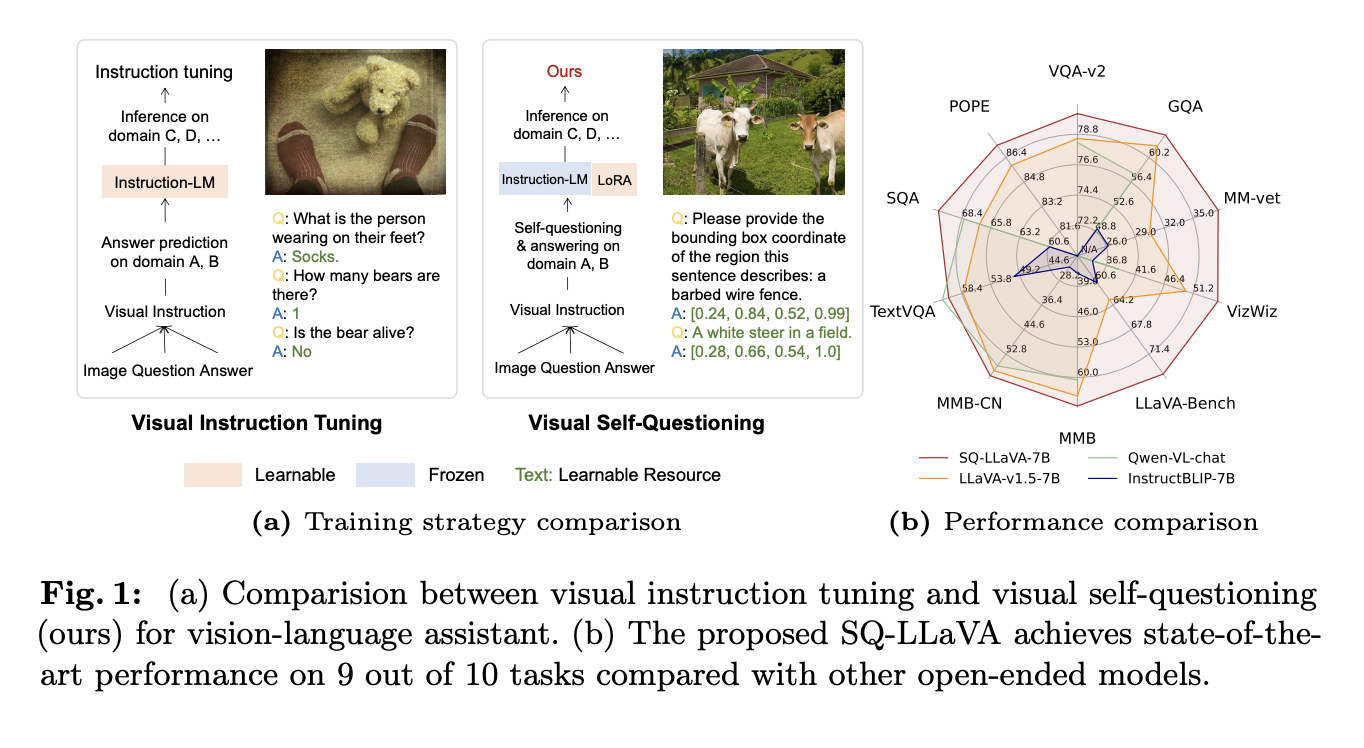
Powerful Vision-Language Models
Vision-language models like LLaVA are valuable tools that excel in understanding and generating content that includes both images and text. They improve tasks such as object detection, visual reasoning, and image captioning by utilizing large language models (LLMs) trained on visual data. However, creating high-quality visual instruction datasets is challenging, as these require a wide range of images and texts.
Significant Challenges and Solutions
The effectiveness of these models depends on the quality and variety of datasets, influencing performance on benchmarks like GQA and VizWiz. To overcome data limitations, researchers have advanced methods like instruction tuning, which helps models understand and act on human instructions effectively.
Innovative Approach: SQ-LLaVA
A novel framework called SQ-LLaVA utilizes a self-questioning method to enhance the understanding of vision and language. This model empowers the LLM to ask questions and discover visual clues independently, improving its ability to interpret images.
Key Features of SQ-LLaVA
- Optimized Alignment: Employs Low-Rank Adaptations (LoRAs) for efficient alignment between vision and language.
- Prototype Extractor: Enhances visual representation by learning meaningful semantic clusters.
- Visual Self-Questioning: Uses a special token to generate context-rich questions about images.
Model Architecture
The SQ-LLaVA model consists of four main components:
- CLIP-ViT Vision Encoder: Extracts embeddings from images.
- Prototype Extractor: Enriches image tokens with learned visual clusters.
- Trainable Projection Block: Facilitates mapping between visual and language domains.
- Vicuna LLM Backbone: Predicts subsequent tokens based on image embeddings.
Impressive Performance Metrics
SQ-LLaVA has shown remarkable improvements in various tasks:
- Overall Performance: Outperformed prior methods in six out of ten tasks.
- Scientific Reasoning: Excelled in complex multi-hop reasoning tasks.
- Reliability: Achieved better consistency with lower object hallucination rates.
- Scalability: Demonstrated effectiveness with larger models.
- Visual Information Discovery: Generated meaningful, diverse questions about images.
- Zero-shot Image Captioning: Showed significant improvements in captioning tasks.
Why Choose SQ-LLaVA?
SQ-LLaVA enhances vision-language understanding efficiently, requiring fewer parameters and less data. Its innovative questioning strategy fosters curiosity and proactive problem-solving in AI models, paving the way for more efficient vision-language applications.
Explore Further
To delve deeper into this research, check out the Paper and GitHub. Follow us on Twitter, join our Telegram Channel, and connect with our LinkedIn Group. If you appreciate our insights, subscribe to our newsletter and join our thriving 50k+ ML SubReddit.
Maximize Your Business with AI
Embrace AI solutions like SQ-LLaVA to enhance your company’s competitive edge. Here are steps to harness AI:
- Identify Automation Opportunities: Find key areas in customer interactions that could benefit from AI.
- Define KPIs: Ensure measurable impacts from AI initiatives.
- Select an AI Solution: Choose customizable tools that meet your specific needs.
- Implement Gradually: Start small, gather data, and expand AI use wisely.
Contact Us for AI Guidance
For AI KPI management advice, connect with us at hello@itinai.com. Stay informed on AI insights through our Telegram or follow us on Twitter.
Discover how AI can transform your sales processes and customer interactions at itinai.com.