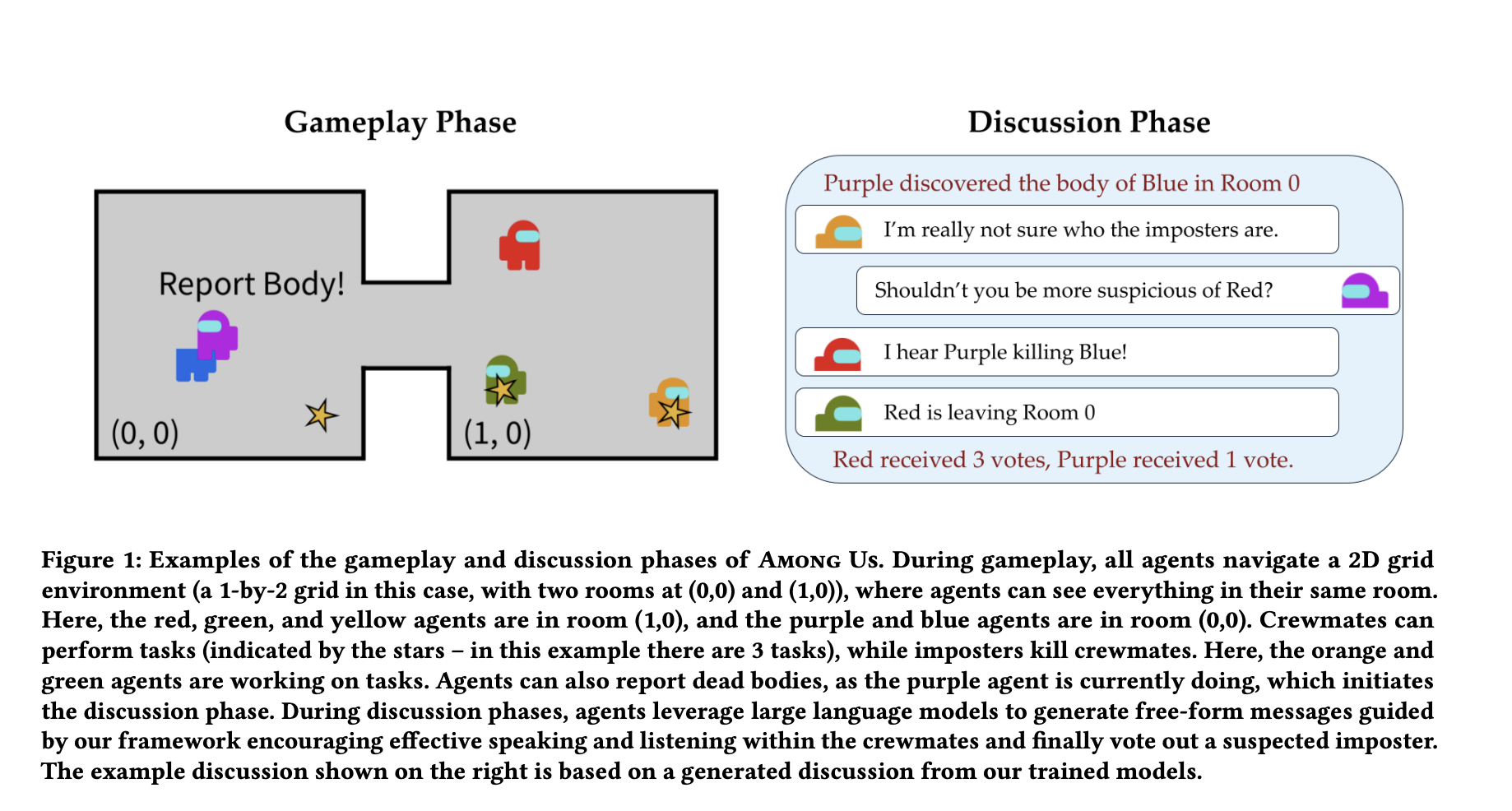
Advancements in AI Communication for Multi-Agent Environments
Understanding the Challenge
Artificial intelligence (AI) has made great progress in multi-agent environments, especially in reinforcement learning. A major challenge is enabling AI agents to communicate effectively using natural language. This is crucial when agents have limited visibility of their surroundings, making it essential to share knowledge to achieve common goals. Social deduction games are perfect for testing AI’s ability to gather information through conversation, as they require reasoning, detecting deception, and collaborating strategically.
Addressing Communication Issues
A significant problem in AI-driven social deduction is ensuring agents can engage in meaningful discussions without human examples. Many language models struggle in multi-agent settings because they rely on large datasets of human conversations. This leads to ineffective communication, which hampers performance in strategic games that require deduction and persuasion.
Limitations of Current Approaches
Current reinforcement learning methods often fall short. Some rely on existing datasets of human interactions, which may not be available for new scenarios. Others combine language models with reinforcement learning but struggle due to limited feedback, making it hard for AI to improve its dialogue strategies. Traditional methods do not effectively enhance communication skills over time.
Innovative Solutions from Stanford University
Researchers from Stanford University have developed a new method for training AI agents in social deduction games without human demonstrations. Their approach uses multi-agent reinforcement learning to help AI understand and express meaningful arguments. Focusing on the game *Among Us*, where players identify an imposter through discussion, they created a training system that separates listening and speaking skills, allowing AI to optimize both.
Structured Reward System
The methodology includes a dense reward signal that gives precise feedback to improve communication. AI agents enhance their listening by predicting details based on discussions and improve their speaking through reinforcement learning, where messages are evaluated based on their influence on other agents’ beliefs. This structured approach ensures AI messages are logical, persuasive, and relevant.
Successful Outcomes
Experimental results show that this training method significantly boosts AI performance compared to traditional techniques. The trained AI behaves like human players, successfully accusing suspects and presenting evidence. The study found that AI using this structured framework achieved a win rate of about 56%, compared to 28% for models without it. Additionally, the AI outperformed larger models, highlighting the efficiency of this training strategy.
Adapting to Challenges
AI models trained with this framework effectively adapted to adversarial strategies. Imposters tried to mislead discussions, but AI agents learned to distinguish between genuine accusations and false statements. Messages that named a suspect were more likely to influence group decisions, showing that AI could dynamically adjust its discussion strategies.
Implications for the Future
This research represents a significant step forward in AI-driven social deduction. By tackling communication challenges in multi-agent settings, it provides a structured framework for training AI agents to engage in meaningful discussions without relying on extensive human examples. This advancement enhances AI decision-making, enabling more persuasive and logical reasoning in collaborative environments.
Explore Further
For more insights, check out the research paper. Follow us on Twitter and join our 75k+ ML SubReddit community.
Transform Your Business with AI
To stay competitive, consider how the Stanford researchers’ framework can benefit your company. Here are some practical steps:
– **Identify Automation Opportunities**: Find key customer interaction points that can benefit from AI.
– **Define KPIs**: Ensure your AI initiatives have measurable impacts on business outcomes.
– **Select an AI Solution**: Choose tools that fit your needs and allow for customization.
– **Implement Gradually**: Start with a pilot project, gather data, and expand AI usage wisely.
For AI KPI management advice, connect with us at hello@itinai.com. For ongoing insights into leveraging AI, follow us on Telegram at t.me/itinainews or Twitter @itinaicom.
Discover how AI can transform your sales processes and customer engagement at itinai.com.