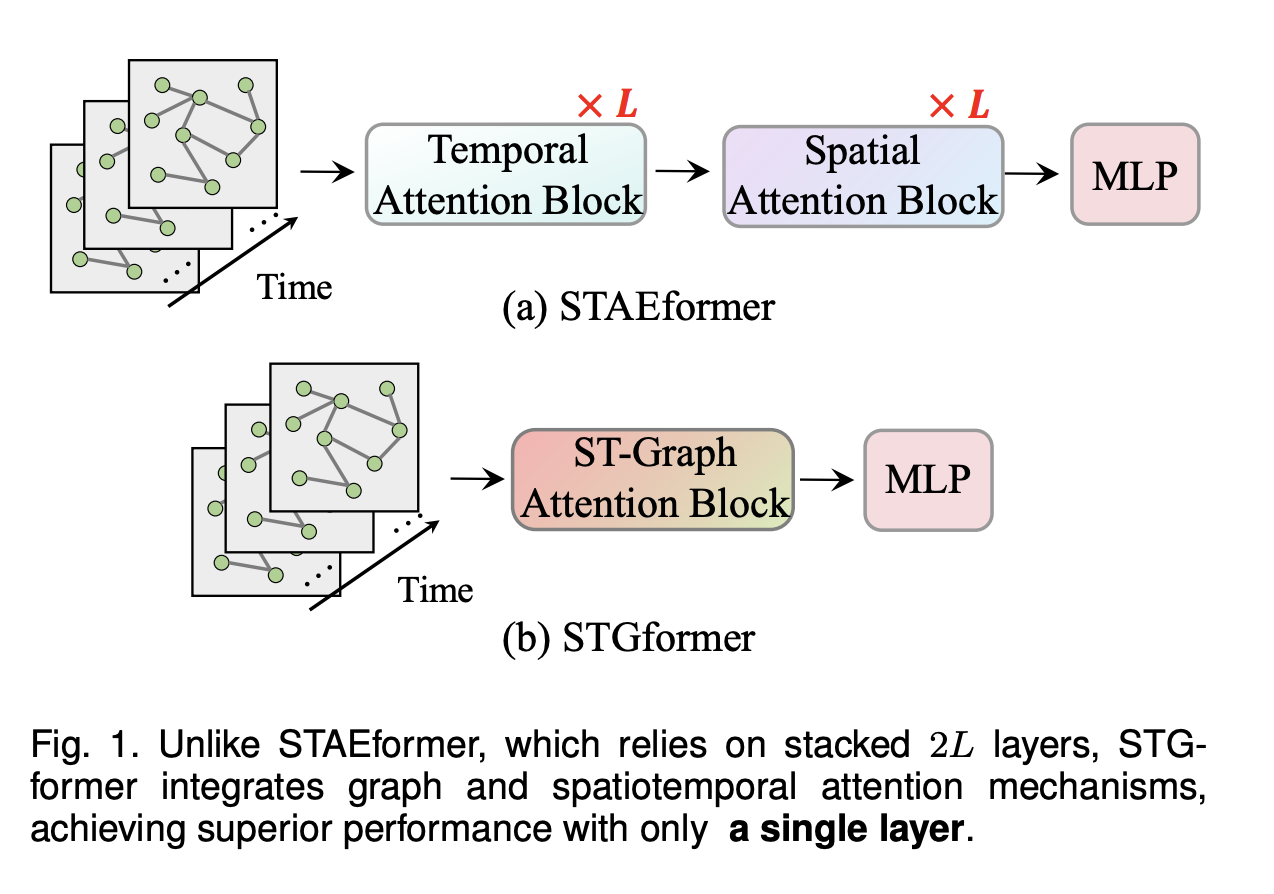
Practical Solutions for Efficient Traffic Forecasting
Challenges in Traffic Forecasting:
Traffic forecasting plays a crucial role in smart city management, but traditional models struggle with the complexity of large-scale road networks like California’s. New deep learning techniques offer potential solutions.
Introducing STGformer Model:
The STGformer model combines graph-based convolutions with Transformer-like attention blocks to efficiently forecast traffic. It offers a 100x speedup and 99.8% reduction in GPU memory usage compared to existing models.
Key Features and Benefits:
- Computational Efficiency: STGformer is 100 times faster and consumes 99.8% less GPU memory than traditional models like STAEformer.
- Scalability: Handles networks with up to 20,000 sensors, overcoming limitations of current models.
- Performance Gains: Shows significant improvements in forecasting accuracy across various datasets.
- Generalization Capability: Maintains high accuracy in diverse traffic scenarios and cross-year tests.
Advantages of STGformer:
- Efficiently captures local and global traffic patterns, enabling deployment on large-scale networks.
- Provides a scalable and accurate solution for traffic forecasting in smart city management.
AI Integration for Business Evolution:
Embrace AI solutions like STGformer to redefine your workflows. Implement AI gradually, starting with key customer touchpoints, and measure the impact on business outcomes.
Want to explore AI opportunities for your company? Contact us at hello@itinai.com for AI KPI management advice. For more insights, follow us on Telegram and Twitter.
Discover how AI can enhance your sales processes and customer engagement at itinai.com.