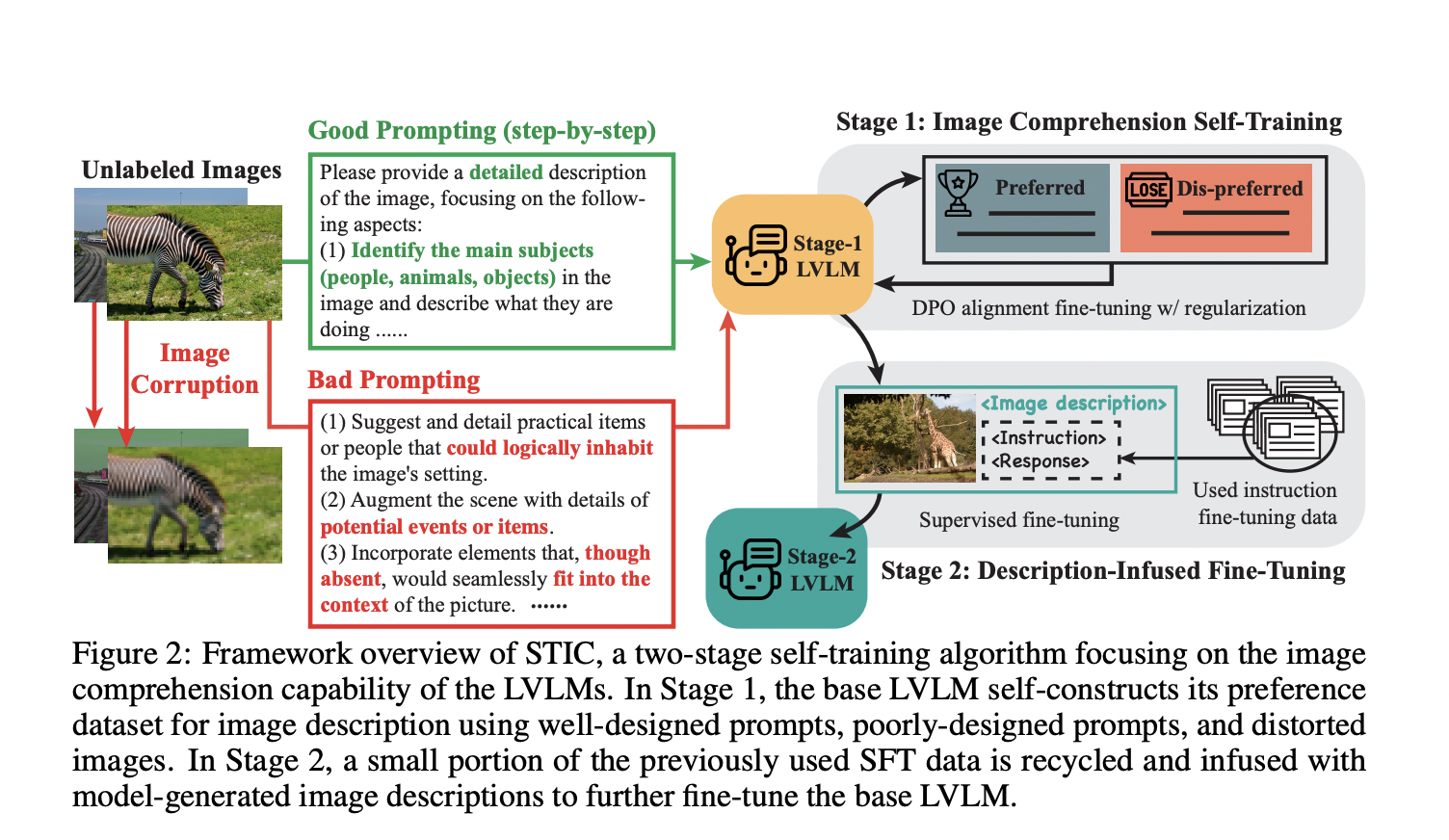
Practical Solutions and Value of Self-Training on Image Comprehension (STIC) for Large Vision Language Models (LVLMs)
Overview
Large Vision Language Models (LVLMs) combine language models with image encoders to process multimodal input. Enhancing LVLMs requires cost-effective methods for acquiring fine-tuning data.
Key Developments
Recent advancements integrate open-source language models with image encoders to create LVLMs like LLaVA, LLaMA-Adapter-V2, Qwen-VL, and InternVL. However, obtaining fine-tuning data remains a challenge.
STIC Method
STIC focuses on self-training for image comprehension in LVLMs, generating preference data from unlabeled images. It enhances reasoning on visual information through self-generated descriptions.
Performance and Results
STIC improves LVLMs’ performance significantly across seven benchmarks, showcasing an average increase of 1.7% for LLaVA-v1.5 and 4.0% for LLaVA-v1.6. It demonstrates the potential for self-improvement in LVLMs.
Future Research
Future studies could explore STIC with larger models, analyze image distribution effects on self-training, and investigate different image corruptions and prompts for further enhancements in LVLM development.
AI Integration for Business
Utilize AI solutions to redefine work processes, identify automation opportunities, define measurable KPIs, select suitable tools, and implement AI gradually for business impact.
Connect with Us
For AI KPI management advice and insights on leveraging AI, reach out to us at hello@itinai.com or follow us on Telegram and Twitter for continuous updates.