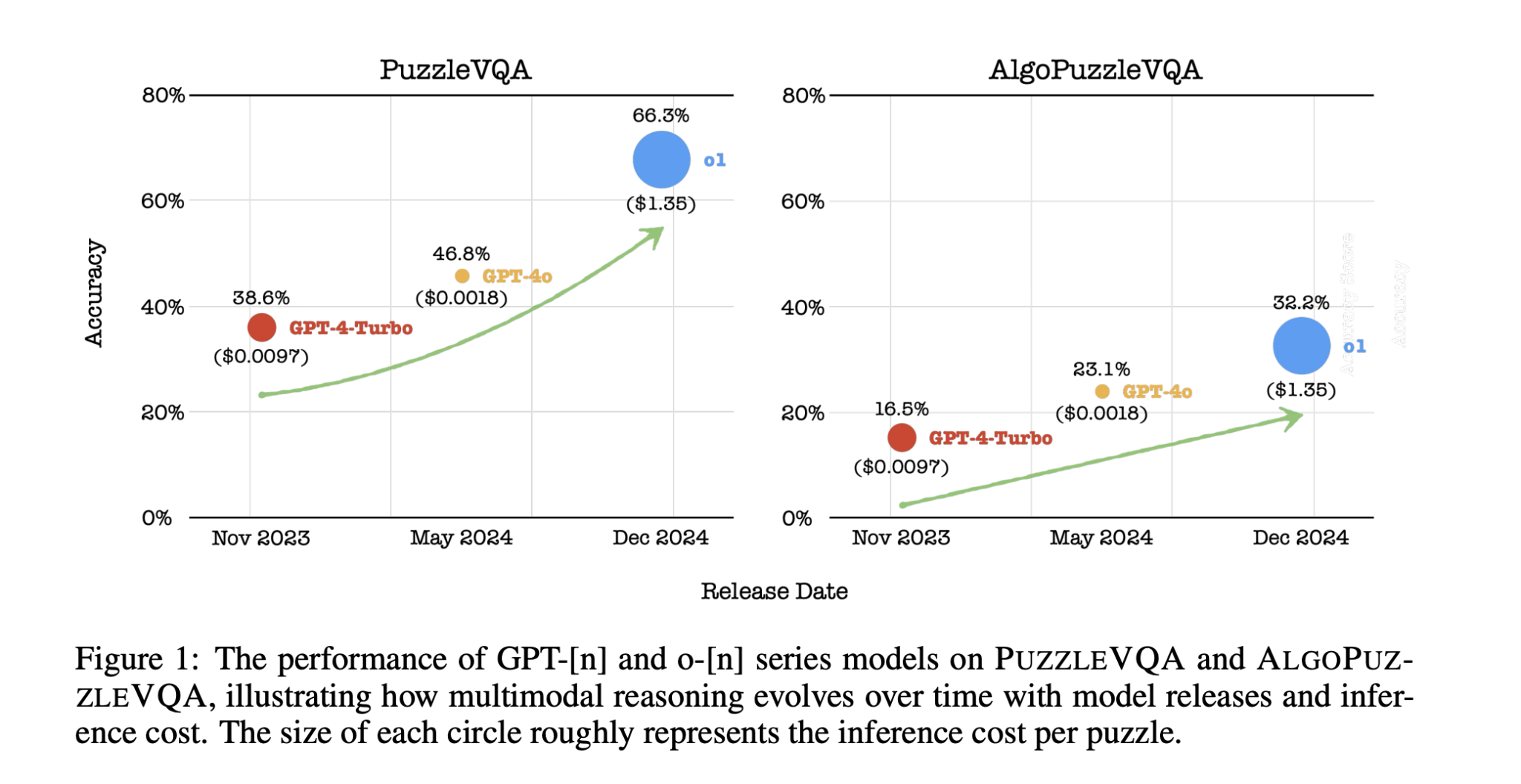
Advancements in AI Multimodal Reasoning
Overview of Current Research
After the success of large language models (LLMs), research is now focusing on multimodal reasoning, which combines vision and language. This is crucial for achieving artificial general intelligence (AGI). New cognitive benchmarks like PuzzleVQA and AlgoPuzzleVQA are designed to test AI’s ability to understand complex visual information and solve algorithmic problems.
Challenges in Multimodal Reasoning
Despite advancements, LLMs still face difficulties in multimodal reasoning, especially in recognizing patterns and solving spatial problems. High computational costs add to these challenges. Previous evaluations using symbolic benchmarks did not adequately test AI’s ability to handle multimodal inputs.
New Evaluation Datasets
Recent datasets like PuzzleVQA and AlgoPuzzleVQA assess AI’s skills in abstract visual reasoning and algorithmic problem-solving. These require models to integrate visual perception, logical deduction, and structured reasoning.
Research Findings
Researchers from the Singapore University of Technology and Design (SUTD) evaluated OpenAI’s GPT models on multimodal puzzle-solving tasks. They aimed to identify gaps in AI’s perception and reasoning skills by comparing models like GPT-4-Turbo, GPT-4o, and o1 on the new datasets.
Key Datasets Used
– **PuzzleVQA**: Focuses on recognizing patterns in numbers, shapes, colors, and sizes.
– **AlgoPuzzleVQA**: Involves logical deduction and computational reasoning tasks.
Evaluation Methodology
The evaluation included multiple-choice and open-ended questions. A zero-shot Chain of Thought (CoT) prompting method was used for reasoning. The study analyzed performance drops when switching from multiple-choice to open-ended tasks.
Results and Observations
– **Improvement in Reasoning**: There was a noticeable improvement in reasoning capabilities from GPT-4-Turbo to GPT-4o and o1, with o1 showing the most significant advancements, especially in algorithmic reasoning.
– **Performance Metrics**:
– In PuzzleVQA, o1 achieved 79.2% accuracy in multiple-choice tasks, outperforming GPT-4o and GPT-4-Turbo.
– In open-ended tasks, all models showed performance drops, with o1 at 66.3%.
– In AlgoPuzzleVQA, o1 scored 55.3% in multiple-choice tasks, significantly better than previous models.
Identified Limitations
Perception was a major challenge across all models. Providing explicit visual details improved accuracy significantly. Inductive reasoning guidance also enhanced performance, particularly in numerical and spatial tasks. While o1 excelled in numerical reasoning, it struggled with shape-based puzzles.
Conclusion
The study highlights the progress and ongoing challenges in AI multimodal reasoning. For businesses looking to leverage AI, consider the following practical steps:
– **Identify Automation Opportunities**: Find customer interaction points that can benefit from AI.
– **Define KPIs**: Ensure measurable impacts on business outcomes.
– **Select an AI Solution**: Choose tools that fit your needs and allow customization.
– **Implement Gradually**: Start with a pilot project, gather data, and expand AI usage wisely.
Stay Connected
For more insights and AI management advice, contact us at hello@itinai.com. Follow us on @itinaicom and join our Telegram Channel for continuous updates.
Explore AI Solutions
Discover how AI can transform your business processes and customer engagement at itinai.com.