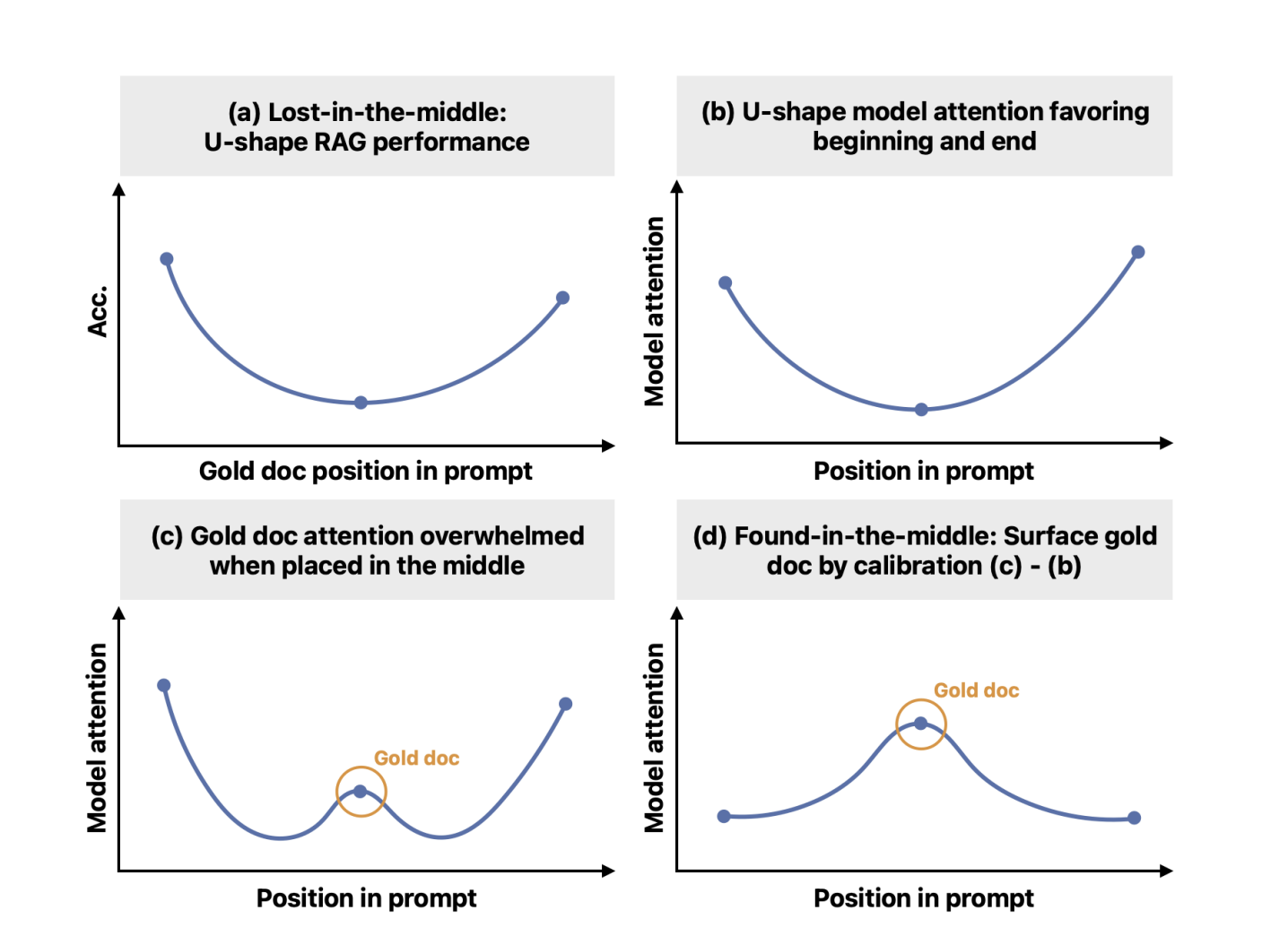
Solving the ‘Lost-in-the-Middle’ Problem in Large Language Models: A Breakthrough in Attention Calibration
Practical Solutions and Value
Despite the advancements in large language models (LLMs), they often struggle with long contexts, leading to the “lost in the middle” problem. This affects their ability to effectively utilize mid-sequence information.
Researchers have collaborated to address this issue by proposing a novel calibration mechanism called “found-in-the-middle.” This mechanism disentangles positional bias from attention scores, significantly improving the model’s ability to locate relevant information within long contexts.
The proposed solution has demonstrated improvements of up to 15 percentage points on the NaturalQuestions dataset and consistently outperforms uncalibrated models across various tasks and models. It also complements existing reordering methods, enhancing model performance.
This breakthrough in attention calibration opens new possibilities for enhancing LLM attention mechanisms and their application in various user-facing applications.
For AI KPI management advice and continuous insights into leveraging AI, connect with us at hello@itinai.com or stay tuned on our Telegram channel or Twitter.