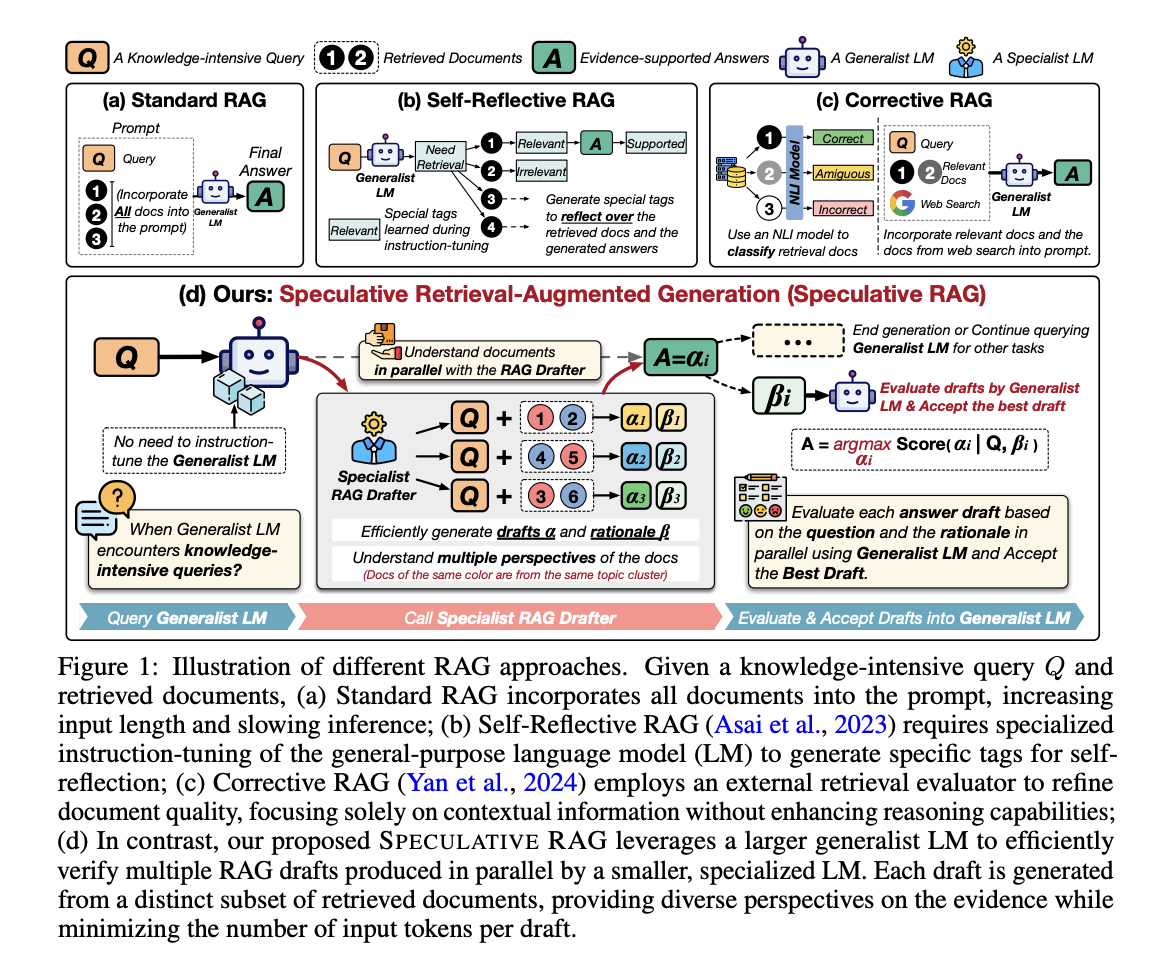
The Value of Speculative Retrieval Augmented Generation (Speculative RAG)
Enhancing Accuracy and Efficiency in Knowledge-intensive Query Processing with LLMs
The field of natural language processing has seen significant advancements with the emergence of Large Language Models (LLMs). These models excel in tasks like question answering but face challenges with knowledge-intensive queries, leading to factual inaccuracies and content generation issues.
Efficiently integrating external knowledge into LLMs is a critical area of research. The Speculative Retrieval Augmented Generation (Speculative RAG) framework addresses this by combining specialist and generalist language models to improve response generation efficiency and accuracy.
Speculative RAG strategically generates multiple drafts of potential answers in parallel and leverages diverse perspectives to ensure accurate and efficient responses. Rigorous testing has shown substantial improvements in accuracy and latency across various benchmarks, highlighting the framework’s potential to set new standards in applying LLMs for complex queries.
For companies looking to evolve with AI, Speculative RAG offers the opportunity to redefine work processes, enhance customer engagement, and identify automation opportunities. It is crucial to select AI solutions that align with business needs and provide measurable impacts on outcomes, implementing them gradually to gather data and expand usage judiciously.
To explore AI solutions and receive AI KPI management advice, connect with us at hello@itinai.com. Stay tuned for continuous insights into leveraging AI on our Telegram channel or Twitter.