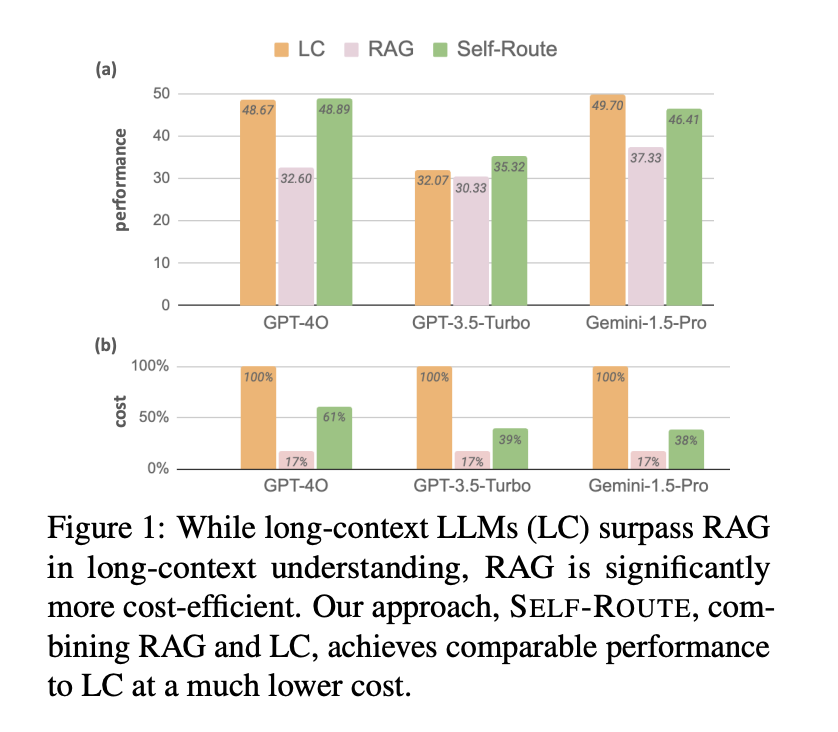
Practical Solutions for Long-Context Language Models
Revolutionizing Natural Language Processing
Large Language Models (LLMs) like GPT-4 and Gemini-1.5 have transformed natural language processing, enabling machines to understand and generate human language for tasks like summarization and question answering.
Challenges and Innovative Approaches
Managing long contexts poses computational and cost challenges. Researchers are exploring approaches like Retrieval Augmented Generation (RAG) and the SELF-ROUTE method to balance performance and efficiency.
SELF-ROUTE Method
The SELF-ROUTE method efficiently routes queries to RAG or long-context LLMs based on model self-reflection, reducing computational costs while maintaining high performance.
Evaluation and Performance Analysis
SELF-ROUTE achieved notable cost reductions while maintaining comparable performance to long-context LLMs, leveraging the strengths of both RAG and LC models.
Conclusion and Future Prospects
While long-context LLMs demonstrate superior performance, RAG remains viable due to its lower cost. The SELF-ROUTE method effectively combines the strengths of both RAG and LC, achieving performance comparable to LC at a significantly reduced cost.
AI Solutions for Business Evolution
Identify Automation Opportunities
Locate key customer interaction points that can benefit from AI.
Define KPIs
Ensure your AI endeavors have measurable impacts on business outcomes.
Select an AI Solution
Choose tools that align with your needs and provide customization.
Implement Gradually
Start with a pilot, gather data, and expand AI usage judiciously.
AI KPI Management and Insights
Connect with us at hello@itinai.com for AI KPI management advice. Stay tuned on our Telegram t.me/itinainews or Twitter @itinaicom for continuous insights into leveraging AI.