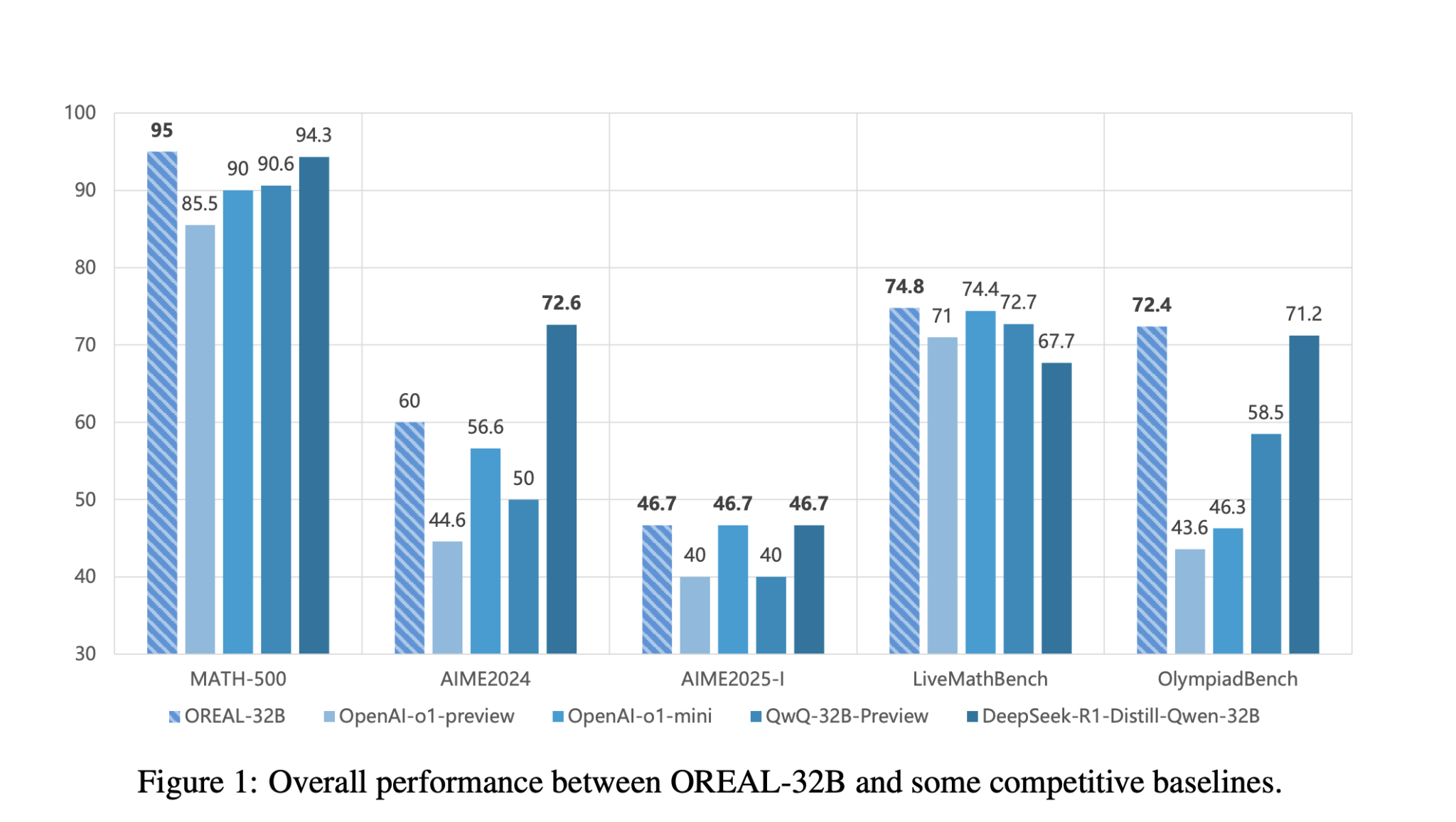
Mathematical Reasoning in AI: New Solutions from Shanghai AI Laboratory
Understanding the Challenges
Mathematical reasoning is a complex area for artificial intelligence (AI). While large language models (LLMs) have improved, they often struggle with tasks that require multi-step logic. Traditional reinforcement learning (RL) faces issues when feedback is limited to simple right or wrong answers.
Introducing OREAL Models
Shanghai AI Laboratory has created the Outcome REwArd-based reinforcement Learning (OREAL) framework, featuring two models: OREAL-7B and OREAL-32B. These models are designed to perform well even when feedback is binary. Unlike traditional RL methods, OREAL uses Best-of-N (BoN) sampling to enhance learning and adjusts negative rewards to ensure consistent performance.
Performance Highlights
– **OREAL-7B:** Achieves a 94.0% pass rate on the MATH-500 benchmark, comparable to larger models.
– **OREAL-32B:** Reaches a 95.0% pass rate, outperforming previous models.
Technical Innovations and Advantages
The OREAL framework introduces several effective techniques for mathematical reasoning:
– **Best-of-N Sampling:** This method selects the best reasoning paths for the model to learn from, improving understanding.
– **Reward Reshaping:** Adjusting negative rewards helps maintain consistency during training, leading to better optimization.
– **Token-Level Reward System:** This focuses on important reasoning steps, aiding the model in handling complex sequences.
– **On-Policy Learning:** The model improves dynamically based on responses, enhancing training efficiency.
These innovations allow for better training and performance when tackling lengthy reasoning tasks.
Benchmark Performance
OREAL models have demonstrated strong performance across various benchmarks:
– **MATH-500:** Both OREAL-7B and OREAL-32B set new standards in results, matching or exceeding larger models.
– **AIME2024 and OlympiadBench:** They show exceptional generalization across different problem types.
– **Comparison with Competitors:** OREAL-32B outshines other models, indicating effective training strategies.
Conclusion and Future Directions
The OREAL-7B and OREAL-32B models provide innovative approaches to mathematical reasoning via reinforcement learning. By tackling the challenge of sparse feedback, these models perform competitively, even at smaller scales. The findings suggest new possibilities for enhancing AI’s problem-solving capabilities.
Get Involved
Explore the foundations of OREAL in their published paper. Follow our research on Twitter, join our Telegram Channel, and connect with our LinkedIn Group. Join our thriving community on ML SubReddit with 75k+ members.
Embrace AI for Business Success
To remain competitive with AI, consider the following steps:
– **Identify Automation Opportunities:** Find customer interactions that can be improved with AI.
– **Define KPIs:** Ensure your AI projects can be measured for impact.
– **Select Suitable AI Solutions:** Choose tools that fit your needs.
– **Implement Gradually:** Start small, collect data, and expand wisely.
For advice on AI KPI management, contact us at hello@itinai.com. Stay updated on AI insights through our Telegram channel at t.me/itinainews or on Twitter @itinaicom.
Explore how AI can transform your sales and customer engagement by visiting itinai.com.