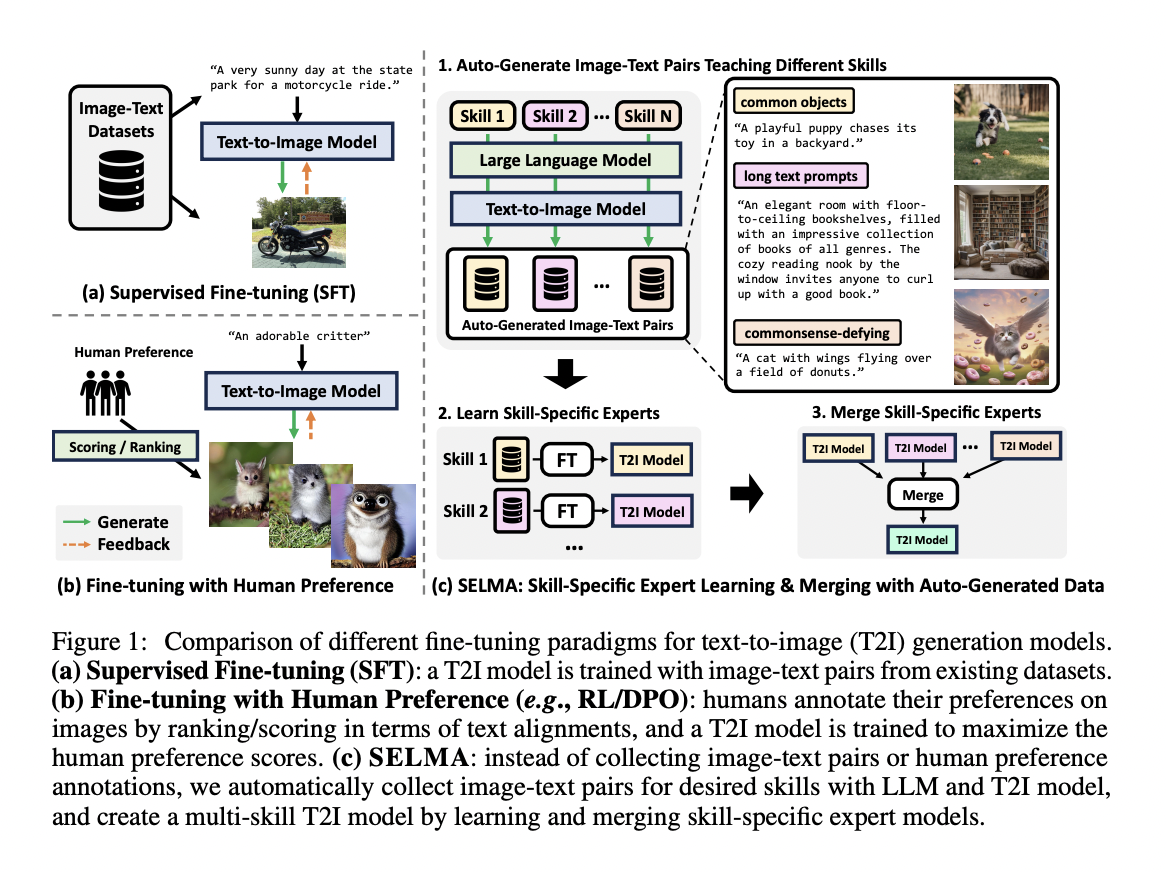
Practical Solutions for Enhancing Text-to-Image Models
Challenges in Text-to-Image Models
Text-to-image models struggle to accurately reflect all details from textual prompts, leading to unrealistic images.
Current Solutions
Researchers are working on methods to improve image faithfulness without relying on extensive human-annotated data.
SELMA: A Breakthrough Approach
SELMA introduces a new method that enhances T2I models using auto-generated skill-specific text prompts, resulting in high-quality images.
SELMA’s Four-Stage Pipeline
1. Generate diverse skill-specific prompts using Large Language Models (LLMs).
2. Create images based on these prompts using T2I models.
3. Fine-tune the model with Low-Rank Adaptation (LoRA) for each skill.
4. Merge skill-specific experts to create a robust T2I model capable of handling diverse prompts.
Key Takeaways from SELMA Research
– Improved T2I model performance on benchmarks.
– Cost-effective data generation with auto-generated datasets.
– Enhanced human preference metrics.
– Potential for weak-to-strong generalization in T2I models.
– Reduced dependency on human annotation.
Conclusion
SELMA offers a cost-effective and efficient way to enhance T2I models, addressing key limitations and paving the way for future advancements.
Evolve Your Company with AI
Stay competitive and redefine your work processes with SELMA’s innovative approach to text-to-image generation models.
AI Implementation Tips
– Identify automation opportunities.
– Define measurable KPIs.
– Select AI solutions aligned with your needs.
– Implement gradually and expand usage judiciously.
Connect with Us
For AI KPI management advice, contact us at hello@itinai.com. Follow us on Telegram and Twitter for insights into leveraging AI.