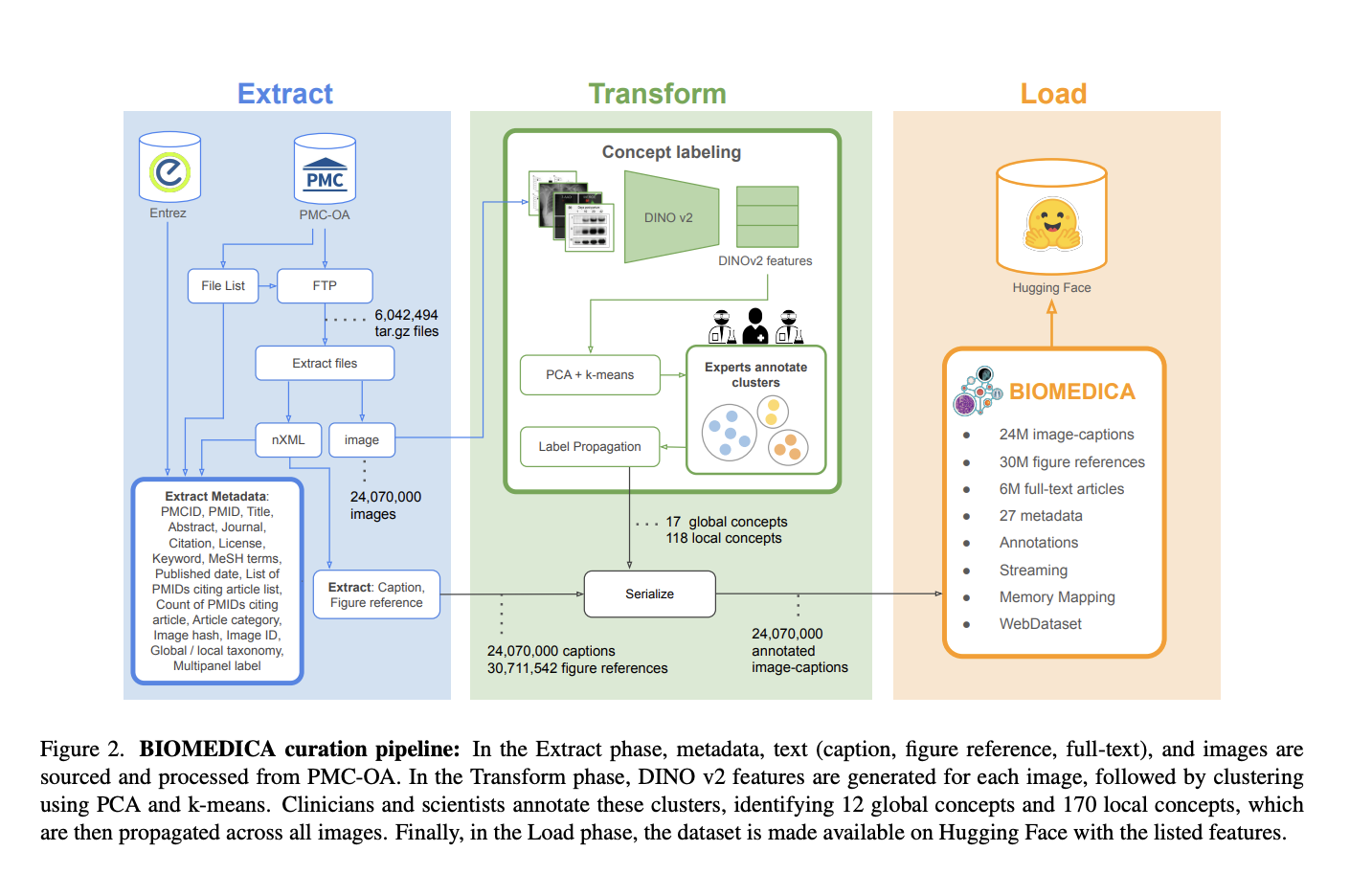
Challenges in Developing Biomedical Vision-Language Models
The creation of Vision-Language Models (VLMs) in the biomedical field is difficult due to:
- Lack of Large Datasets: There are few publicly accessible datasets that cover diverse biomedical areas. Existing datasets often focus too much on radiology and pathology while ignoring other important fields.
- Privacy and Complexity Issues: Concerns about patient privacy and the difficulty of expert-level data annotation hinder the development of comprehensive datasets.
Existing Solutions and Limitations
Previous methods, like ROCO and MEDICAT, aimed to create extensive image-caption pairs. However, these methods have limitations in capturing the full scope of biomedical knowledge needed for effective VLMs.
Advancements with BIOMEDICA Framework
Introduction to BIOMEDICA
Stanford University researchers developed BIOMEDICA, an open-source framework that organizes data from PubMed Central. It offers:
- 24 Million Image-Text Pairs: From over 6 million articles, this dataset includes valuable metadata and expert annotations.
- State-of-the-Art Performance: Models trained on BIOMEDICA excel in various medical tasks—improving classification accuracy by an average of 6.56% and reducing computational needs.
Data Curation Process
BIOMEDICA’s data curation involves:
- Extraction: Collecting articles and images from the NCBI server.
- Labeling: Using expert-driven hierarchical taxonomy to label images effectively.
- Efficient Streaming: The dataset is organized for easy access and use in machine learning applications.
Evaluation and Results
BIOMEDICA was evaluated using 39 established biomedical classification tasks, demonstrating superior performance over previous methods. Key points include:
- Robust Metrics: The evaluation included various metrics such as accuracy and retrieval recall.
- Improved Efficiency: Models trained on this dataset achieved better results while using significantly less data and computation.
Conclusion: A Valuable Resource for Biomedical AI
BIOMEDICA transforms the PubMed Central dataset into a rich resource for AI research, featuring:
- Large-Scale Data: 24 million image-caption pairs with extensive metadata.
- Open-Source Accessibility: All resources, including datasets and models, are available for public use.
- Enhanced Performance: Achieves state-of-the-art results across multiple biomedical tasks with fewer resources.
For further information, explore the Paper and Project Page. Follow us on Twitter, join our Telegram Channel, and connect on LinkedIn.
Explore How AI Can Transform Your Business
To stay competitive, leverage AI solutions such as BIOMEDICA. Consider these steps:
- Identify Automation Opportunities: Find areas in customer interactions that could benefit from AI.
- Define KPIs: Measure the impact of your AI initiatives on business results.
- Select Suitable AI Tools: Choose and customize tools that fit your needs.
- Gradual Implementation: Start small with pilot programs and scale up based on results.
For AI KPI management advice, contact us at hello@itinai.com. Stay informed about AI developments through our Telegram or follow us on @itinaicom.
Discover how AI can enhance your sales processes and customer engagement at itinai.com.