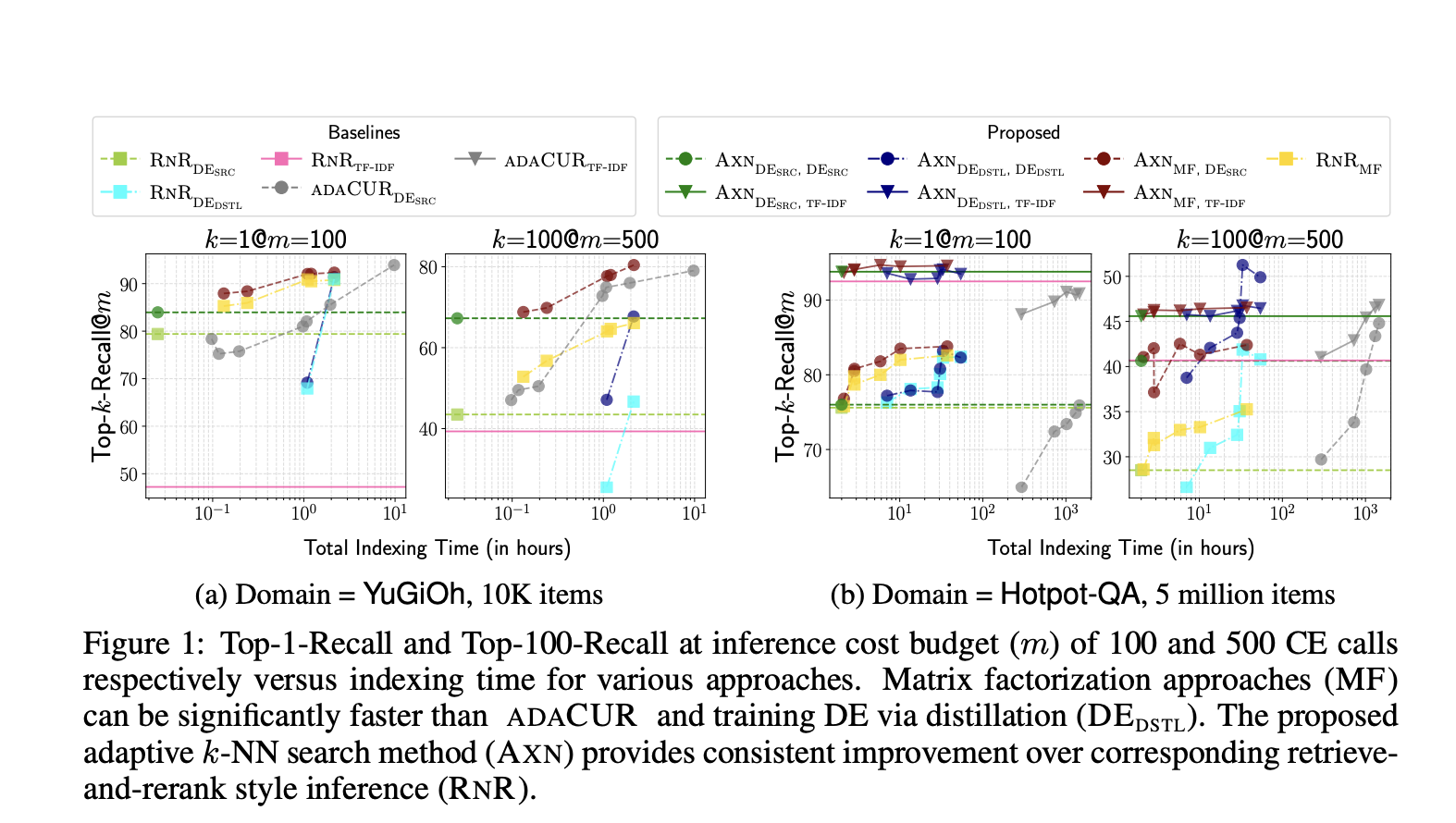
Cross-Encoder Models for Efficient Query-Item Similarity Evaluation
Cross-encoder (CE) models are used to evaluate similarity between a query and an item by encoding them simultaneously. These models outperform traditional methods, such as dot-product with embedding-based models, in estimating query-item relevance.
Practical Solutions and Value
The introduced sparse-matrix factorization-based method efficiently computes latent query and item representations to approximate CE scores. This enables optimal k-NN search using the approximate CE similarity, generating high-quality approximations with fewer CE similarity calls. The method aligns item embeddings with the cross-encoder, leading to significant improvements in k-NN recall and speedup over baseline methods.
Matrix Factorization for Sparse Matrices
Matrix factorization is widely used for evaluating low-rank approximation of matrices and recovering missing entries. Researchers have explored methods for factorizing sparse matrices and optimizing the recovery of missing entries when features describing the matrix’s rows and columns are available.
Practical Solutions and Value
The method introduced by the University of Massachusetts Amherst and Google DeepMind optimally computes latent query and item representations, efficiently approximates CE scores, and enhances k-NN search performance. The experimentation on ZESHEL and BEIR datasets demonstrates its effectiveness in tasks like zero-shot entity linking and information retrieval.
AI Implementation Recommendations
Discover how AI can redefine your way of work. Identify Automation Opportunities, Define KPIs, Select an AI Solution, and Implement Gradually. For AI KPI management advice, connect with us at hello@itinai.com.
Spotlight on a Practical AI Solution
Consider the AI Sales Bot from itinai.com/aisalesbot designed to automate customer engagement 24/7 and manage interactions across all customer journey stages.