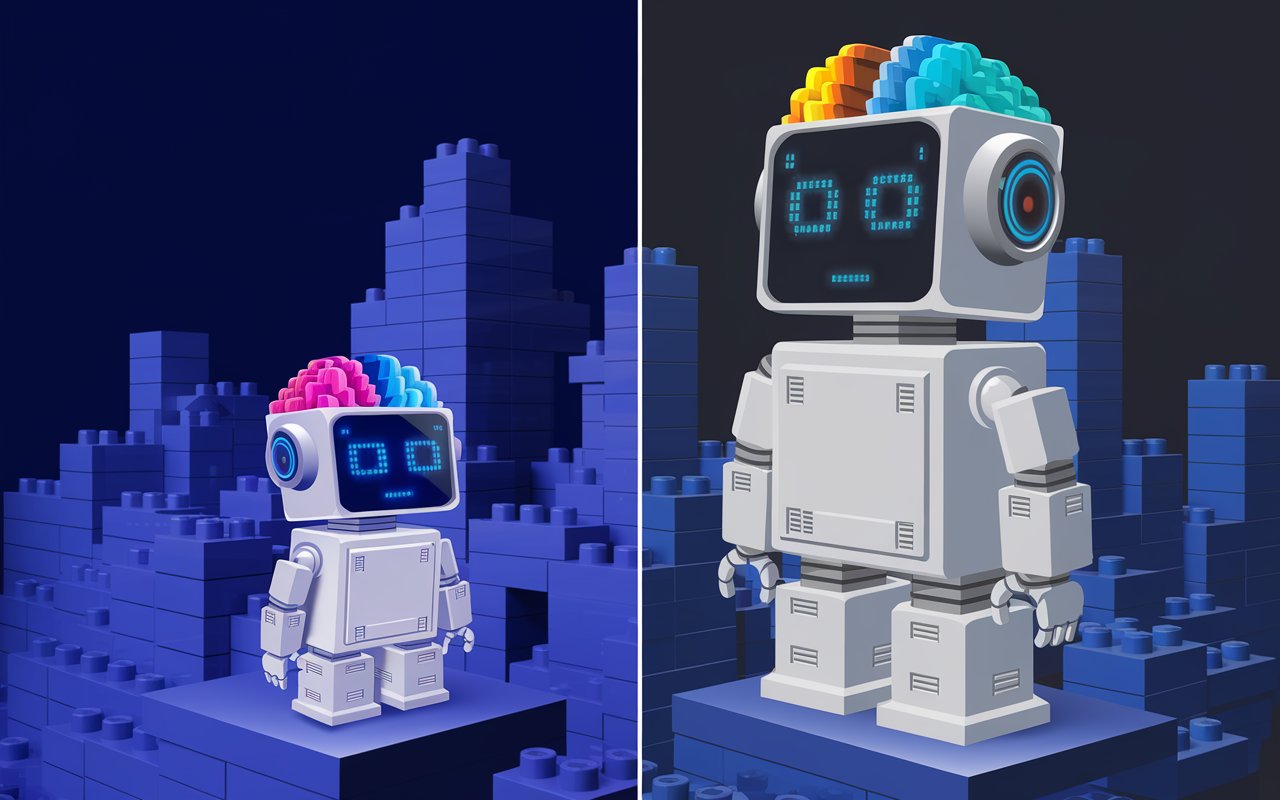
Practical Solutions and Value of Small Language Models (SLMs) in the Age of Large Language Models (LLMs)
Overview
Large Language Models (LLMs) have transformed natural language processing, but their size brings challenges. Smaller Language Models (SLMs) offer practical solutions and value in various scenarios.
Advantages of SLMs
SLMs like Phi-3.8B and Gemma-2B achieve comparable performance with fewer parameters, offering efficiency and cost-effectiveness.
They outperform LLMs in specialized tasks and are suitable for real-time applications and resource-constrained environments.
SLMs are more interpretable, crucial in fields like healthcare and finance.
Enhancing LLMs through SLMs
SLMs help curate high-quality subsets from large datasets for pre-training, improving model performance.
They assist in curating smaller, high-quality datasets for instruction tuning, optimizing the alignment with human preferences.
Collaboration and Competition
SLMs play a crucial role in enhancing LLMs through data curation and can also compete effectively in specific scenarios.
They offer advantages in accessibility, simplicity, cost-effectiveness, and interoperability.
Practical Implementation
SLMs are advantageous in computation-constrained environments, task-specific applications, and situations requiring high interpretability.
They offer promising returns in specialized domains and high-stakes decision-making contexts.
AI Solutions for Business
Identify Automation Opportunities, Define KPIs, Select an AI Solution, and Implement Gradually to evolve your company with AI.
For AI KPI management advice and continuous insights into leveraging AI, connect with us at hello@itinai.com or stay tuned on our Telegram t.me/itinainews or Twitter @itinaicom.