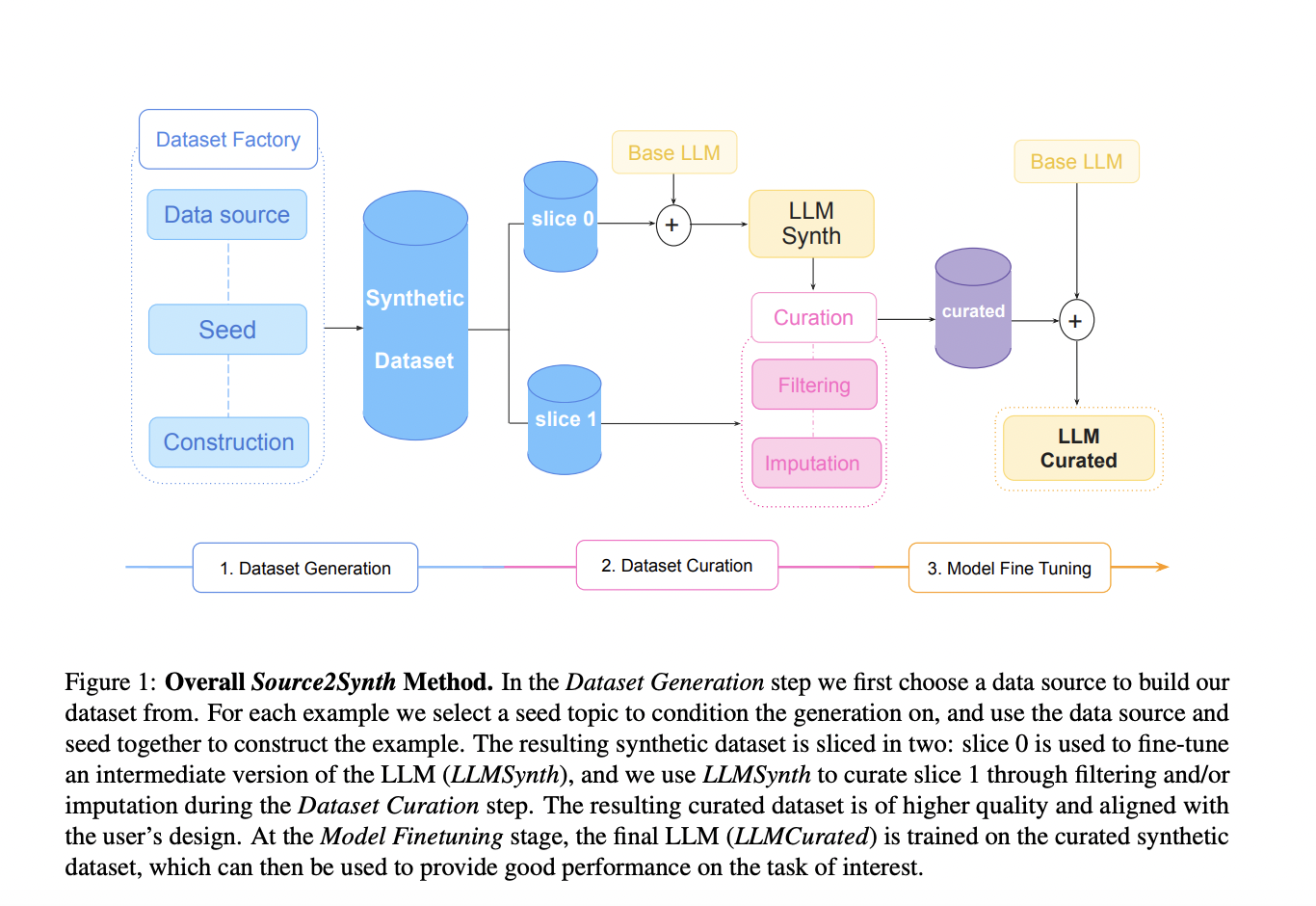
Practical Solutions and Value of Source2Synth AI Technique
Challenges Addressed:
Large Language Models (LLMs) struggle with tasks requiring structured data handling and multi-step reasoning.
Source2Synth Overview:
Source2Synth is a technique that enhances LLMs’ skills without costly human annotations by generating realistic synthetic data.
Key Features:
- Creates diverse and factually correct synthetic data based on real sources.
- Generates intricate examples with reasoning steps to improve LLM performance.
- Filters data to ensure high quality and valuable examples for training.
Applications:
- Multi-Hop Question Answering (MHQA): Achieved 22.57% improvement on HotPotQA dataset.
- Tabular Question Answering (TQA): Showed 25.51% enhancement on WikiSQL dataset.
Benefits:
Enhances LLM performance on complex tasks without extensive human annotations. Offers scalable training method for advanced reasoning and tool usage.
Conclusion:
Source2Synth is a groundbreaking technique for improving LLM capabilities in structured data handling and multi-step reasoning, ensuring high-quality training examples.