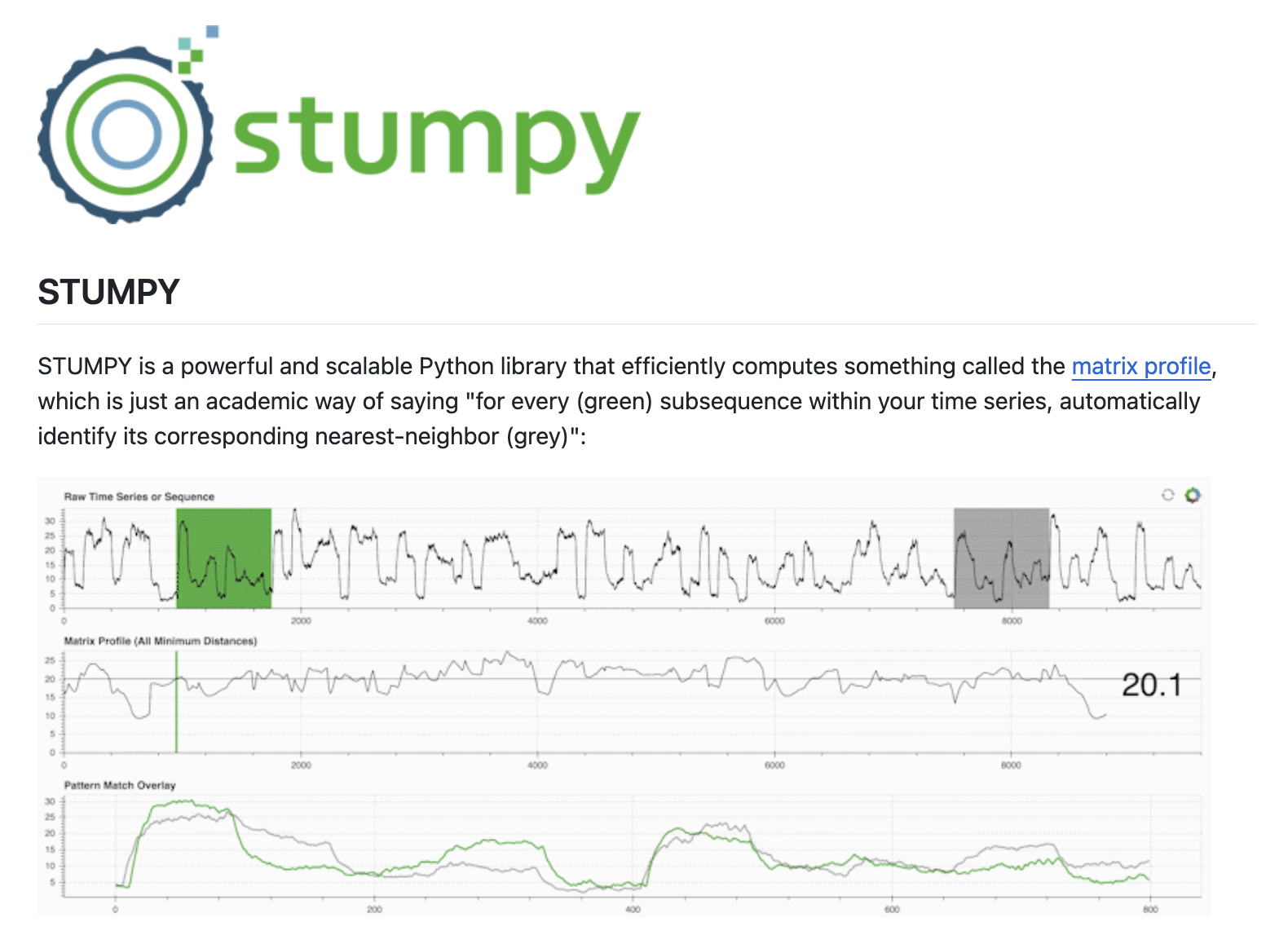
Stumpy: A Powerful and Scalable Python Library for Modern Time Series Analysis
Practical Solutions and Value
Time series data is utilized globally in finance, healthcare, and sensor networks. Identifying patterns and anomalies within this data is crucial for tasks like anomaly detection, pattern discovery, and time series classification, impacting decision-making and risk management. Time series analysis methods require high computational resources for understanding complex patterns in massive datasets. Traditional methods are constrained by high time complexity and sensitivity to noise.
Stumpy efficiently addresses the challenge of extracting meaningful patterns and anomalies from large time series datasets. It introduces a highly efficient method for time series analysis by computing matrix profiles. The matrix profile enables the quick identification of motifs, anomalies, and shapelets within time series data. Stumpy’s optimized algorithms, parallel processing, and early termination techniques offer a robust solution that significantly reduces computational overhead and enhances scalability.
Stumpy outperforms previous methods in speed and scalability, allowing data scientists and analysts to extract valuable insights from time series data more effectively, supporting applications from anomaly detection to pattern discovery and classification.
If you want to evolve your company with AI, stay competitive, and use Stumpy for modern time series analysis. Discover how AI can redefine your way of work by identifying automation opportunities, defining KPIs, selecting an AI solution, and implementing gradually. For AI KPI management advice, connect with us at hello@itinai.com. For continuous insights into leveraging AI, stay tuned on our Telegram or Twitter.
Discover how AI can redefine your sales processes and customer engagement. Explore solutions at itinai.com.